Federated learning by employing knowledge distillation on edge devices with limited hardware resources
Neurocomputing(2023)
摘要
This paper presents a federated learning approach based on utilizing computational resources of the IoT edge devices for training deep neural networks. In this approach, the edge devices and the cloud server collaborate in the training phase while preserving the privacy of the edge device data. Owing to the lim-ited computational power and resources available to the edge devices, instead of the original neural net-work (NN), we suggest to use a smaller NN generated using a proposed heuristic method. In the proposed approach, the smaller model, which is trained on the edge device, is generated from the main NN model. By the exploiting Knowledge Distillation (KD) approach, the learned knowledge in the server and the edge devices can be exchanged, leading to lower required computation on the server and preserving data pri-vacy of the edge devices. Also, to reduce the knowledge transfer overhead on the communication links between the server and the edge devices, a method for selecting the most valuable data to transfer the knowledge is introduced. The effectiveness of this method is assessed by comparing it to state-of-the-art methods. The results show that the proposed method lowers the communication traffic by up to 250 x and increases the learning accuracy by an average of 8.9 % in the cloud compared to the prior KD-based distributed training approaches in CIFAR-10 dataset.(c) 2023 Elsevier B.V. All rights reserved.
更多查看译文
关键词
Edge devices,Deep learning,Federated learning,Knowledge distillation,Data privacy
AI 理解论文
溯源树
样例
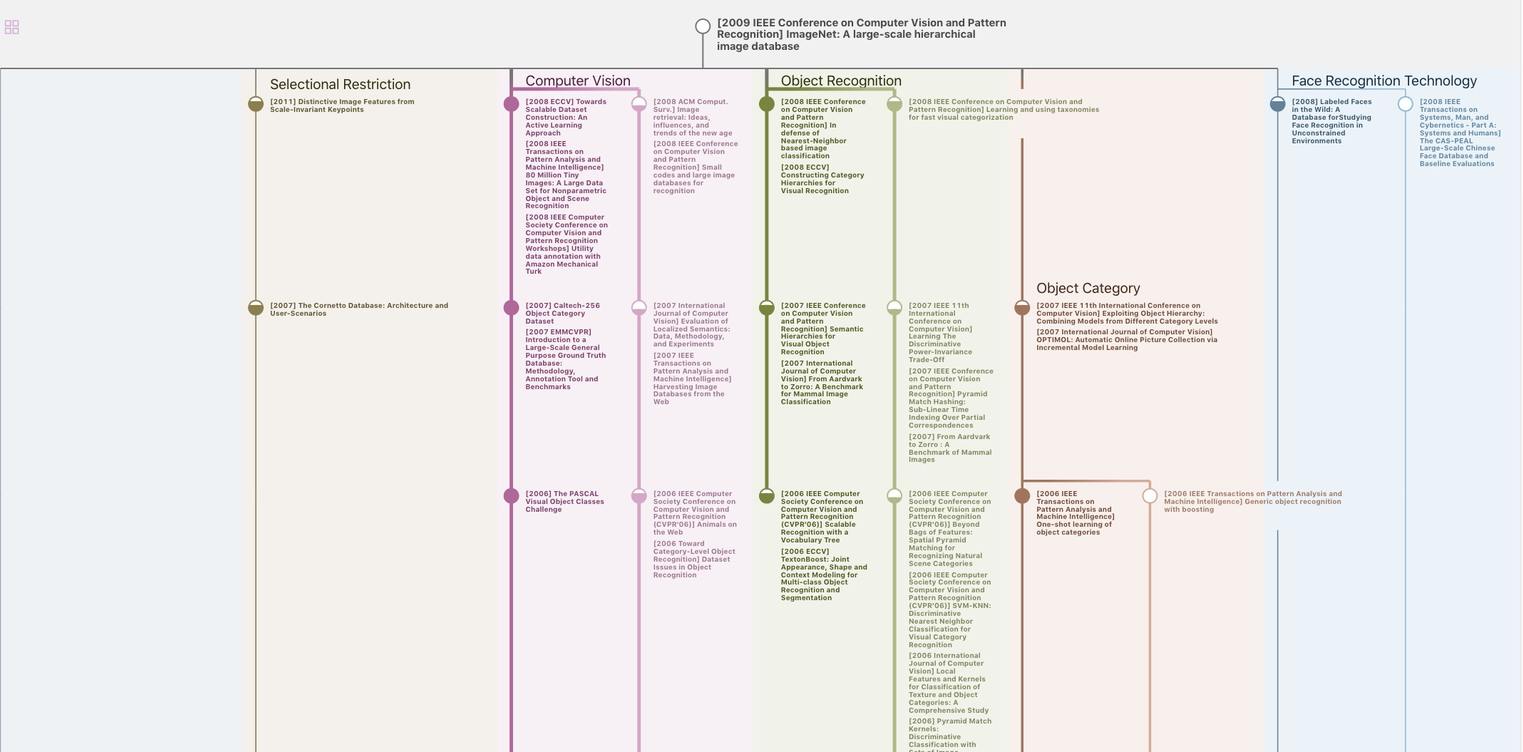
生成溯源树,研究论文发展脉络
Chat Paper
正在生成论文摘要