Temporal Dynamic Synchronous Functional Brain Network for Schizophrenia Diagnosis and Lateralization Analysis
CoRR(2023)
摘要
Available evidence suggests that dynamic functional connectivity (dFC) can capture time-varying abnormalities in brain activity in rs-fMRI data and has a natural advantage in uncovering mechanisms of abnormal brain activity in schizophrenia(SZ) patients. Hence, an advanced dynamic brain network analysis model called the temporal brain category graph convolutional network (temporal-BCGCN) was employed. Firstly, a unique dynamic brain network analysis module, DSF-BrainNet, was designed to construct dynamic synchronization features. Subsequently, a revolutionary graph convolution method, TemporalConv, was proposed, based on the synchronous temporal properties of feature. Finally, the first modular abnormal hemispherical lateralization test tool in deep learning based on rs-fMRI data, named CategoryPool, was proposed. This study was validated on COBRE and UCLA datasets and achieved 83.62% and 89.71% average accuracy, respectively, outperforming the baseline model and other State-of-the-Art methods. The ablation results also demonstrate the advantages of TemporalConv over the traditional edge feature convolution approach of graph convolutional neural network (GCN) and the improvement of CategoryPool over the classical graph pooling approach. Interestingly, this study showed that the lower order perceptual system and higher order network regions in the left hemisphere are more severely dysfunctional than in the right hemisphere of SZ, and reaffirms the importance of the left medial superior frontal gyrus in SZ. Our core code is available at: https://github.com/swfen/Temporal-BCGCN.
更多查看译文
AI 理解论文
溯源树
样例
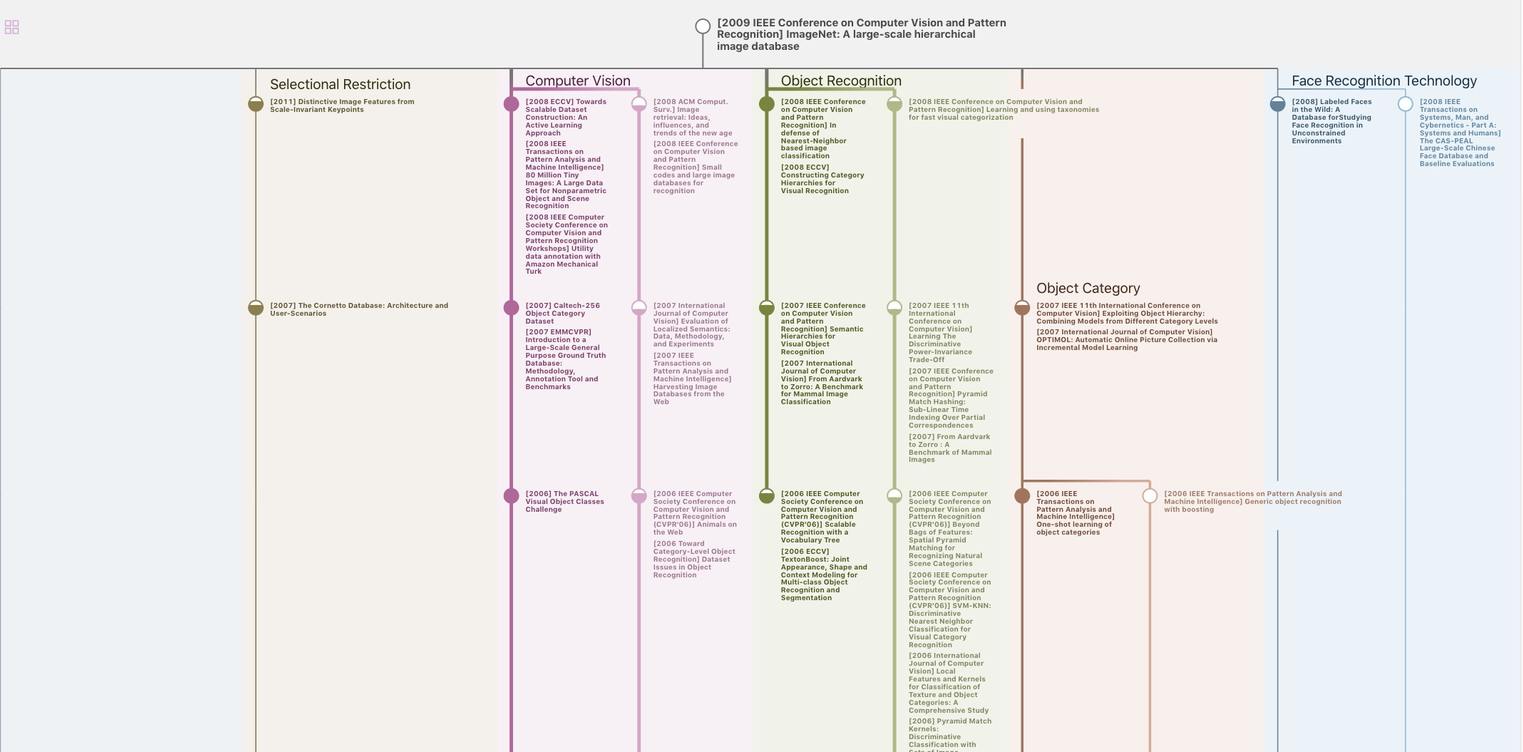
生成溯源树,研究论文发展脉络
Chat Paper
正在生成论文摘要