Lightweight image super-resolution based on deep learning: State-of-the-art and future directions.
Inf. Fusion(2023)
摘要
Recently, super-resolution (SR) techniques based on deep learning have taken more and more attention, aiming to improve the images and videos resolutions. Most of the SR methods are related to other fields of computer vision such as image classification, image segmentation, and object detection. Based on the success of the image SR task, many image SR surveys are introduced to summarize the recent work in the image SR domains. However, there is no survey to summarize the SR models for the lightweight image SR domain. In this paper, we present a comprehensive survey of the state-of-the-art lightweight SR models based on deep learning. The SR techniques are grouped into six major categories: include convolution, residual, dense, distillation, attention, and extremely lightweight based models. Also, we cover some other issues related to the SR task, such as benchmark datasets and metrics for performance evaluation. Finally, we discuss some future directions and open problems, that may help other community researchers in the future.
更多查看译文
关键词
Lightweight image super-resolution,Convolution neural network,Attention mechanism,Model distillation
AI 理解论文
溯源树
样例
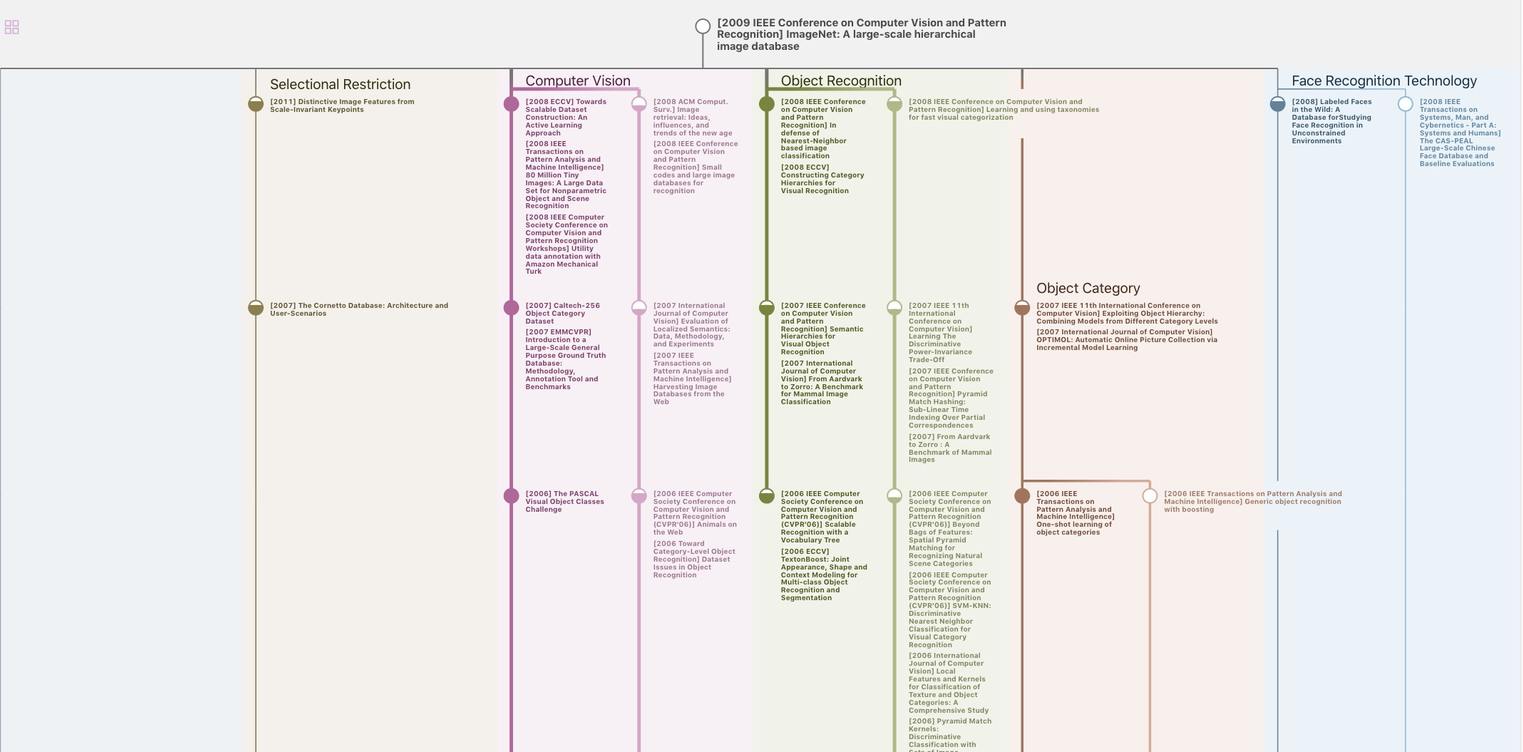
生成溯源树,研究论文发展脉络
Chat Paper
正在生成论文摘要