Density-adaptive registration of pointclouds based on Dirichlet Process Gaussian Mixture Models
PHYSICAL AND ENGINEERING SCIENCES IN MEDICINE(2023)
摘要
We propose an algorithm for rigid registration of pre- and intra-operative patient anatomy, represented as pointclouds, during minimally invasive surgery. This capability is essential for development of augmented reality systems for guiding such interventions. Key challenges in this context are differences in the point density in the pre- and intra-operative pointclouds, and potentially low spatial overlap between the two. Solutions, correspondingly, must be robust to both of these phenomena. We formulated a pointclouds registration approach which considers the pointclouds after rigid transformation to be observations of a global non-parametric probabilistic model named Dirichlet Process Gaussian Mixture Model. The registration problem is solved by minimizing the Kullback–Leibler divergence in a variational Bayesian inference framework. By this means, all unknown parameters are recursively inferred, including, importantly, the optimal number of mixture model components, which ensures the model complexity efficiently matches that of the observed data. By presenting the pointclouds as KDTrees, both the data and model are expanded in a coarse-to-fine style. The scanning weight of each point is estimated by its neighborhood, imparting the algorithm with robustness to point density variations. Experiments on several datasets with different levels of noise, outliers and pointcloud overlap show that our method has a comparable accuracy, but higher efficiency than existing Gaussian Mixture Model methods, whose performance is sensitive to the number of model components.
更多查看译文
关键词
Pointclouds registration,Density adaptive,Variational Bayesian inference,Dirichlet Process Gaussian mixture model
AI 理解论文
溯源树
样例
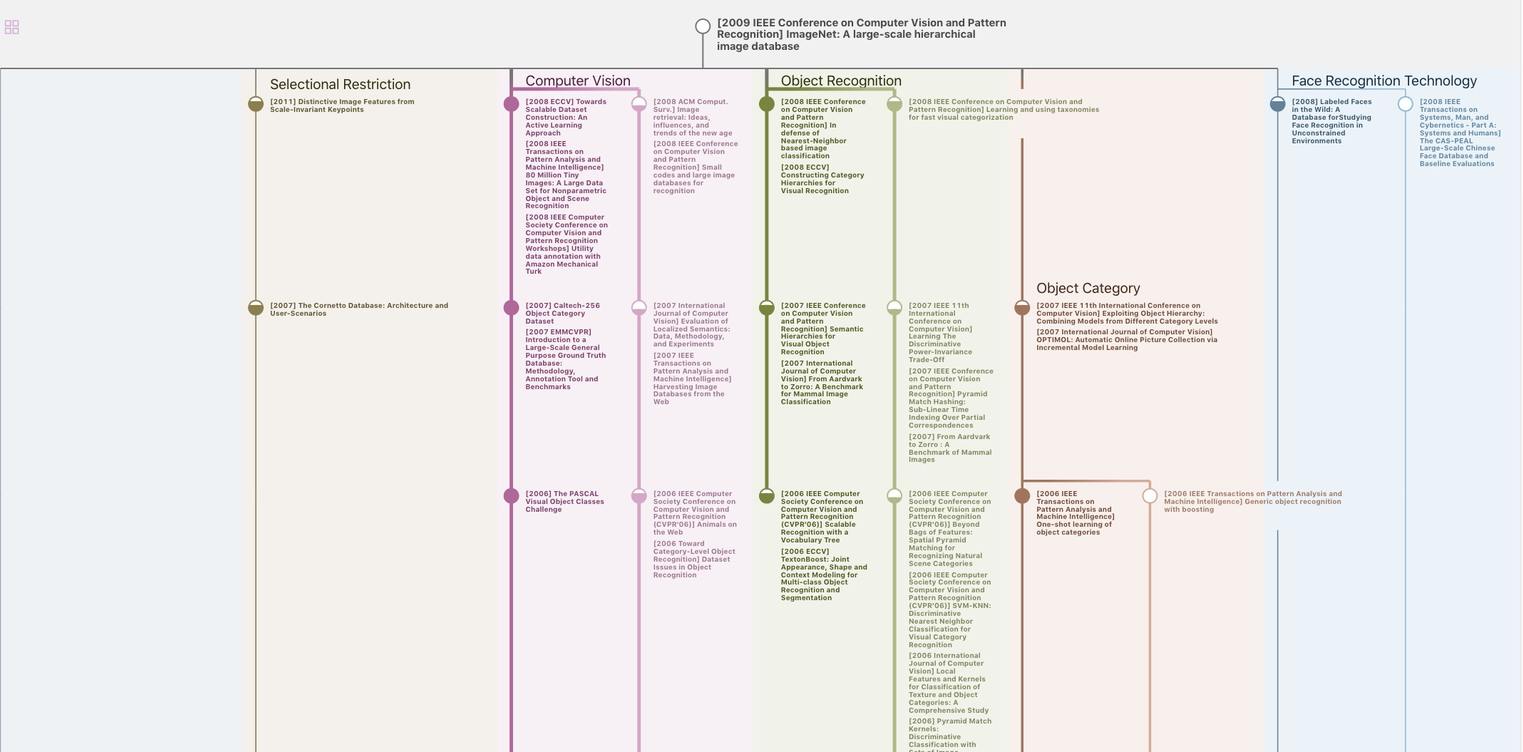
生成溯源树,研究论文发展脉络
Chat Paper
正在生成论文摘要