Geometric constraints improve inference of sparsely observed stochastic dynamics
CoRR(2023)
摘要
The dynamics of systems of many degrees of freedom evolving on multiple scales are often modeled in terms of stochastic differential equations. Usually the structural form of these equations is unknown and the only manifestation of the system's dynamics are observations at discrete points in time. Despite their widespread use, accurately inferring these systems from sparse-in-time observations remains challenging. Conventional inference methods either focus on the temporal structure of observations, neglecting the geometry of the system's invariant density, or use geometric approximations of the invariant density, which are limited to conservative driving forces. To address these limitations, here, we introduce a novel approach that reconciles these two perspectives. We propose a path augmentation scheme that employs data-driven control to account for the geometry of the invariant system's density. Non-parametric inference on the augmented paths, enables efficient identification of the underlying deterministic forces of systems observed at low sampling rates.
更多查看译文
关键词
observed stochastic
AI 理解论文
溯源树
样例
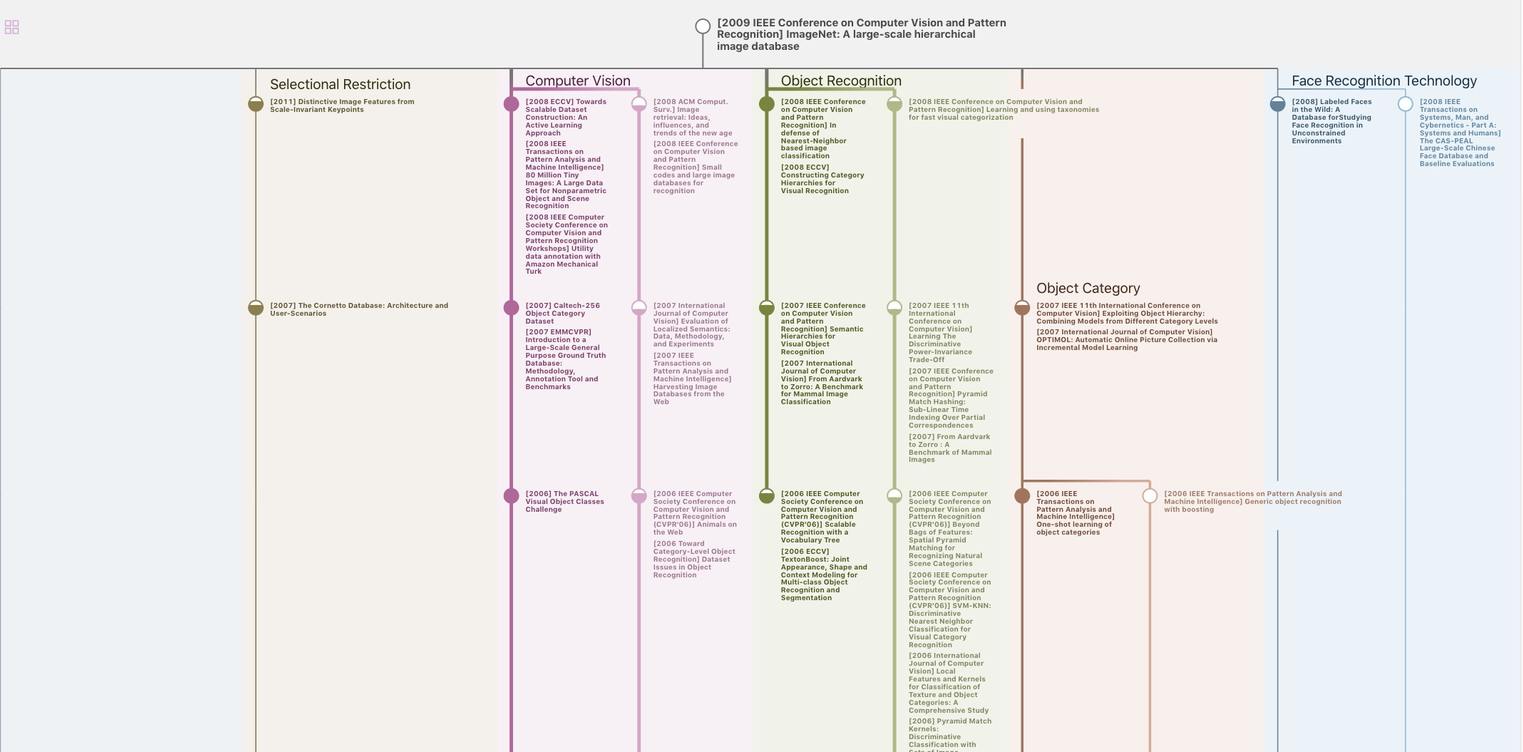
生成溯源树,研究论文发展脉络
Chat Paper
正在生成论文摘要