Spatial transcriptomics analysis of gene expression prediction using exemplar guided graph neural network
PATTERN RECOGNITION(2024)
摘要
Spatial transcriptomics (ST) is essential for understanding diseases and developing novel treatments. It measures the gene expression of each fine-grained area (i.e., different windows) in the tissue slide with low throughput. This paper proposes an exemplar guided graph network dubbed EGGN to accurately and efficiently predict gene expression from each window of a tissue slide image. We apply exemplar learning to dynamically boost gene expression prediction from nearest/similar exemplars of a given tissue slide image window. Our framework has three main components connected in a sequence: (i) an extractor to structure a feature space for exemplar retrievals; (ii) a graph construction strategy to connect windows and exemplars as a graph; (iii) a graph convolutional network backbone to process window and exemplar features, and a graph exemplar bridging block to adaptively revise the window features using its exemplars. Finally, we complete the gene expression prediction task with a simple attention-based prediction block. Experiments on standard benchmark datasets indicate the superiority of our approach when compared with past state-of-the-art methods. We release our code at https://github.com/Yan98/EGN.
更多查看译文
关键词
Spatial transcriptomics,Gene expression prediction,Deep learning,Graph convolution,Tissue slide image
AI 理解论文
溯源树
样例
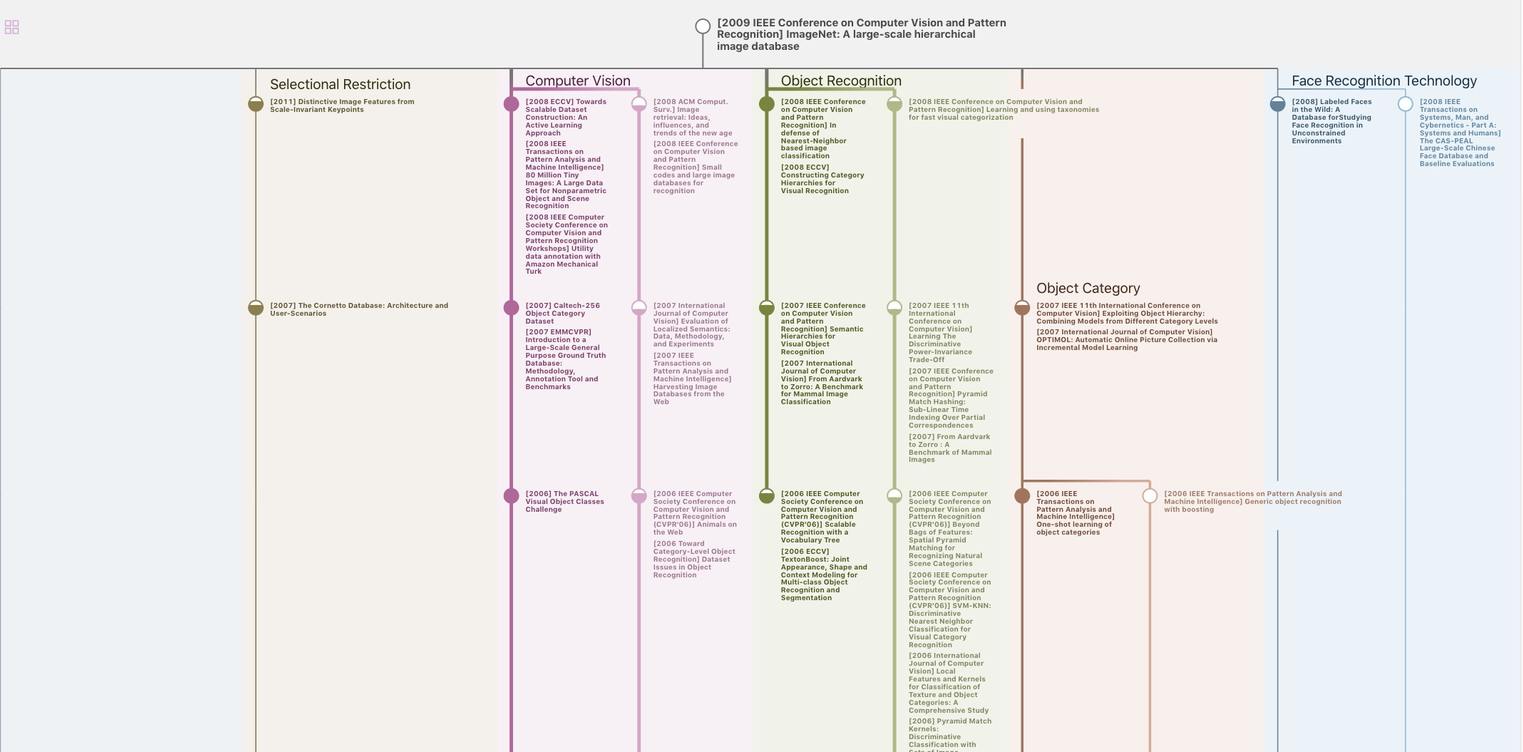
生成溯源树,研究论文发展脉络
Chat Paper
正在生成论文摘要