WBA: A Warping-based Approach to Generating Imperceptible Adversarial Examples
2022 IEEE INTERNATIONAL CONFERENCE ON TRUST, SECURITY AND PRIVACY IN COMPUTING AND COMMUNICATIONS, TRUSTCOM(2022)
摘要
The human can easily recognize the incongruous parts of an image, for example, perturbations unrelated to the image itself, but are poor at spotting the small geometric transformations. However, in terms of the robustness of deep neural networks (DNNs), the ability to properly recognize objects with small geometric transformations is still a challenge. In this work, we investigate the problem from the perspective of adversarial attacks: does the performance of DNNs degrade even when small geometric transformations are applied to images? To this end, we propose a novel adversarial attack method, called WBA, a Warping-Based Adversarial attack method, which does not introduce information independent of the original images but manipulates the existing pixels of the images by elastic warping transformations to generate adversarial examples that are imperceptible to the human eye. At the same time, existing adversarial attacks typically generate adversarial examples by modifying pixels in the spatial domain of the image, the addition of such perturbations introduces extra information unrelated to the image itself and is easily detected by the naked eyes. We demonstrate the effectiveness of WBA by extensive experiments on commonly used datasets, including MNIST, CIFAR10, and ImageNet. The results show that WBA can quickly generate adversarial examples with the highest adversarial strength, consumes less time, and can be comparable to optimization-based adversarial attack methods in image perception evaluation metrics such as LPIPS, SSIM, and far more than gradient direction-based iterative methods.
更多查看译文
关键词
Neural network,Adversarial attack,Adversarial eamples,Elastic warping transformation,Imperceptibility
AI 理解论文
溯源树
样例
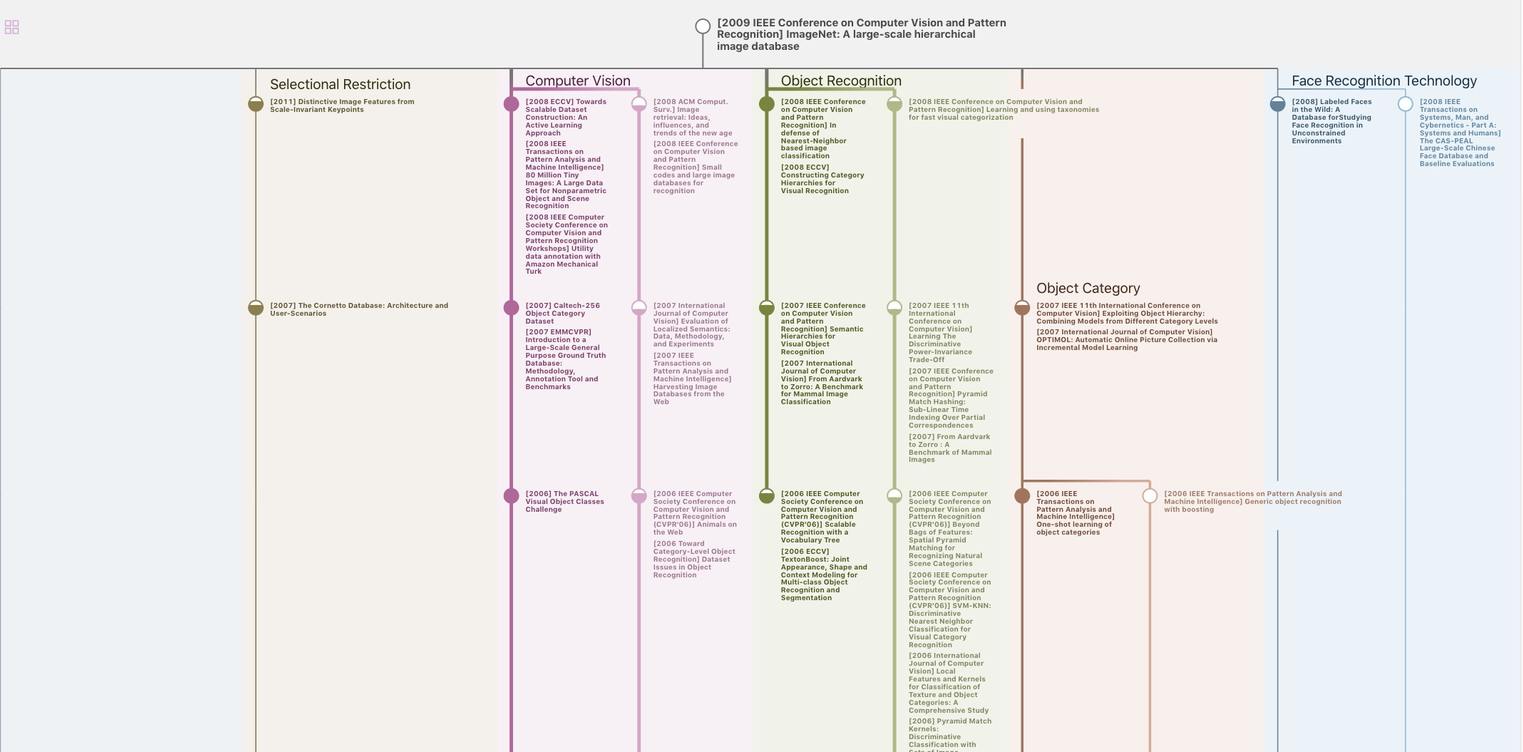
生成溯源树,研究论文发展脉络
Chat Paper
正在生成论文摘要