A study of distributionally robust mixed-integer programming with Wasserstein metric: on the value of incomplete data
EUROPEAN JOURNAL OF OPERATIONAL RESEARCH(2024)
摘要
This study addresses a class of linear mixed-integer programming (MILP) problems that involve uncertainty in the objective function parameters. The parameters are assumed to form a random vector, whose probability distribution can only be observed through a finite training data set. Unlike most of the related studies in the literature, we also consider uncertainty in the underlying data set. The data uncertainty is described by a set of linear constraints for each random sample, and the uncertainty in the distribution (for a fixed realization of data) is defined using a type-1 Wasserstein ball centered at the empirical distribution of the data. The overall problem is formulated as a three-level distributionally robust optimization (DRO) problem. First, we prove that the three-level problem admits a single-level MILP reformulation, if the class of loss functions is restricted to biaffine functions. Secondly, it turns out that for several particular forms of data uncertainty, the outlined problem can be solved reasonably fast by leveraging the nominal MILP problem. Finally, we conduct a computational study, where the out-of-sample performance of our model and computational complexity of the proposed MILP reformulation are explored numerically for several application domains.
更多查看译文
关键词
Uncertainty modeling,Distributionally robust optimization,Mixed-integer programming,Wasserstein metric,Incomplete data
AI 理解论文
溯源树
样例
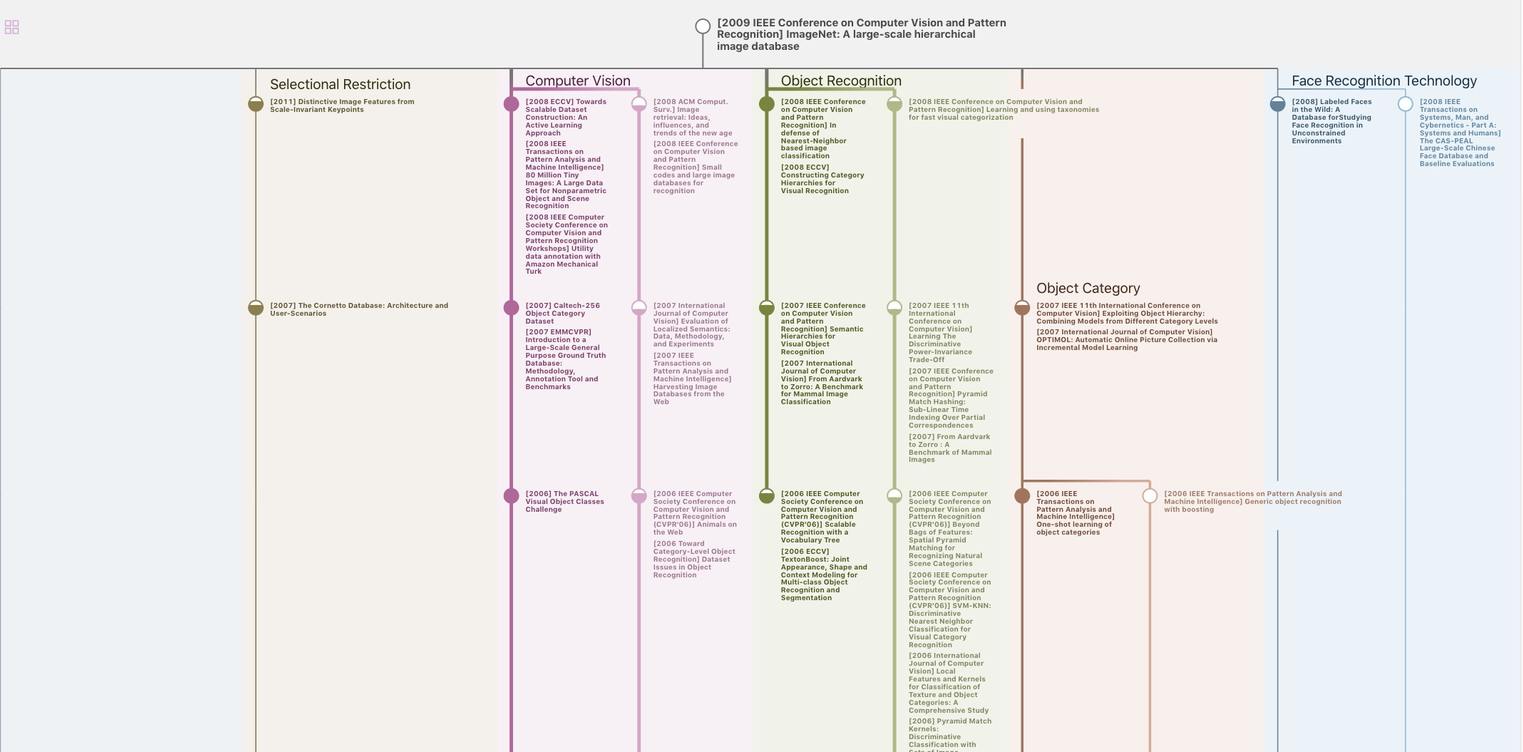
生成溯源树,研究论文发展脉络
Chat Paper
正在生成论文摘要