Moving Obstacle Collision Avoidance via Chance-Constrained MPC with CBF
CoRR(2023)
摘要
Model predictive control (MPC) with control barrier functions (CBF) is a promising solution to address the moving obstacle collision avoidance (MOCA) problem. Unlike MPC with distance constraints (MPC-DC), this approach facilitates early obstacle avoidance without the need to increase prediction horizons. However, the existing MPC-CBF method is deterministic and fails to account for perception uncertainties. This paper proposes a generalized MPC-CBF approach for stochastic scenarios, which maintains the advantages of the deterministic method for addressing the MOCA problem. Specifically, the chance-constrained MPC-CBF (CC-MPC-CBF) technique is introduced to ensure that a user-defined collision avoidance probability is met by utilizing probabilistic CBFs. However, due to the potential empty intersection between the reachable set and the safe region confined by CBF constraints, the CC-MPC-CBF problem can pose challenges in achieving feasibility. To address this issue, we propose a sequential implementation approach that involves solving a standard MPC optimization problem followed by a predictive safety filter optimization, which leads to improved feasibility. Furthermore, we introduce an iterative convex optimization scheme to further expedite the resolution of the predictive safety filter, which results in an efficient approach to tackling the non-convex CC-MPC-CBF problem. We apply our proposed algorithm to a 2-D integrator system for MOCA, and we showcase its resilience to obstacle measurement uncertainties and favorable feasibility properties.
更多查看译文
关键词
obstacle collision avoidance,cbf,chance-constrained
AI 理解论文
溯源树
样例
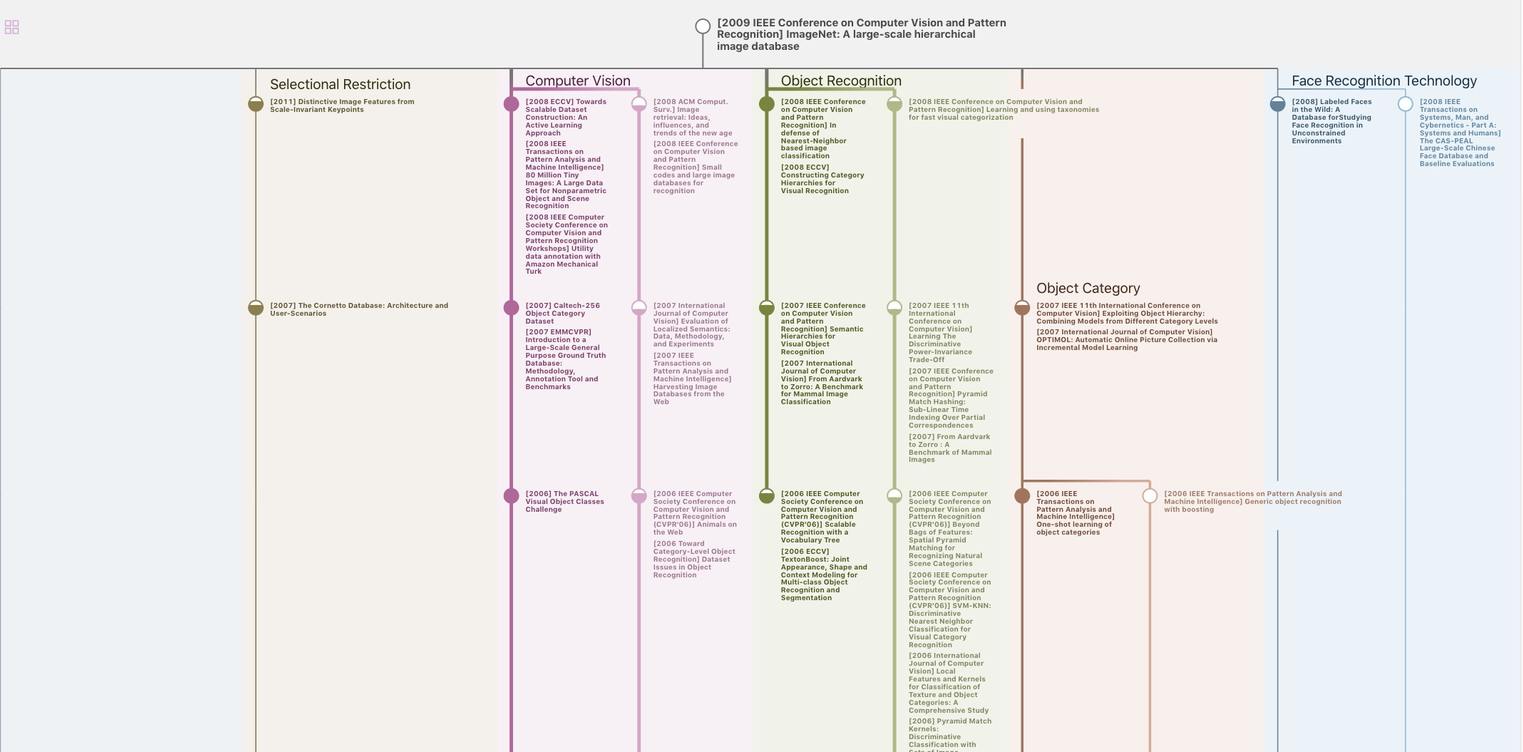
生成溯源树,研究论文发展脉络
Chat Paper
正在生成论文摘要