Direct prediction of carbapenem-resistant, carbapenemase-producing, and colistin-resistant Klebsiella pneumoniae isolates from routine MALDI-TOF mass spectra using machine learning and outcome evaluation.
International journal of antimicrobial agents(2023)
摘要
The objective of this study was to develop a rapid prediction method for carbapenem-resistant Klebsiella pneumoniae (CRKP) and colistin-resistant K. pneumoniae (ColRKP) based on routine MALDI-TOF mass spectrometry (MS) results in order to formulate a suitable and rapid treatment strategy. A total of 830 CRKP and 1462 carbapenem-susceptible K. pneumoniae (CSKP) isolates were collected; 54 ColRKP isolates and 1592 colistin-intermediate K. pneumoniae (ColIKP) isolates were also included. Routine MALDI-TOF MS, antimicrobial susceptibility testing, NG-Test CARBA 5, and resistance gene detection were followed by machine learning (ML). Using the ML model, the accuracy and area under the curve for differentiating CRKP and CSKP were 0.8869 and 0.9551, respectively, and those for ColRKP and ColIKP were 0.8361 and 0.8447, respectively. The most important MS features of CRKP and ColRKP were m/z 4520-4529 and m/z 4170-4179, respectively. Of the CRKP isolates, MS m/z 4520-4529 was a potential biomarker for distinguishing KPC from OXA, NDM, IMP, and VIM. Of the 34 patients who received preliminary CRKP ML prediction results (by texting), 24 (70.6%) were confirmed to have CRKP infection. The mortality rate was lower in patients who received antibiotic regimen adjustment based on the preliminary ML prediction (4/14, 28.6%). In conclusion, the proposed model can provide rapid results for differentiating CRKP and CSKP, as well as ColRKP and ColIKP. The combination of ML-based CRKP with preliminary reporting of results can help physicians alter the regimen approximately 24 h earlier, resulting in improved survival of patients with timely antibiotic intervention.
更多查看译文
AI 理解论文
溯源树
样例
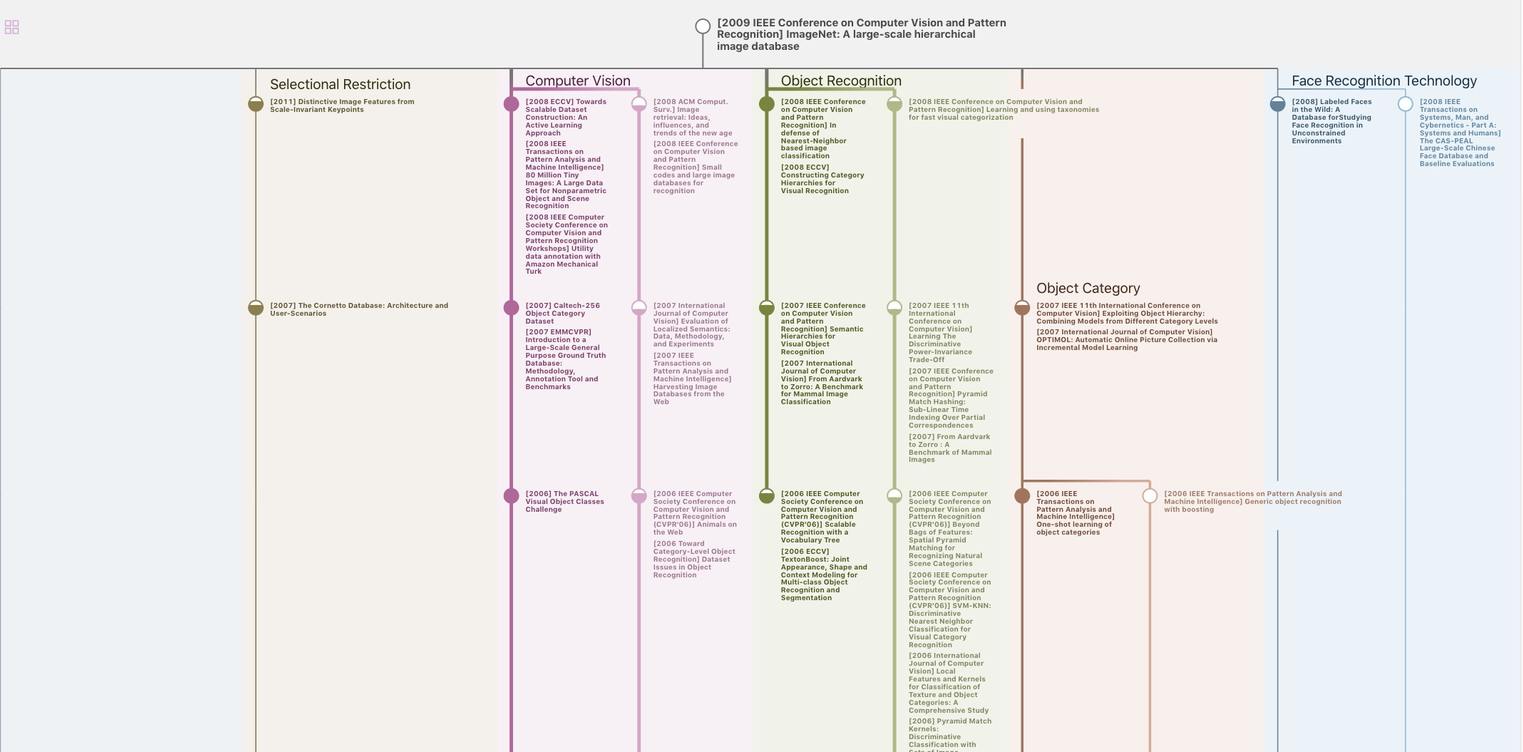
生成溯源树,研究论文发展脉络
Chat Paper
正在生成论文摘要