VDN-NeRF: Resolving Shape-Radiance Ambiguity via View-Dependence Normalization
CVPR 2023(2023)
Abstract
We propose VDN-NeRF, a method to train neural radiance fields (NeRFs) for better geometry under non-Lambertian surface and dynamic lighting conditions that cause significant variation in the radiance of a point when viewed from different angles. Instead of explicitly modeling the underlying factors that result in the view-dependent phenomenon, which could be complex yet not inclusive, we develop a simple and effective technique that normalizes the view-dependence by distilling invariant information already encoded in the learned NeRFs. We then jointly train NeRFs for view synthesis with view-dependence normalization to attain quality geometry. Our experiments show that even though shape-radiance ambiguity is inevitable, the proposed normalization can minimize its effect on geometry, which essentially aligns the optimal capacity needed for explaining view-dependent variations. Our method applies to various baselines and significantly improves geometry without changing the volume rendering pipeline, even if the data is captured under a moving light source. Code is available at: https://github.com/BoifZ/VDN-NeRF.
MoreTranslated text
Key words
3D from multi-view and sensors
AI Read Science
Must-Reading Tree
Example
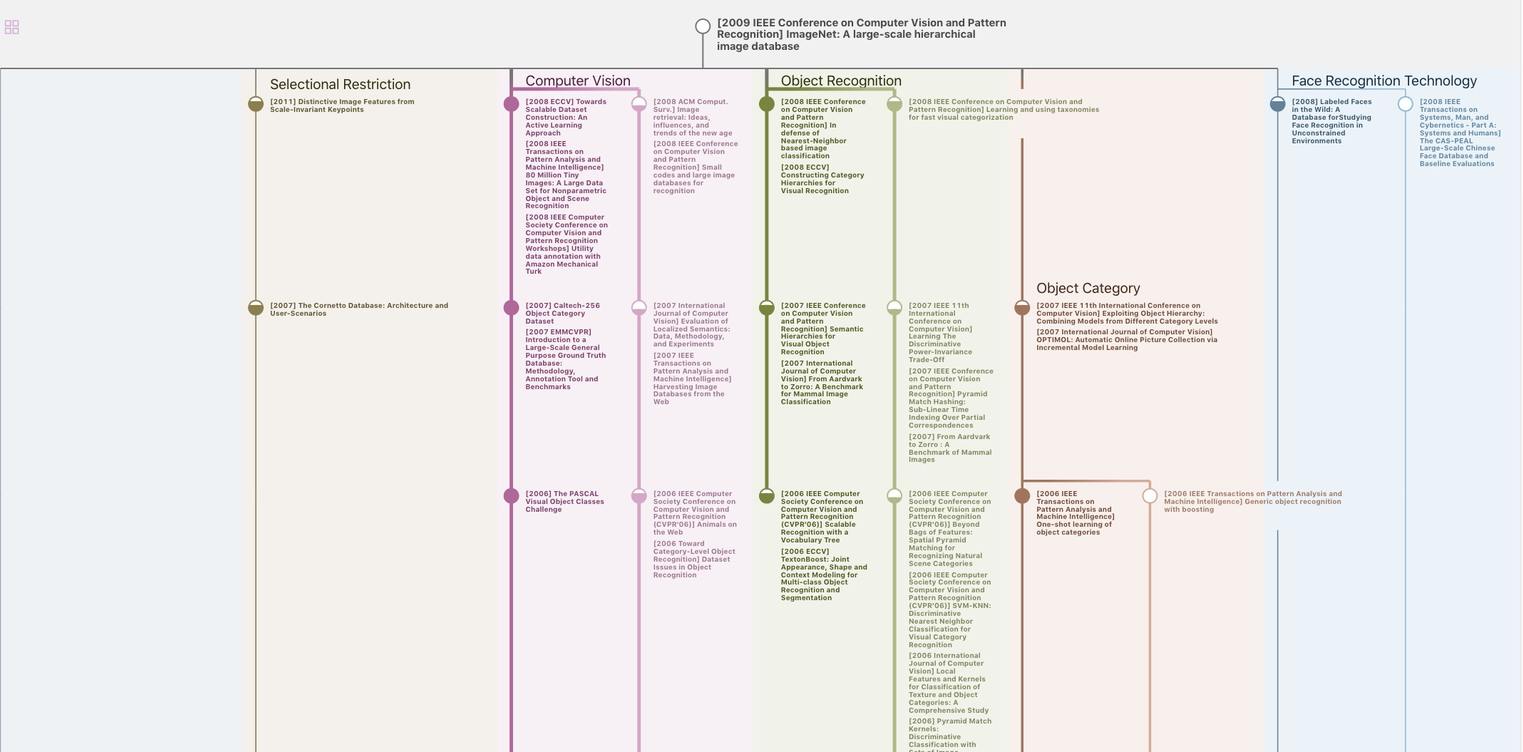
Generate MRT to find the research sequence of this paper
Chat Paper
Summary is being generated by the instructions you defined