Optimistic Exploration Based on Categorical-DQN for Cooperative Markov Games.
DAI(2022)
摘要
In multiagent reinforcement learning (MARL), independent cooperative learners face numerous challenges when learning the optimal joint policy, such as non-stationarity, stochasticity, and relative over-generalization problems. To achieve multiagent coordination and collaboration, a number of works designed heuristic experience replay mechanisms based on the `optimistic' principle. However, it is difficult to evaluate the quality of an experience effectively, different treatments of experience may lead to overfitting and be prone to converge to sub-optimal policies. In this paper, we propose a new method named optimistic exploration categorical DQN (OE-CDQN) to apply the `optimistic' principle to the action exploration process rather than in the network training process, to bias the probability of choosing an action with the frequency of receiving the maximum reward for that action. OE-CDQN is a combination of the `optimistic' principle and CDQN, using an `optimistic' re-weight function on the distributional value output of the CDQN network. The effectiveness of OE-CDQN is experimentally demonstrated on two well-designed games, i.e., the CMOTP game and a cooperative version of the boat problem which confronts ILs with all the pathologies mentioned above. Experimental results show that OE-CDQN outperforms state-of-the-art independent cooperative methods in terms of both learned return and algorithm robustness.
更多查看译文
关键词
Cooperative Markov games, Distributional reinforcement learning, Independent learning, Optimistic principle
AI 理解论文
溯源树
样例
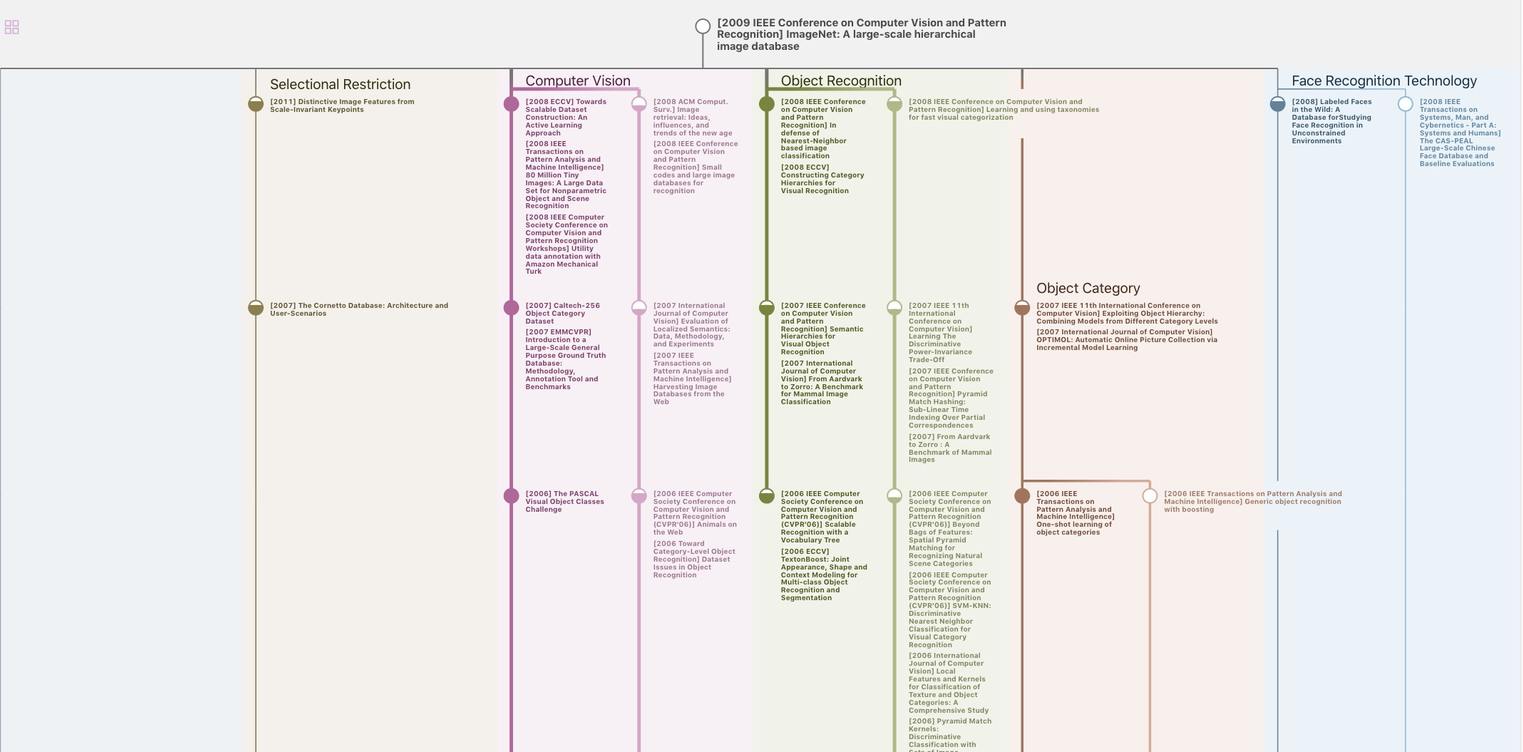
生成溯源树,研究论文发展脉络
Chat Paper
正在生成论文摘要