Correcting Flaws in Common Disentanglement Metrics
CoRR(2023)
摘要
Recent years have seen growing interest in learning disentangled representations, in which distinct features, such as size or shape, are represented by distinct neurons. Quantifying the extent to which a given representation is disentangled is not straightforward; multiple metrics have been proposed. In this paper, we identify two failings of existing metrics, which mean they can assign a high score to a model which is still entangled, and we propose two new metrics, which redress these problems. We then consider the task of compositional generalization. Unlike prior works, we treat this as a classification problem, which allows us to use it to measure the disentanglement ability of the encoder, without depending on the decoder. We show that performance on this task is (a) generally quite poor, (b) correlated with most disentanglement metrics, and (c) most strongly correlated with our newly proposed metrics.
更多查看译文
关键词
metrics,flaws
AI 理解论文
溯源树
样例
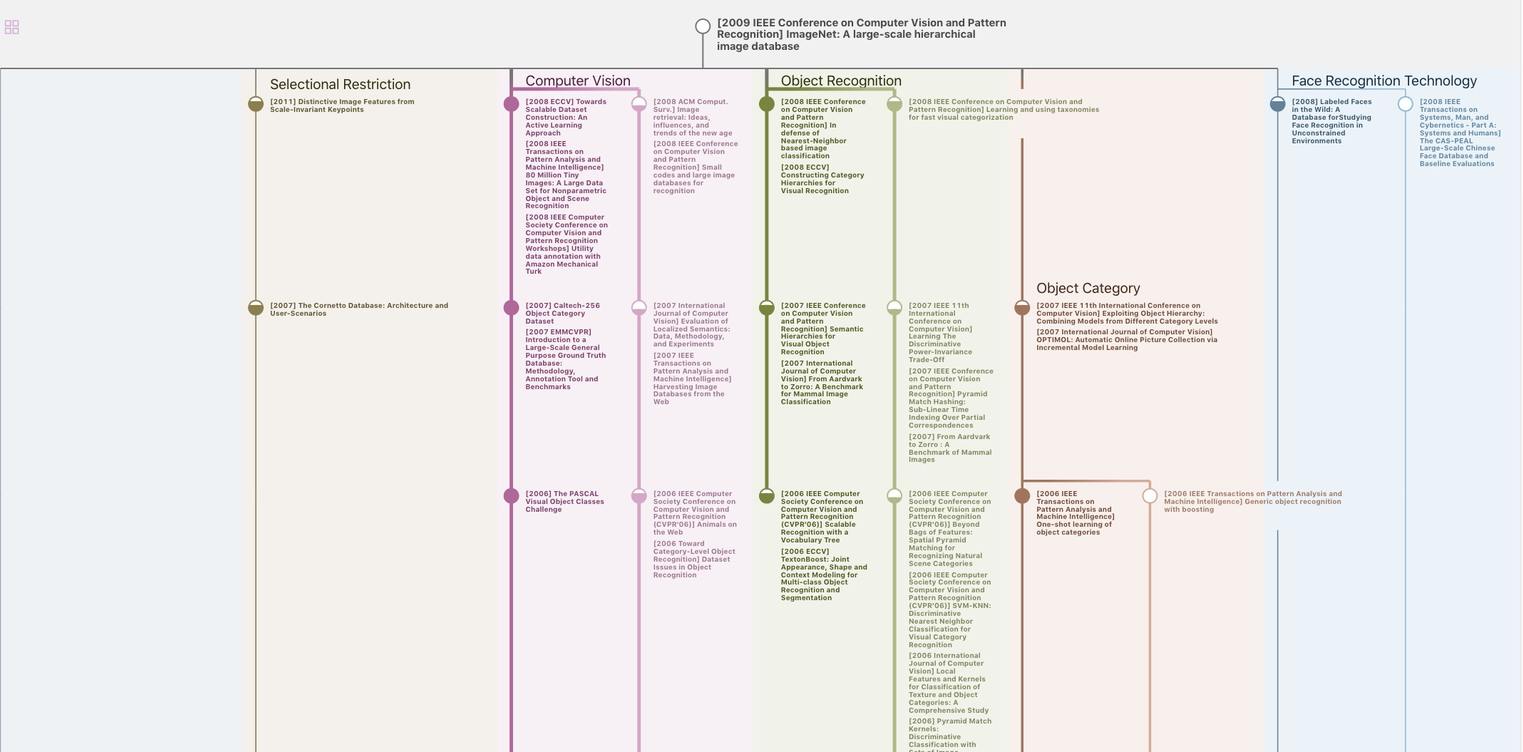
生成溯源树,研究论文发展脉络
Chat Paper
正在生成论文摘要