SciCNN: A 0-Shot-Retraining Patient-Independent Epilepsy-Tracking SoC.
ISSCC(2023)
摘要
Patient-specific seizure-detection SoCs targeting ambulatory seizure treatment [1–8] achieve outstanding accuracy and low energy consumption for monitoring over an extended period powered by a small battery, energy harvesting or body-coupled powering [9]. However, they must collect each patient's seizure episode EEG to train a classifier before the actual deployment, which requires patients to undergo costly and time-consuming hospitalizations, as there is no guarantee a single hospitalization can capture the event. In contrast, a patient-independent seizure detection can address these issues by training the classifier with pre-existing databases, then directly deploying to new patients (Fig. 32.6.1). Traditional classifiers, such as Logistic Regression (LR), Support Vector Machines (SVM) and Decision Trees (DT) [1–7] are not suitable for patient-independent detection as they have difficulty capturing all the possible seizure patterns across patients without firing too many false alarms; this is due to their computational structures and the nature of inter-patient seizure pattern variation. In contrast, Neural Networks (NN) could perform better by mining the features automatically [8]. However, the inter-patient seizure pattern variation could still be vague to the trained NN. Thus, we present a patient-independent (non-specific) seizure-detection SoC with a Seizure-Cluster-Inception Convolutional Neural Network (SciCNN) to first be trained offline with the pre-existing EEG database for auto feature extraction of the neural patterns, then to be further tuned online for fine calibration after being deployed to an unseen patient.
更多查看译文
AI 理解论文
溯源树
样例
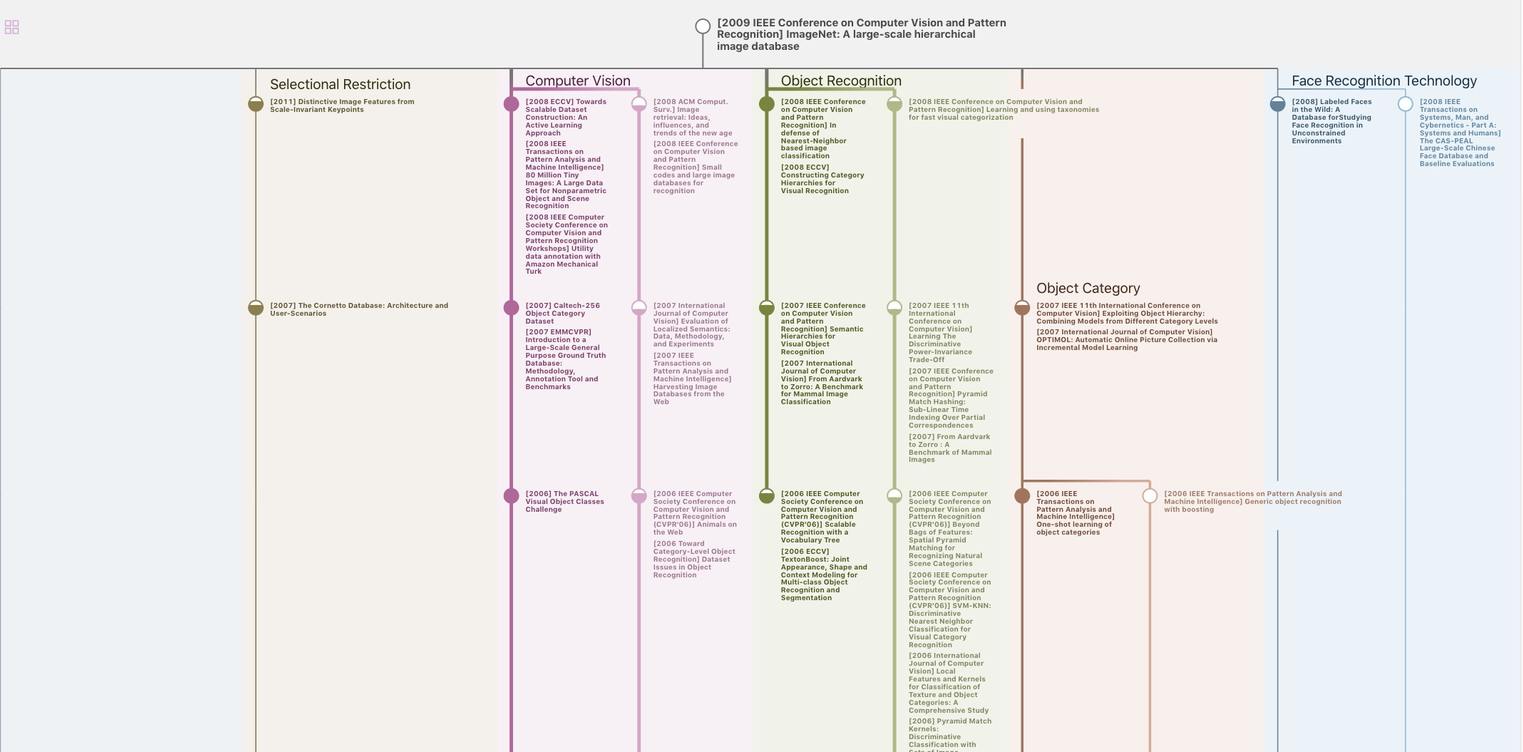
生成溯源树,研究论文发展脉络
Chat Paper
正在生成论文摘要