A Relation Surrogate Model for Expensive Multiobjective Continuous and Combinatorial Optimization.
EMO(2023)
摘要
Currently, the research on expensive optimization problems mainly focuses on continuous problems and ignores combinatorial problems, which exist in many real-world applications. Since in surrogate model assisted evolution algorithms (SAEAs), the surrogate models from the community of machine learning are usually designed from continuous problems, and they are not suitable from combinatorial problems. For this reason, we propose a convolution relation model for both continuous and combinatorial problems. In the new relation model, a sample representation method of a relation map is proposed in the data preparation, and the convolution neural network is used to learn the relationships between pairs of candidate solutions. The new method is embedded into a basic multiobjective evolutionary algorithm and applied to a set of continuous and combinatorial problems. The experimental results suggest that the relation model with the same settings can solve continuous and combinatorial problems, and it has an advantage in terms of problem scalability.
更多查看译文
AI 理解论文
溯源树
样例
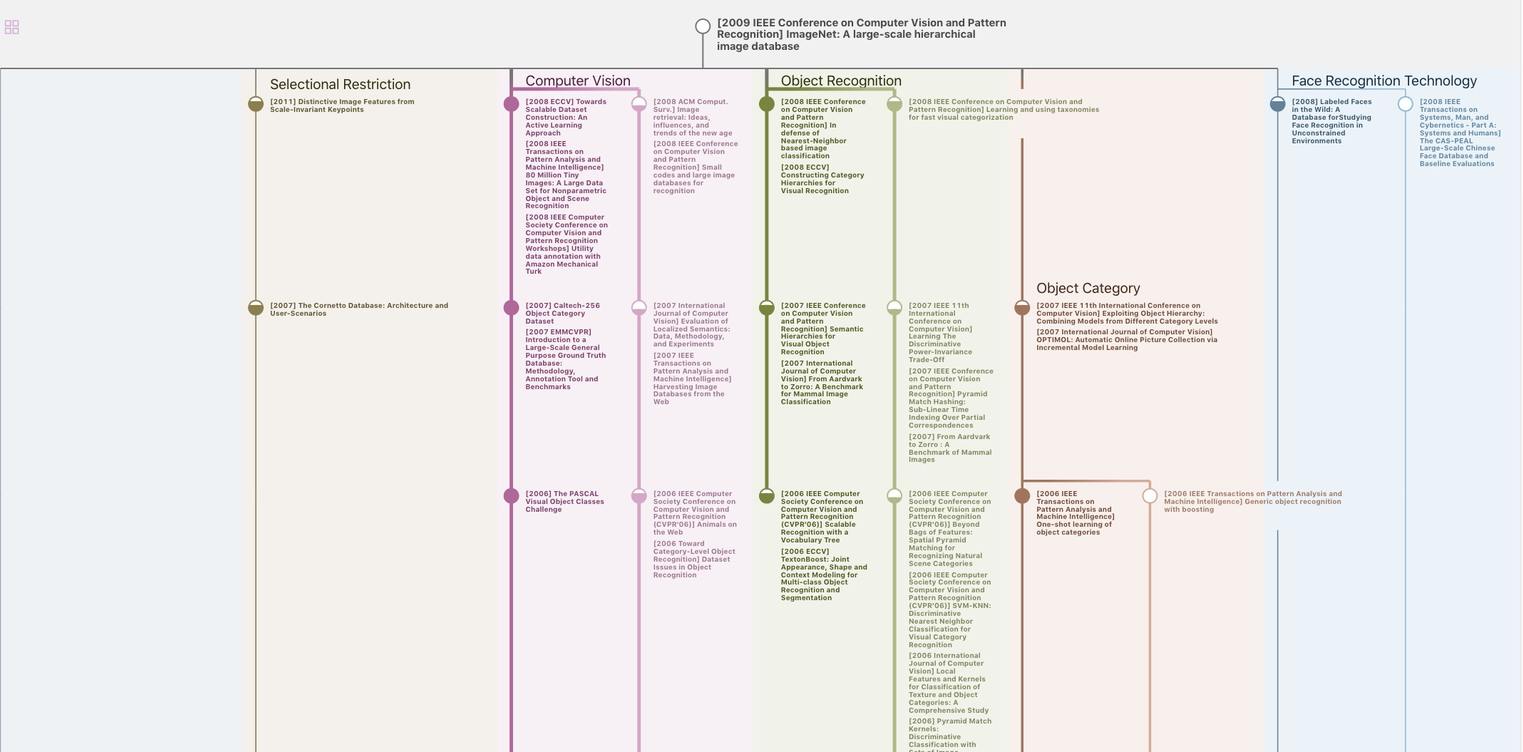
生成溯源树,研究论文发展脉络
Chat Paper
正在生成论文摘要