Complexity analysis of weakly noisy quantum states via quantum machine learning
arXiv (Cornell University)(2023)
摘要
Quantum computers capable of fault-tolerant operation are expected to provide provable advantages over classical computational models. However, the question of whether quantum advantages exist in the noisy intermediate-scale quantum era remains a fundamental and challenging problem. The root of this challenge lies in the difficulty of exploring and quantifying the power of noisy quantum states. In this work, we focus on the complexity of weakly noisy states, which we define as the size of the shortest quantum circuit required to prepare the noisy state. To analyze this complexity, we first establish a general relationship between circuit depth, noise model, and purity. Based on this necessary condition, we propose a quantum machine learning (QML) algorithm that exploits the intrinsic-connection property of structured quantum neural networks. The proposed QML algorithm enables efficiently predicting the complexity of weakly noisy states from measurement results, representing a paradigm shift in our ability to characterize the power of noisy quantum computation.
更多查看译文
关键词
weakly noisy quantum states,quantum states,quantum machine
AI 理解论文
溯源树
样例
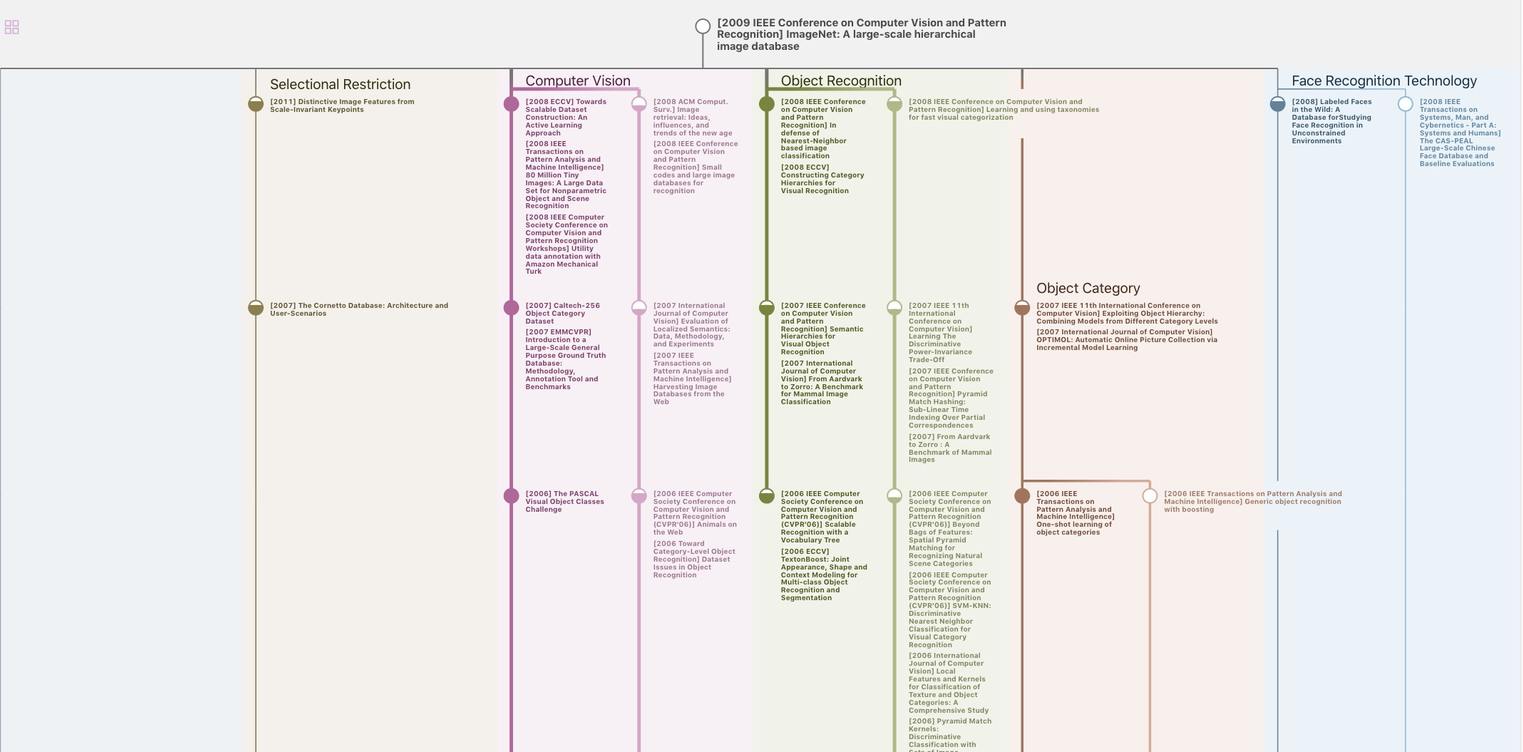
生成溯源树,研究论文发展脉络
Chat Paper
正在生成论文摘要