Modeling forest above-ground biomass using freely available satellite and multisource datasets.
Ecol. Informatics(2023)
摘要
Achieving the 2 °C global warming target will require all stakeholders, from local to global, to accelerate carbon reduction plans. To properly estimate carbon amounts sequestered and stored by forests, these stakeholders need accurate maps of forest above-ground biomass (AGB). However, AGB maps are developed solely by researchers using methodologies that are typically difficult for non-researchers to understand. To ensure that non-researchers can prepare such maps, freely available open-source datasets are needed along with a simple AGB estimation method. Remote sensing data have become widely used, and some satellite images are provided as open-source data. However, few AGB estimation studies have relied exclusively on freely available datasets. This study, therefore, examined the capability of using open-source satellite and multisource datasets to estimate AGB for two contrasting test sites in Hokkaido, Japan, and for the two sites combined (the unified sites). Test site 1 contained three forest types on a hilly terrain, while test site 2 contained one dominant forest type on a flat terrain. The datasets used were L-band backscatter, a canopy height model, slope, aspect, annual mean temperature, annual precipitation, and forest type. Three machine-learning algorithms and one parametric algorithm were compared. The results showed that the machine-learning models outperformed the parametric model in all instances, reproducing low and high AGB values properly with reduced saturation issues. A variable importance analysis revealed that in the best-performing machine-learning models, forest type and annual precipitation were the two most important variables for test site 1 and the unified sites, and another climatic factor, annual mean temperature, rose in rank for the unified sites. These variables had relatively large intra-variability and inter-variability in common, both of which appear to have offered advantages in modeling AGB for the sites where AGB variability was large. In contrast, the synthetic aperture radar (SAR)-based variables (the backscatter and the canopy height model) ranked lower in relative importance. This may reflect the negative influence of hilly terrain of test site 1 (and the unified sites) on the precision of SAR-based datasets. For test site 2, with its flat terrain, these SAR-based variables were the two dominant variables. Our results show the promise of freely available satellite and multisource datasets for modeling AGB and the important roles that non-SAR-based variables may play in large-scale AGB mapping that includes varied terrain.
更多查看译文
关键词
Above-ground biomass,Multisource data,Open-source data,Machine learning,Variable importance,Non-researchers
AI 理解论文
溯源树
样例
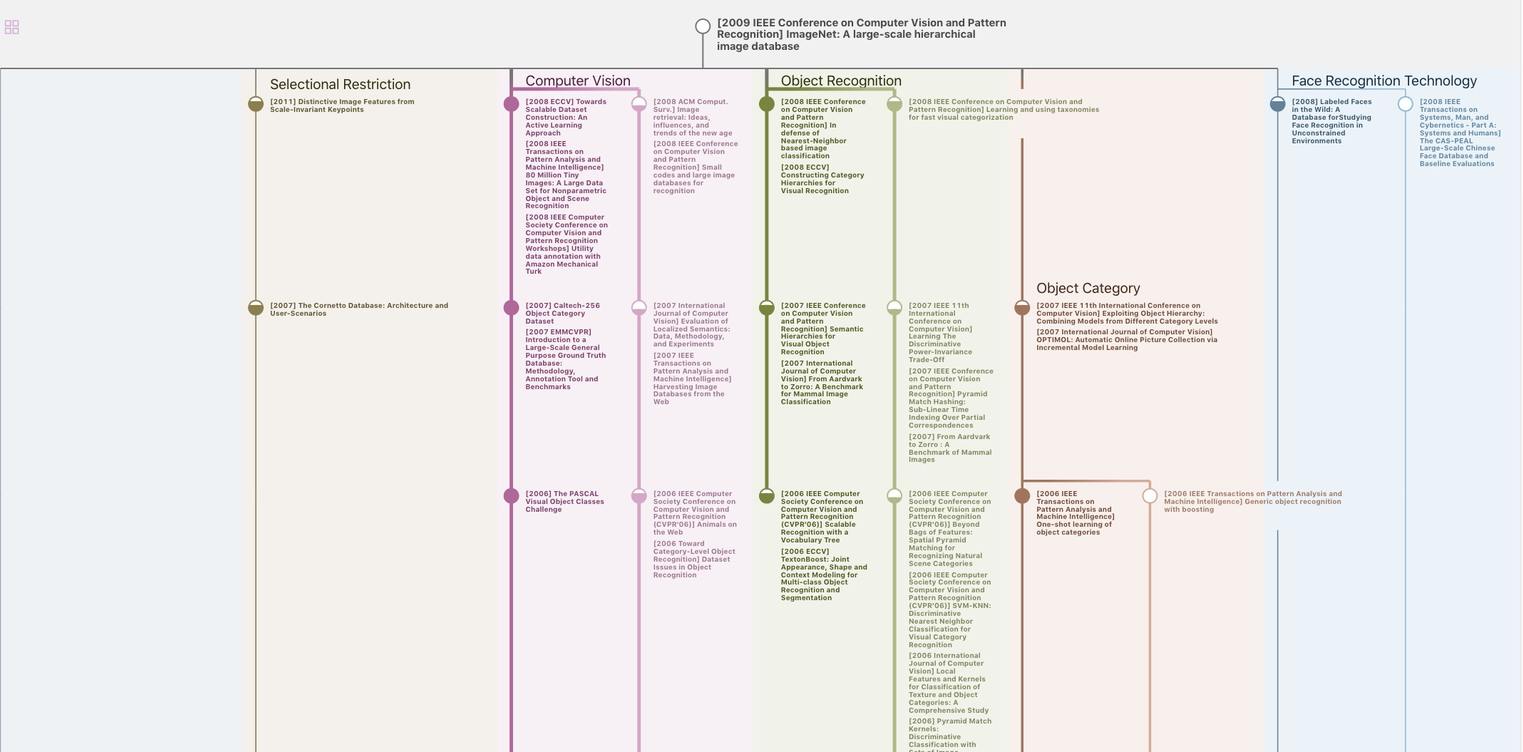
生成溯源树,研究论文发展脉络
Chat Paper
正在生成论文摘要