Scalable Bayesian Meta-Learning through Generalized Implicit Gradients
CoRR(2023)
摘要
Meta-learning owns unique effectiveness and swiftness in tackling emerging tasks with limited data. Its broad applicability is revealed by viewing it as a bi-level optimization problem. The resultant algorithmic viewpoint however, faces scalability issues when the inner-level optimization relies on gradient-based iterations. Implicit differentiation has been considered to alleviate this challenge, but it is restricted to an isotropic Gaussian prior, and only favors deterministic meta-learning approaches. This work markedly mitigates the scalability bottleneck by cross-fertilizing the benefits of implicit differentiation to probabilistic Bayesian meta-learning. The novel implicit Bayesian meta-learning (iBaML) method not only broadens the scope of learnable priors, but also quantifies the associated uncertainty. Furthermore, the ultimate complexity is well controlled regardless of the inner-level optimization trajectory. Analytical error bounds are established to demonstrate the precision and efficiency of the generalized implicit gradient over the explicit one. Extensive numerical tests are also carried out to empirically validate the performance of the proposed method.
更多查看译文
关键词
generalized implicit gradients,meta-learning
AI 理解论文
溯源树
样例
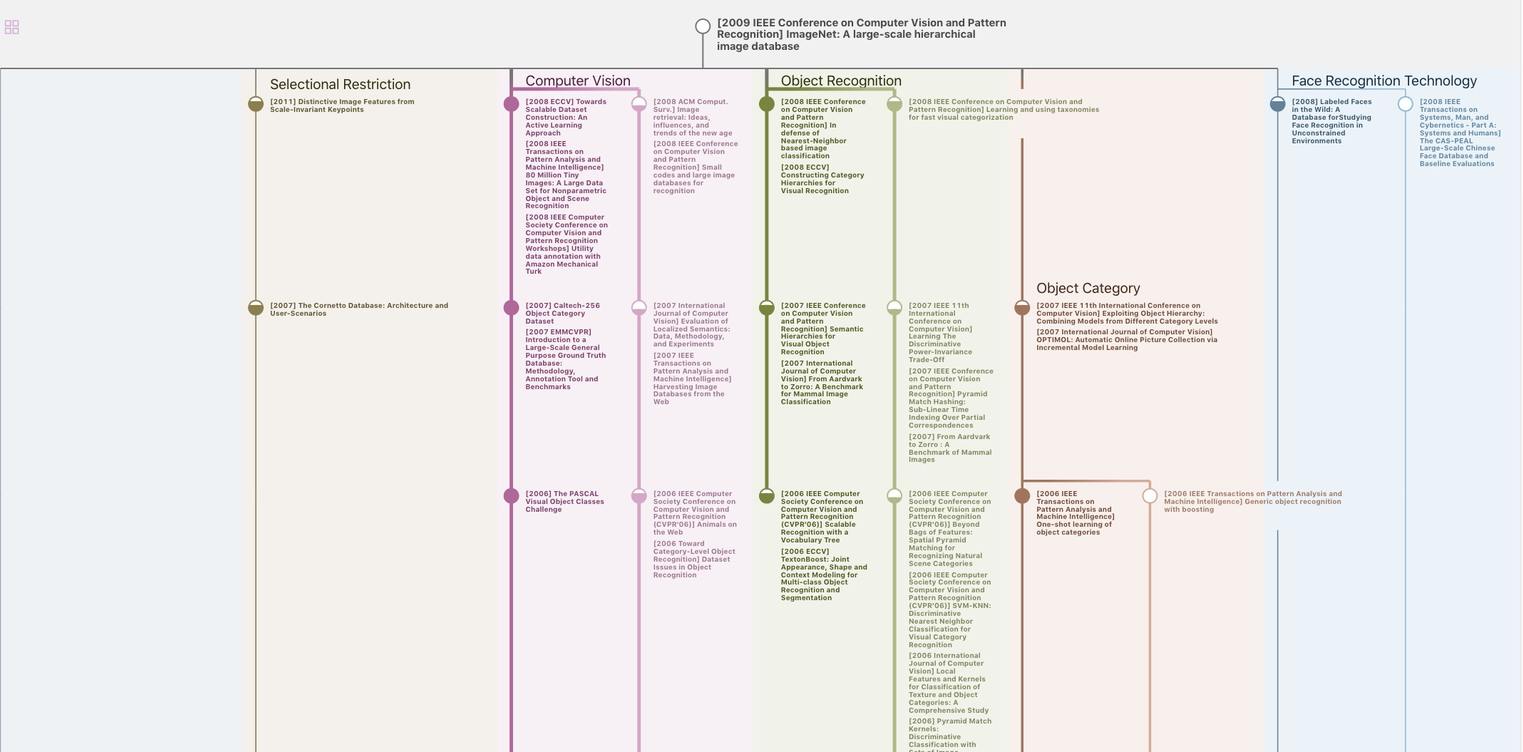
生成溯源树,研究论文发展脉络
Chat Paper
正在生成论文摘要