Exploiting Prototypical Explanations for Undersampling Imbalanced Datasets.
ICMLA(2022)
摘要
Among the reported solutions to the class imbalance issue, the undersampling approaches, which remove instances of insignificant samples from the majority class, are quite prevalent. However, the undersampling approaches may discard significant patterns in the datasets. A prototype, which is always an actual sample from the data, represents a group of samples in the dataset. Our hypothesis is that prototypes can fill the missing significant patterns that are discarded by undersampling methods and help to improve model performance. To confirm our intuition, we articulate prototypes to undersampling methods in the machine learning pipeline. We show that there is a statistically significant difference between the AUPR and AUROC results of undersampling methods and our approach.
更多查看译文
关键词
Prototypical Explanations,Undersampling,Imbalanced Datasets
AI 理解论文
溯源树
样例
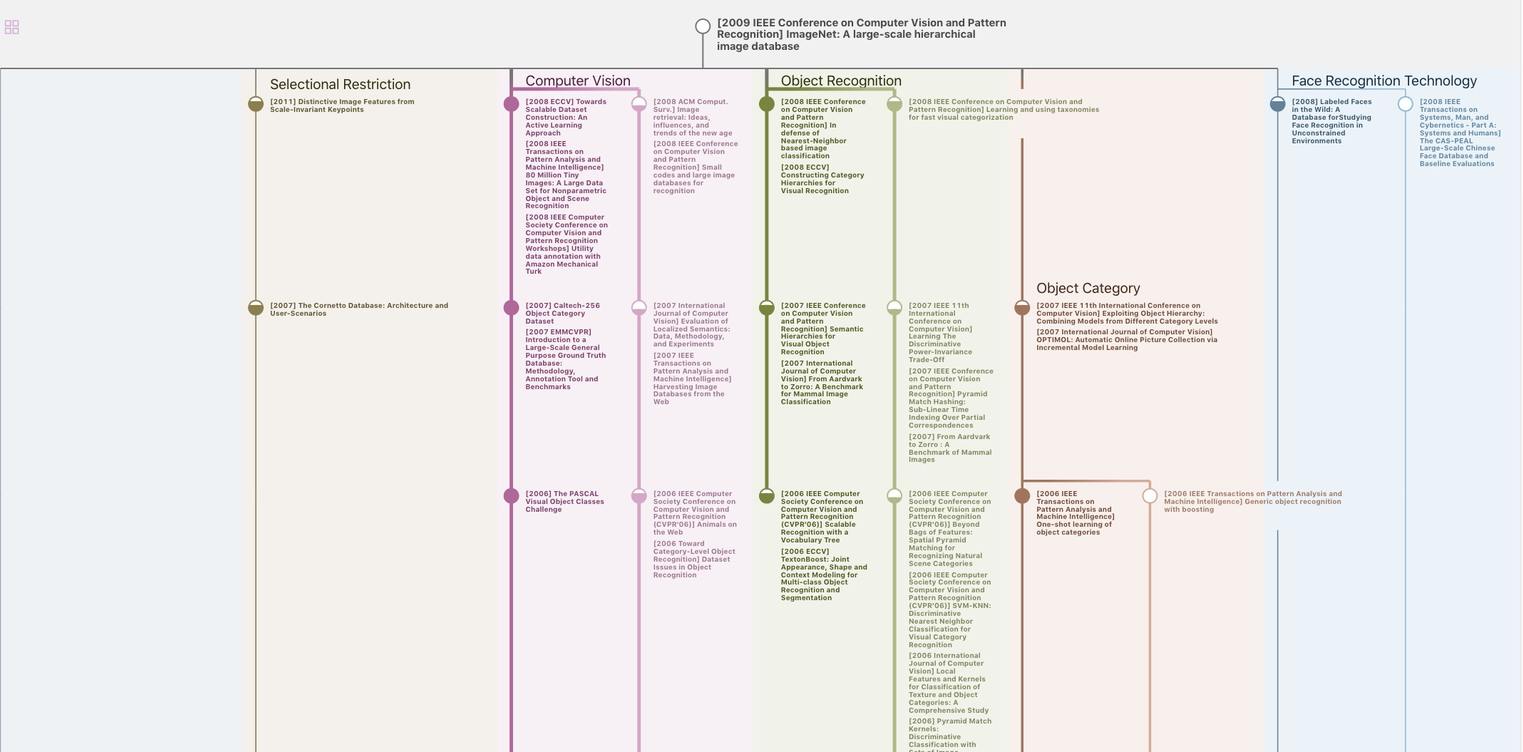
生成溯源树,研究论文发展脉络
Chat Paper
正在生成论文摘要