RunBugRun -- An Executable Dataset for Automated Program Repair
CoRR(2023)
摘要
Recently, we can notice a transition to data-driven techniques in Automated Program Repair (APR), in particular towards deep neural networks. This entails training on hundreds of thousands or even millions of non-executable code fragments. We would like to bring more attention to an aspect of code often neglected in Neural Program Repair (NPR), namely its execution. Code execution has several significant advantages. It allows for test-based evaluation of candidate fixes and can provide valuable information to aid repair. In this work we present a fully executable dataset of 450,000 small buggy/fixed program pairs originally submitted to programming competition websites written in eight different programming languages. Along with the dataset we provide infrastructure to compile, safely execute and test programs as well as fine-grained bug-type labels. To give a point of reference, we provide basic evaluation results for two baselines, one based on a generate-and-validate approach and one on deep learning. With this dataset we follow several goals: we want to lift Neural Program Repair beyond fully static code representations, foster the use of execution-based features and, by including several different languages, counterbalance the predominance of Java in the current landscape of APR datasets and benchmarks.
更多查看译文
关键词
executable dataset,program
AI 理解论文
溯源树
样例
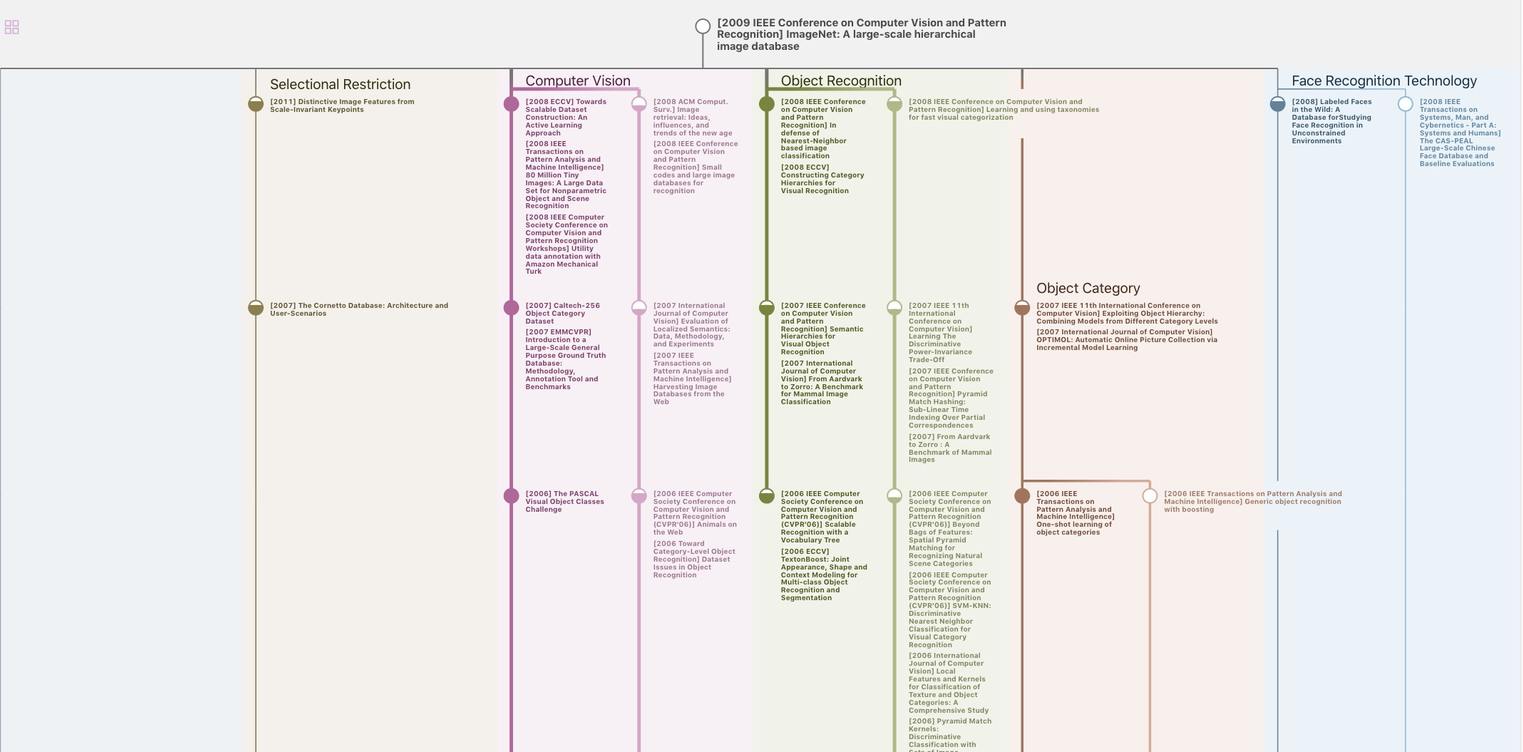
生成溯源树,研究论文发展脉络
Chat Paper
正在生成论文摘要