Few-shot learning with transformers via graph embeddings for molecular property prediction
Expert Systems with Applications(2023)
摘要
Molecular property prediction is an essential task in drug discovery. Recently, deep neural networks have accelerated the discovery of compounds with improved molecular profiles for effective drug development. In particular, graph neural networks (GNNs) have played a pivotal role in identifying promising drug candidates with desirable molecular properties. However, it is common for only a few molecules to share the same set of properties, which presents a low-data problem unanswered by regular machine learning (ML) approaches. Transformer networks have also emerged as a promising solution to model the long-range dependence in molecular embeddings and achieve encouraging results across a wide range of molecular property prediction tasks. Nonetheless, these methods still require a large number of data points per task to achieve acceptable performance. In this study, we propose a few-shot GNN-Transformer architecture, FS-GNNTR to face the challenge of low-data in molecular property prediction. The proposed model accepts molecules in the form of molecular graphs to model the local spatial context of molecular graph embeddings while preserving the global information of deep representations. Furthermore, we introduce a two-module meta-learning framework to iteratively update model parameters across few-shot tasks and predict new molecular properties with limited available data. Finally, we conduct multiple experiments on small-sized biological datasets for molecular property prediction, Tox21 and SIDER, and our results demonstrate the superior performance of FS-GNNTR compared to simpler graph-based baselines. The code and data underlying this article are available in the repository, https://github.com/ltorres97/FS-GNNTR.
更多查看译文
关键词
Few-shot learning, Transformer, Graph neural networks, Graph embedding, Molecular property prediction
AI 理解论文
溯源树
样例
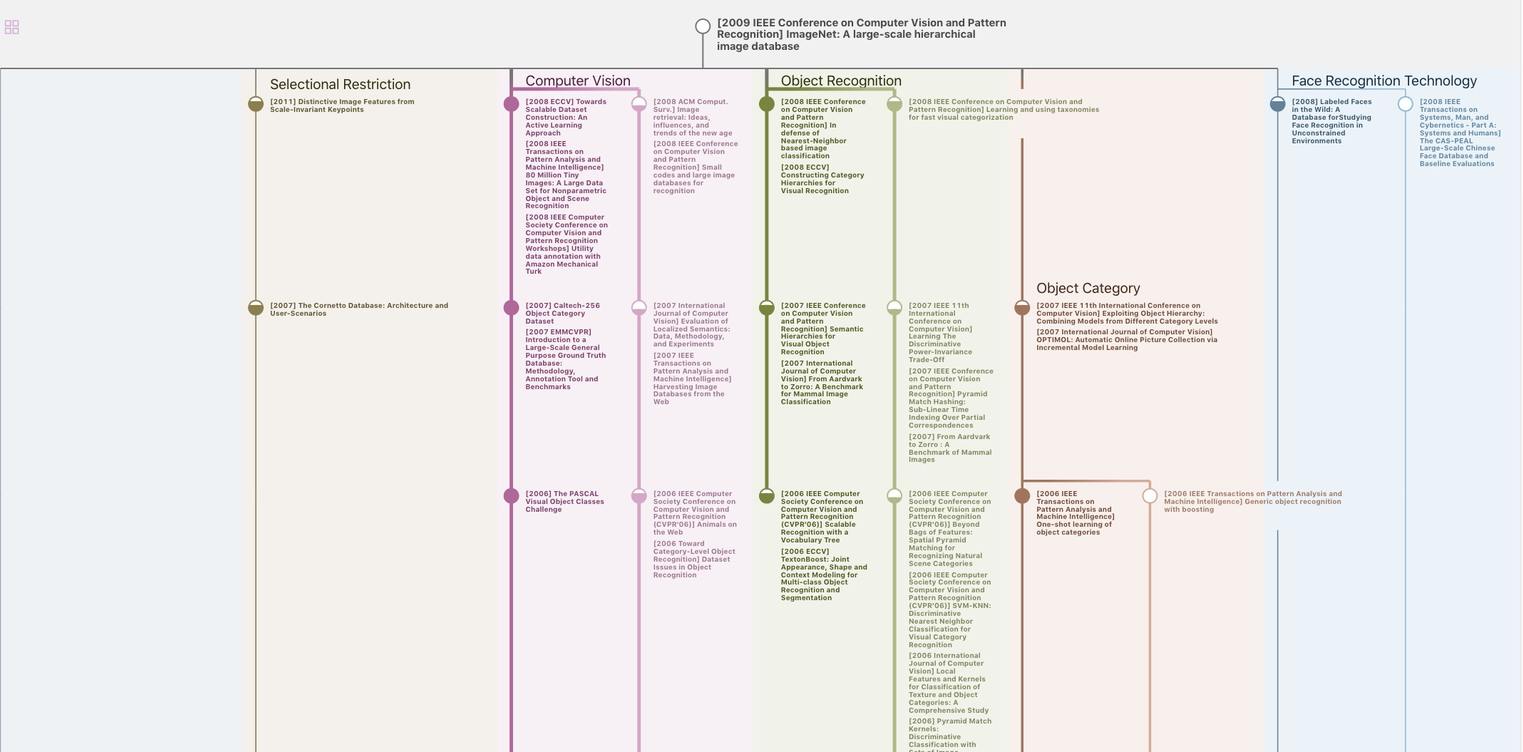
生成溯源树,研究论文发展脉络
Chat Paper
正在生成论文摘要