A CNN-Based Automated Stuttering Identification System
ICMLA(2022)
摘要
Stuttering can affect quality of life, resulting in poor social, emotional, and mental health status. Stuttering is diagnosed and managed by speech language pathologists, who are scarce in developing countries. We propose a novel CNN-based Automated Stuttering Identification System (ASIS) to help speech pathologists autonomously diagnose, classify, and log fluency disorders (blocks, prolongations, sound repetitions, word repetitions, and interjections), and monitor patient’s fluency progress over time. A baseline CNN model was created in Tensorflow/Keras and trained and tested using the Sep-28k dataset, an annotated stuttering database of 28,000 3-second clips. We built individual models for each disfluency label and measured accuracy, precision, recall, and F1 measure. The models were built five times, and the averages were taken of each metric. Three different training-validation-test splits were used: 80-10-10, 70-20-10, and 60-20-20. The models performed very well on the public dataset, exceeding the accuracy and F1 measure of other classifiers. The proposed ASIS can help speech pathologists improve the quality of life of stutterers especially in developing countries immensely, and thus it can make a significant difference for millions around the world.
更多查看译文
关键词
Stuttering,TensorFlow,CNN,Speech Disfluency,Automatic Speech Recognition
AI 理解论文
溯源树
样例
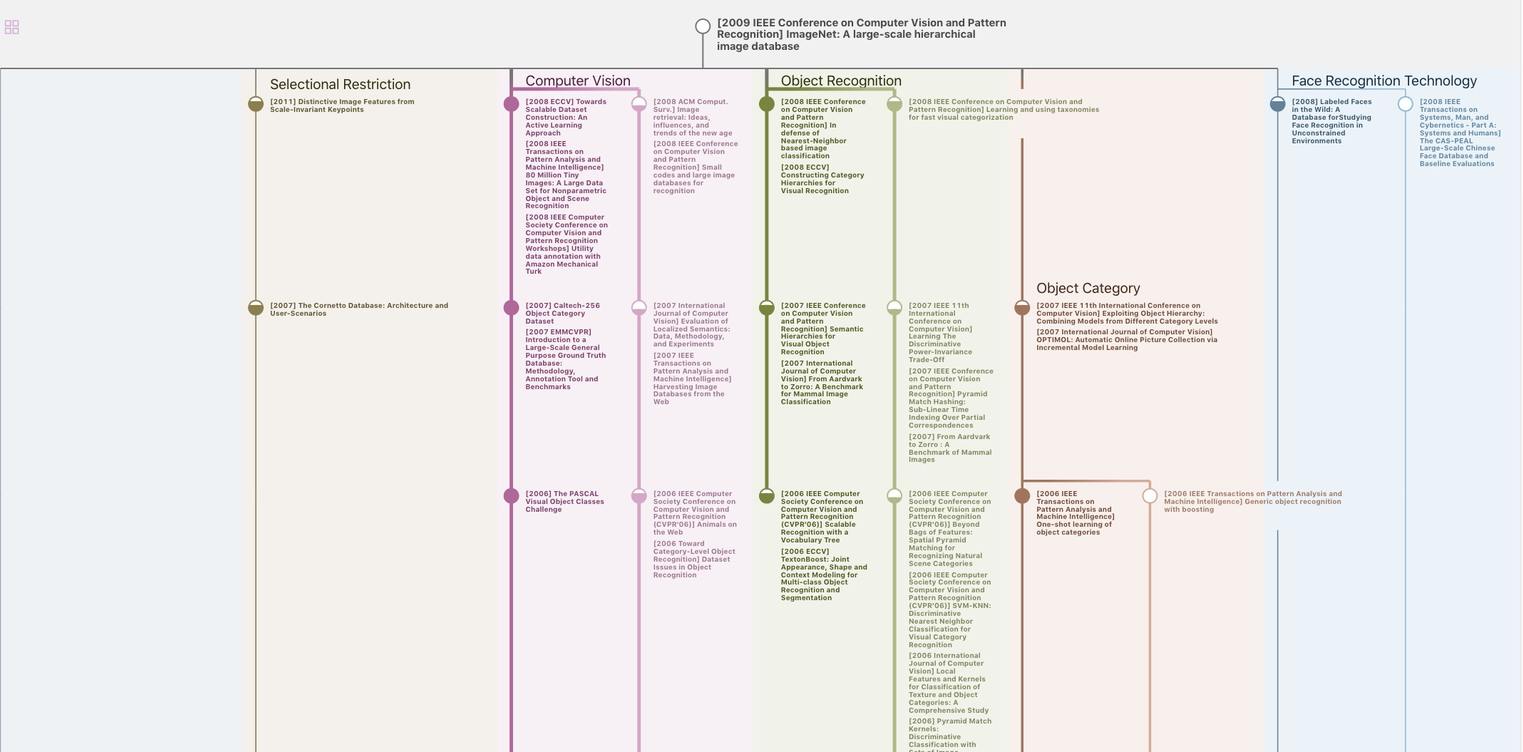
生成溯源树,研究论文发展脉络
Chat Paper
正在生成论文摘要