Learning Non-linear White-box Predictors: A Use Case in Energy Systems.
ICMLA(2022)
摘要
Many applications in energy systems require models that represent the non-linear dynamics of the underlying systems. Black-box models with non-linear architecture are suitable candidates for modeling these systems; however, they are computationally expensive and lack interpretability. An inexpensive white-box linear combination learned over a suitable polynomial feature set can result in a high-performing non-linear model that is easier to interpret, validate, and verify against reference models created by the domain experts. This paper proposes a workflow to learn a linear combination of non-linear terms for an engineered polynomial feature set. We firstly detect non-linear dependencies and then attempt to reconstruct them using feature expansion. Afterwards, we select possible predictors with the highest correlation coefficients for predictive regression analysis. We demonstrate how to learn inexpensive yet comprehensible linear combinations of non-linear terms from four datasets. Experimental evaluations show our workflow yields improvements in the metrics R
2
, CV-RMSE and MAPE in all datasets. Further evaluation of the learned models’ goodness of fit using prediction error plots also confirms that the proposed workflow results in models that can more accurately capture the nature of the underlying physical systems.
更多查看译文
关键词
Non-linear Regression,Polynomial Expansion,Feature Engineering,Energy Consumption Prediction,Energy
AI 理解论文
溯源树
样例
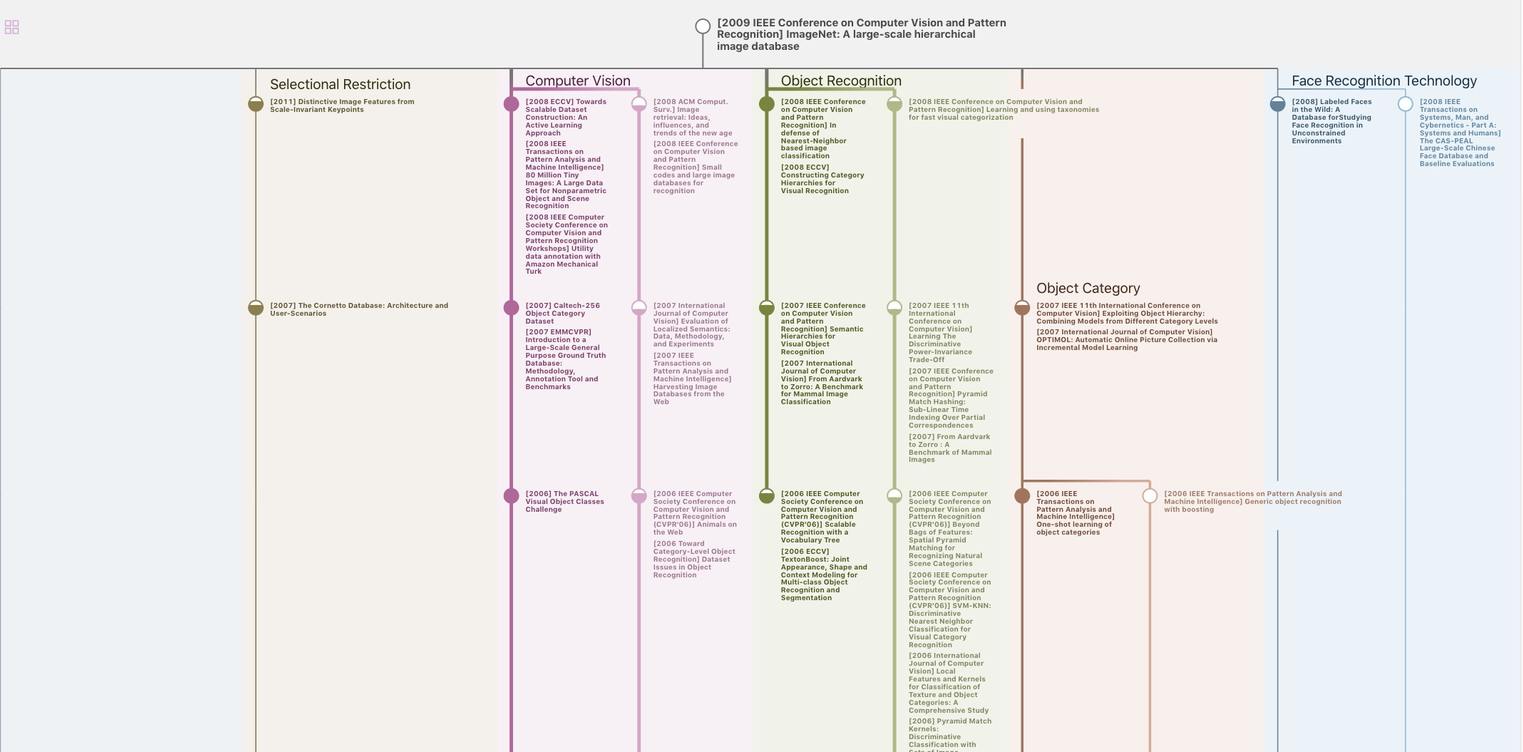
生成溯源树,研究论文发展脉络
Chat Paper
正在生成论文摘要