A hybrid demultiplexing strategy that improves performance and robustness of cell hashing
biorxiv(2023)
摘要
Recent advances in single cell RNA sequencing allow users to pool multiple samples and demultiplex in downstream analysis, which greatly increase experimental efficiency and cost-effectiveness. Among all the demultiplexing methods, nucleotide barcode-based cell hashing has gained widespread popularity due to its compatibility and simplicity. Despite these advantages, certain issues of this technic remain to be solved, such as challenges in distinguishing true positive from background, high reagent cost for samples with large cell numbers, and unpredictable false negative and false doublet rates. Here, we propose a hybrid demultiplexing strategy that increases calling accuracy and cell recovery of cell hashing without adding experimental cost. In this approach, we computationally cluster all single cells based on their natural genetic variations and assign donor identity by finding the dominant hashtag in each genotype cluster. This hybrid strategy assigns donor identity to any cell that is identified as singlet by either genotype clustering or cell hashing, which allows us to demultiplex most majority of cells even if only a small fraction of cells are labeled with hashtags. When comparing its performance with cell hashing on multiple real-world datasets, this hybrid approach consistently generates reliable demultiplexing results with increased cell recovery and accuracy.
Key Points
1. The improved cut-off calling tool, HTOreader, accurately distinguishes true positive from background signal for each individual hashtag.
2. The hybrid demultiplexing strategy increases cell recovery of cell hashing by increasing cut-off calling accuracy and decreasing false negative and false double rates.
3. The hybrid strategy enhances cost-effectiveness of cell hashing and consistently produces reliable demultiplexing results, regardless of hashtag staining quality.
4. The hybrid strategy can be seamlessly integrated into a variety of single-cell experimental protocols and analytic pipelines.
### Competing Interest Statement
The authors have declared no competing interest.
更多查看译文
AI 理解论文
溯源树
样例
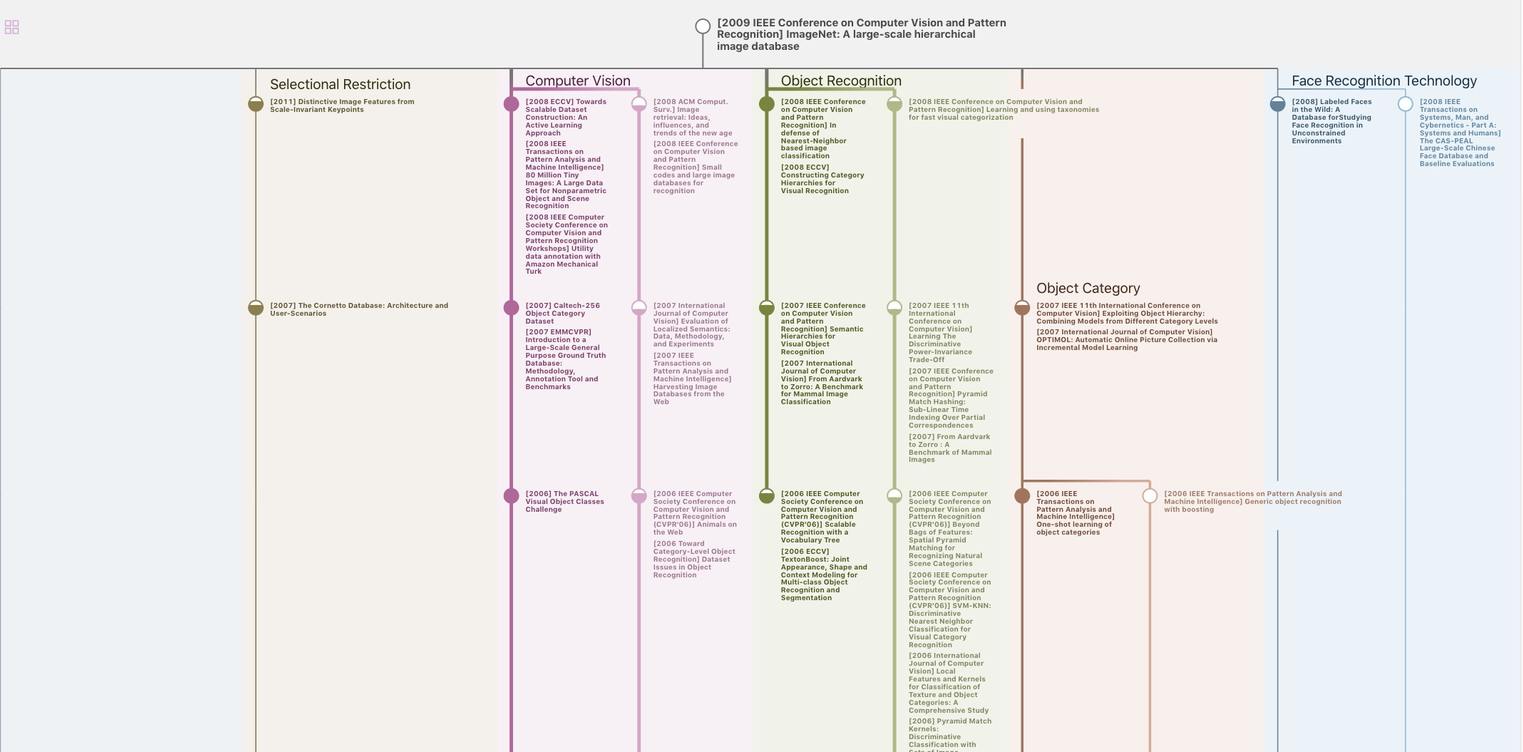
生成溯源树,研究论文发展脉络
Chat Paper
正在生成论文摘要