Practical Policy Optimization with Personalized Experimentation
CoRR(2023)
摘要
Many organizations measure treatment effects via an experimentation platform to evaluate the casual effect of product variations prior to full-scale deployment. However, standard experimentation platforms do not perform optimally for end user populations that exhibit heterogeneous treatment effects (HTEs). Here we present a personalized experimentation framework, Personalized Experiments (PEX), which optimizes treatment group assignment at the user level via HTE modeling and sequential decision policy optimization to optimize multiple short-term and long-term outcomes simultaneously. We describe an end-to-end workflow that has proven to be successful in practice and can be readily implemented using open-source software.
更多查看译文
关键词
personalized experimentation,policy,optimization
AI 理解论文
溯源树
样例
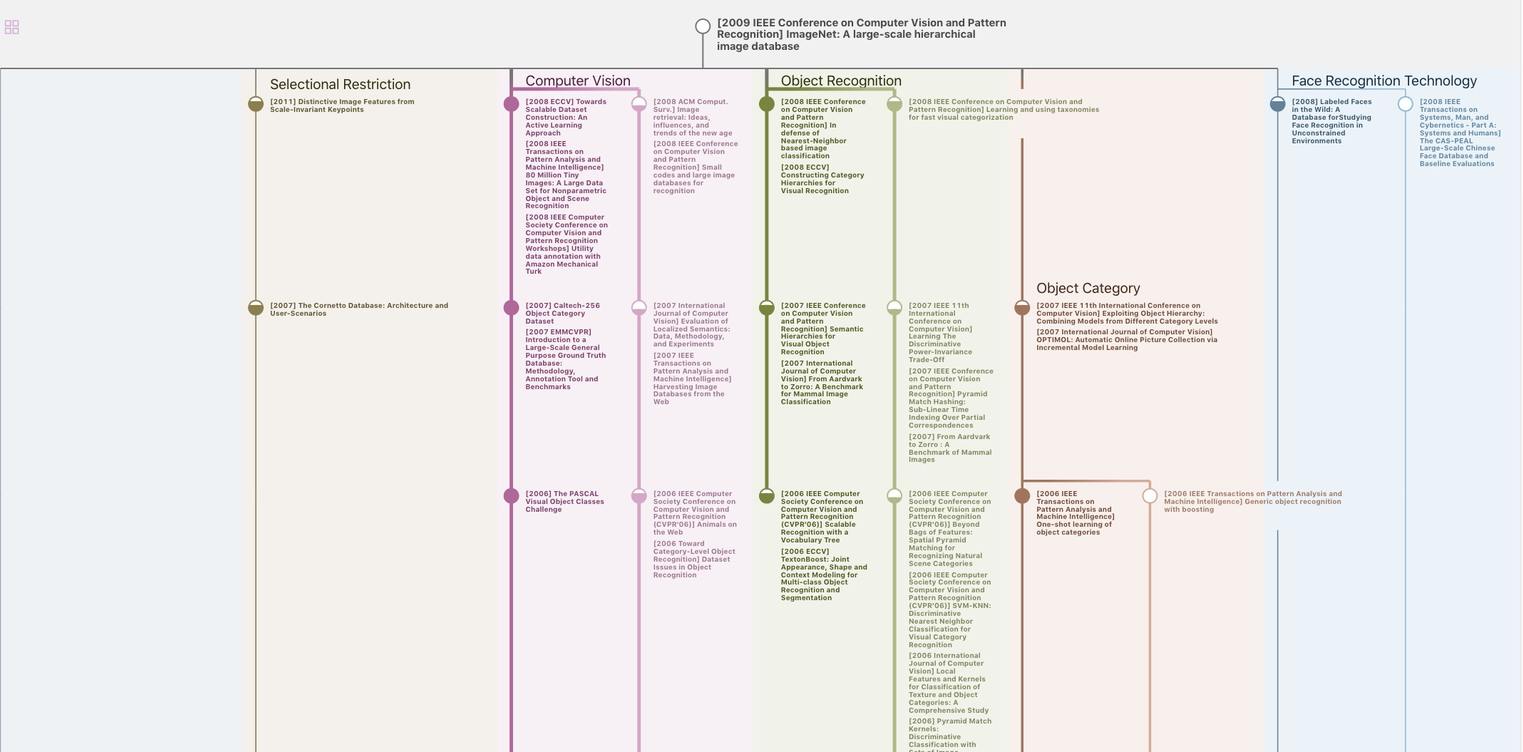
生成溯源树,研究论文发展脉络
Chat Paper
正在生成论文摘要