An End-to-End Multi-label classification model for Arrhythmia based on varied-length ECG signals.
TrustCom(2022)
摘要
Cardiovascular disease is the most important cause of death in the world. In the early stage of cardiovascular disease, arrhythmia is often accompanied. Therefore, it is of great significance to carry out safe and effective arrhythmia detection for prevention and diagnosis of cardiovascular diseases. With the increase in widely available digital ECG data and the algorithmic paradigm of deep learning, multi-class arrhythmia classification based on automatic feature extraction of ECG has become increasingly attractive. However, the majority of studies cannot accept varied-length ECG signals and have limited performance in detecting multi-class arrhythmias. In this study, we propose a multi-label classification network based on the multi-scale feature fusion module for arrhythmia in 12-lead varied-length ECG. Our model utilizes the complementary power between different structures, which include group convolution block, Spatial Pyramid Pooling (SPP) layer, and multi-scale feature fusion module. The proposed method can extract features from every lead separately for 12lead varied-length records, and effectively achieve multi-scale feature extraction and cross-scale information complementarity of ECG by integrating multiple convolution kernels with different receptive fields. The experimental results show that our model achieved an overall classification F1 score of 83.3%. Combining all these excellent features, this model offers a solution for varied-length signal processing problems.
更多查看译文
关键词
multi-label classification,arrhythmia detection,group convolution,Resnet,varied-length ECG,CPSC-2018
AI 理解论文
溯源树
样例
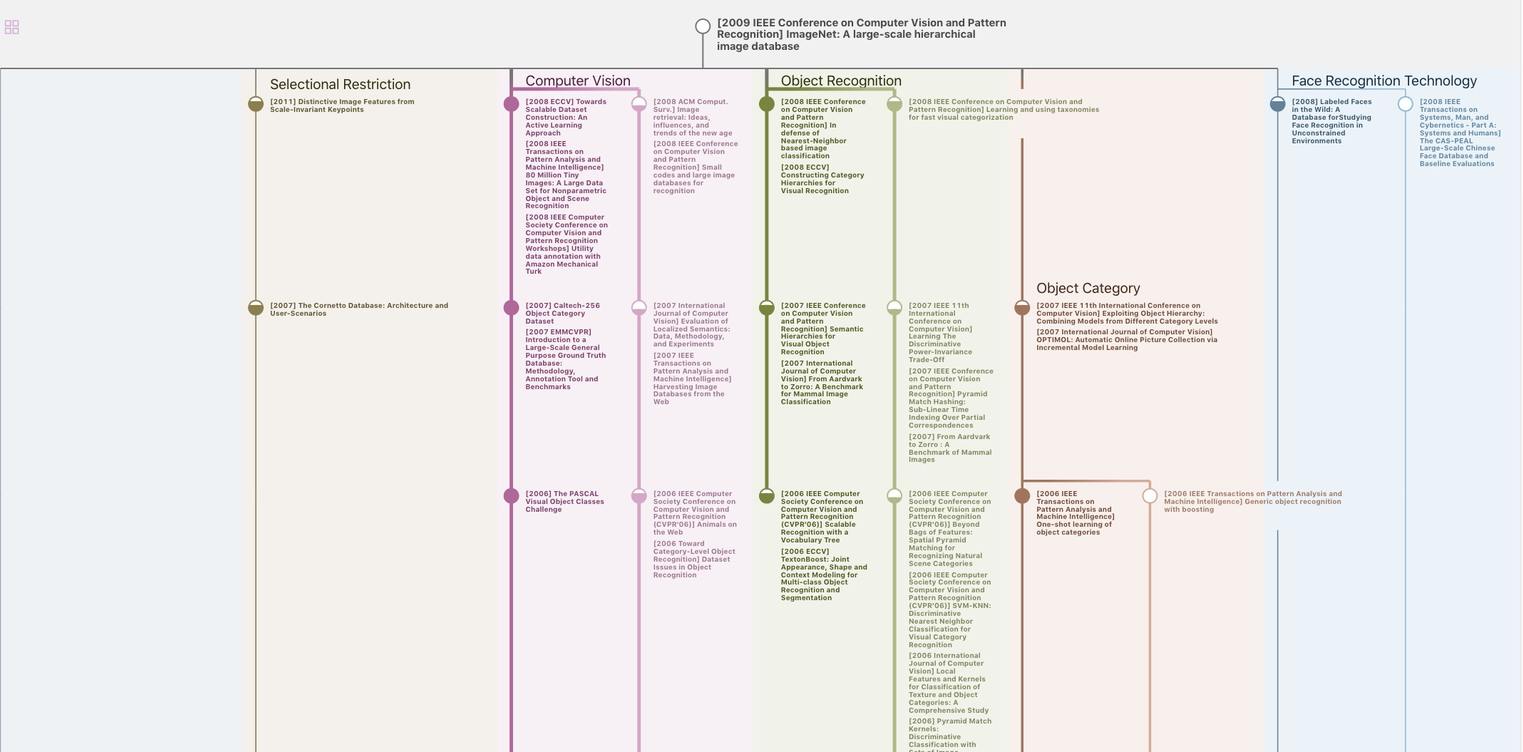
生成溯源树,研究论文发展脉络
Chat Paper
正在生成论文摘要