CCVAE: A Variational Autoencoder for Handling Censored Covariates.
ICMLA(2022)
摘要
For time or safety critical scenarios when faulty predictions or decisions can have crucial consequences, such as in certain telecommunications scenarios, reliable prediction models and accurate data are of the essence. When modeling and predicting data in such scenarios, data with censored covariates remain an issue as ignoring them or imputing them with lack of precision may cause inaccurate or uncertain predictions. In this paper, we provide a fast, reliable Variational Autoencoder framework which can handle covariate censoring in high dimensional data. Our numerical experiments demonstrate that our framework compares favorably to alternative methods in terms of prediction accuracy for both the response and the covariates, while enabling estimation of the prediction uncertainties. We moreover demonstrate that the method is at least 8 times faster than the benchmark models used in this paper, and more robust to initial imputations and noise than existing models. The method can also be used directly for predicting unseen data, which is a challenge for some state-of-the-art methods.
更多查看译文
关键词
variational autoencoder,censored covariates,zero inflated truncated normal,deep learning,dynamic range
AI 理解论文
溯源树
样例
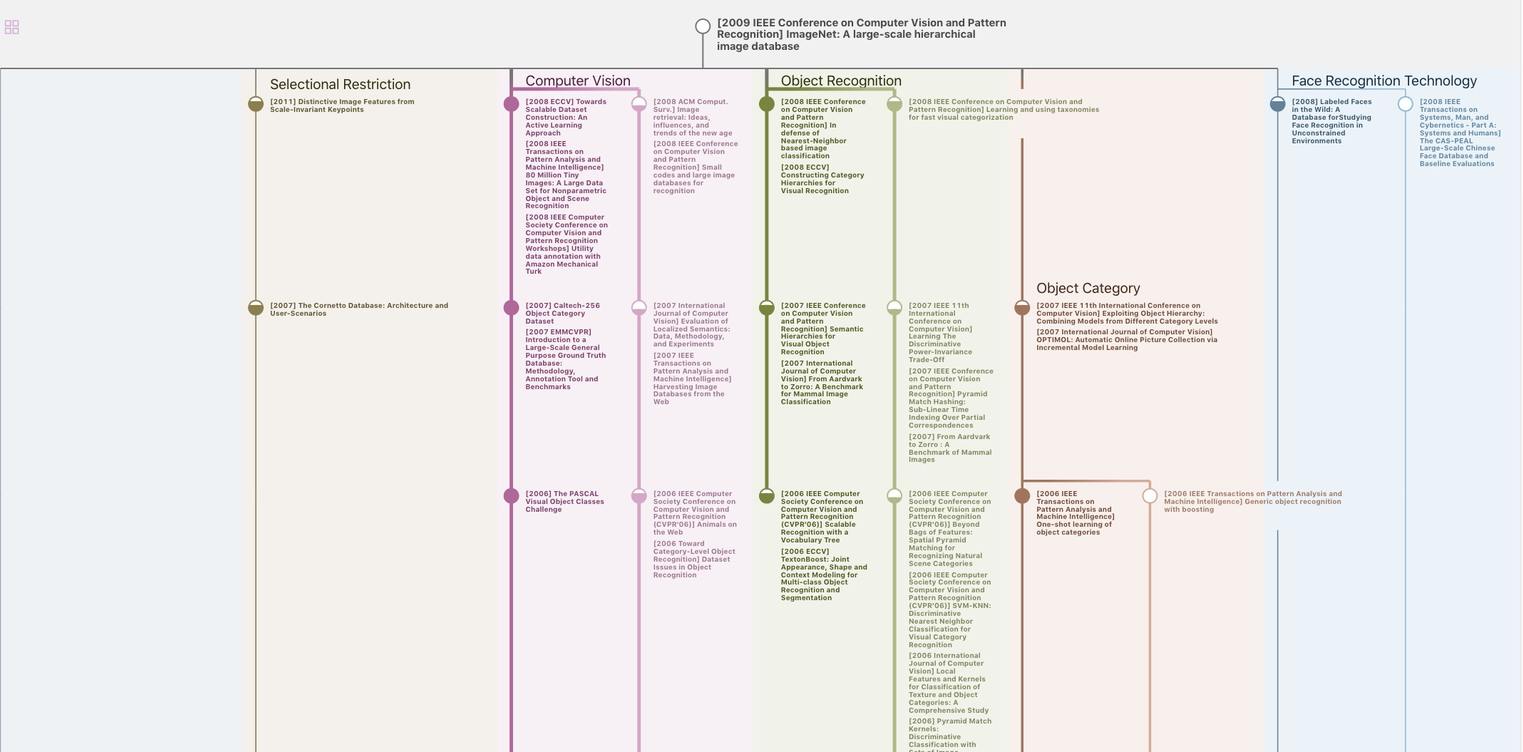
生成溯源树,研究论文发展脉络
Chat Paper
正在生成论文摘要