Prior Knowledge‐infused Neural Network for Efficient Performance Assessment of Structures Through Few‐shot Incremental Learning
Computer-aided civil and infrastructure engineering(2024)
摘要
Structural seismic safety assessment is a critical task in maintaining the resilience of existing civil and infrastructures. This task commonly requires accurate predictions of structural responses under stochastic intensive ground accelerations via time-costly numerical simulations. While numerous studies have attempted to use machine learning (ML) techniques as surrogate models to alleviate this computing burden, a large number of numerical simulations are still required for training ML models. Therefore, this study proposes a prior knowledge-infused neural network (PKNN) for achieving efficient structural seismic response predictions and seismic safety assessment at a low computation cost. In this approach, first, the prior knowledge inherently within a theoretical dynamic model of a structure is infused into a neural network. Then, by utilizing the few-shot incremental learning technique, this network would be further fine-tuned by only a few numerical simulations. The resulting PKNN would be able to accurately predict the seismic response of a structure and facilitate seismic safety assessment. In this study, the PKNN's accuracy and computational efficiency are validated on a typical frame structure. The results revealed that the proposed PKNN could be used for accurately predicting the structural seismic responses and assessing the structural safety under a low computational cost.
更多查看译文
AI 理解论文
溯源树
样例
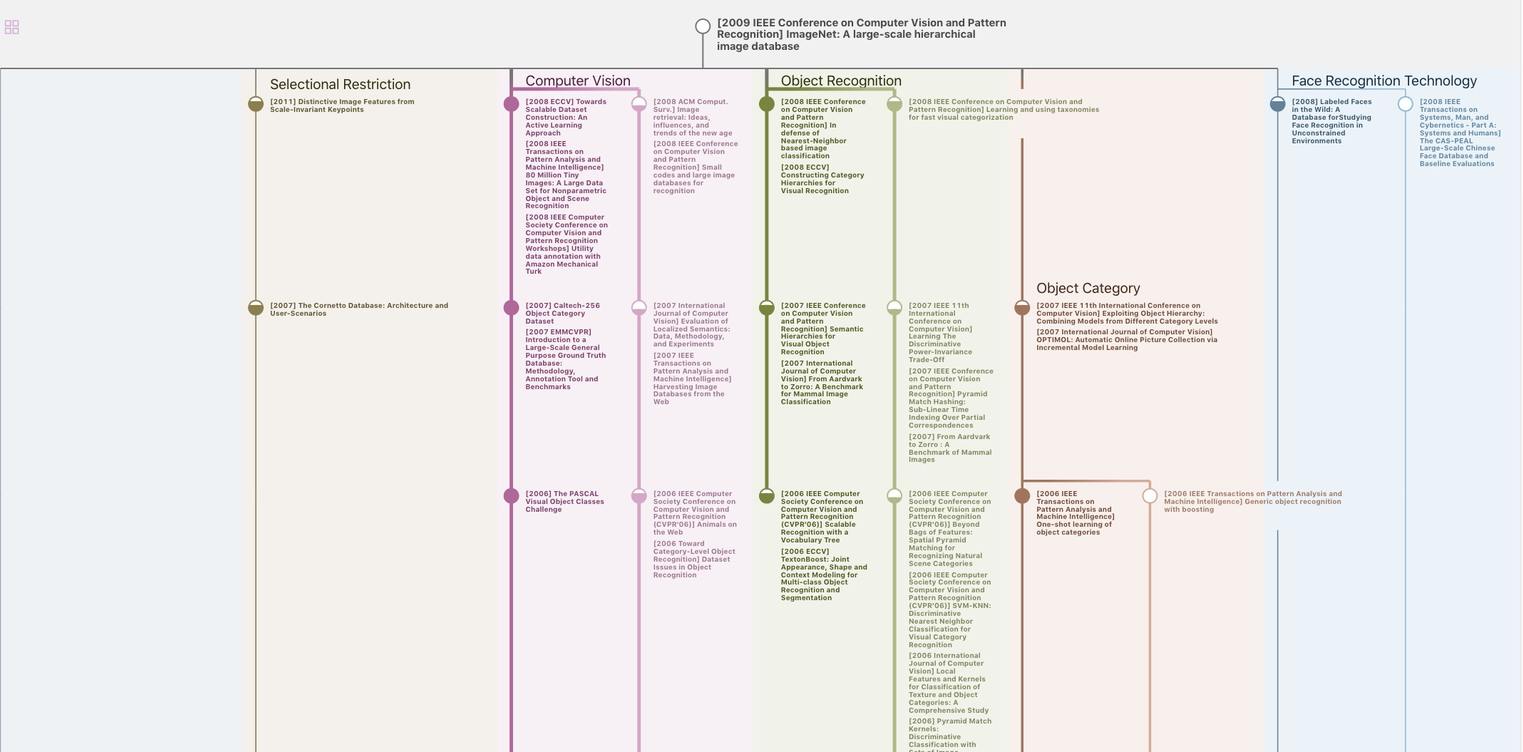
生成溯源树,研究论文发展脉络
Chat Paper
正在生成论文摘要