TSEvo: Evolutionary Counterfactual Explanations for Time Series Classification.
2022 21ST IEEE INTERNATIONAL CONFERENCE ON MACHINE LEARNING AND APPLICATIONS, ICMLA(2022)
摘要
With the increasing predominance of deep learning methods on time series classification, interpretability becomes essential, especially in high-stake scenarios. Although many approaches to interpretability have been explored for images and tabular data, time series data has been mostly neglected. We approach the problem of interpretability by proposing TSEvo, a model-agnostic multiobjective evolutionary approach to time series counterfactuals incorporating a variety of time series transformation mechanisms to cope with different types and structures of time series. We evaluate our framework on both uni- and multivariate benchmark datasets.
更多查看译文
关键词
time series interpretability,counterfactuals,interpretable machine learning
AI 理解论文
溯源树
样例
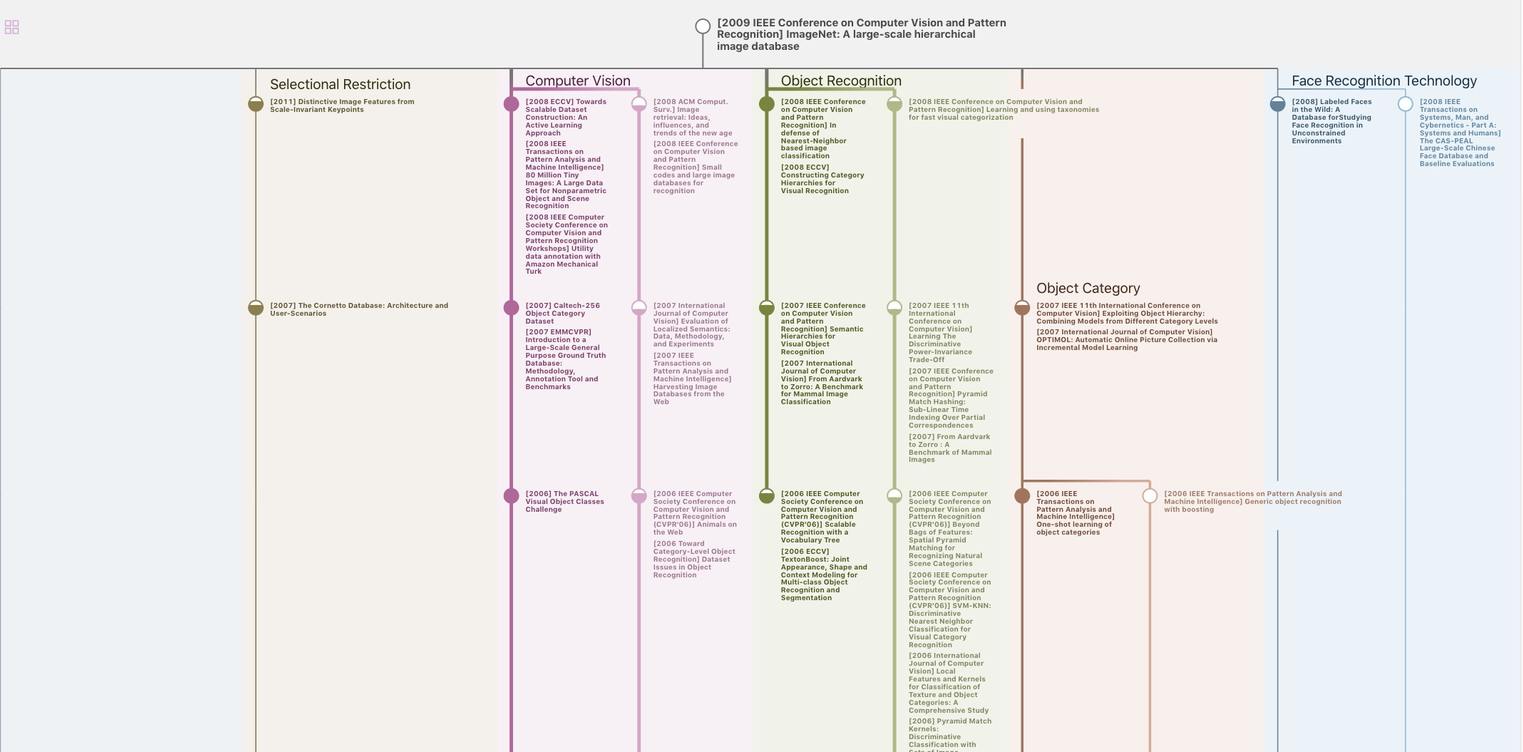
生成溯源树,研究论文发展脉络
Chat Paper
正在生成论文摘要