A parsimonious methodological framework for short-term forecasting of groundwater levels
Science of The Total Environment(2023)
摘要
Groundwater plays a significant role as a strategic resource in reducing the impact of droughts. In spite of its importance, there are still many groundwater bodies in which there is not enough monitoring data to define classic distributed mathematical models to forecast future potential levels. The main aim of this study is to propose and evaluate a novel parsimonious integrated method for the short-term forecasting of groundwater levels. It has low requirements in term of data, and it is operational and relatively easy to apply. It uses geostatistics, optimal meteorological exogenous variables and artificial neural networks. We have illustrated our method in the aquifer "Campo de Montiel" (Spain). The analysis of optimal exogenous variables revealed that, in general, the wells with stronger correlations with precipitation are located closer to the central part of the aquifer. NAR, which does not consider secondary information, is the best approach for 25.5 % of the cases and is associated with well locations with lower R between groundwater levels and precipitation. Amongst the approaches with exogenous variables, the ones that use effective precipitation have been selected more times as the best experiments. NARX and Elman using effective precipitation had the best approaches with 21.6 % and 29.4 % of the cases respectively. For the selected approaches, we obtained a mean RMSE of 1.14 m in the test and 0.76, 0.92, 0.92, 0.87, 0.90, and 1.05 m for the forecasting test for months 1 to 6 respectively for the 51 wells, but the accuracy of the results can vary depending on the well. The interquartile range of the RMSE is around 2 m for the test and forecasting test. The uncertainty of the forecasting is also considered by generating multiple groundwater level series.
更多查看译文
关键词
Artificial neural networks,Effective precipitation,Groundwater levels,Ordinary kriging
AI 理解论文
溯源树
样例
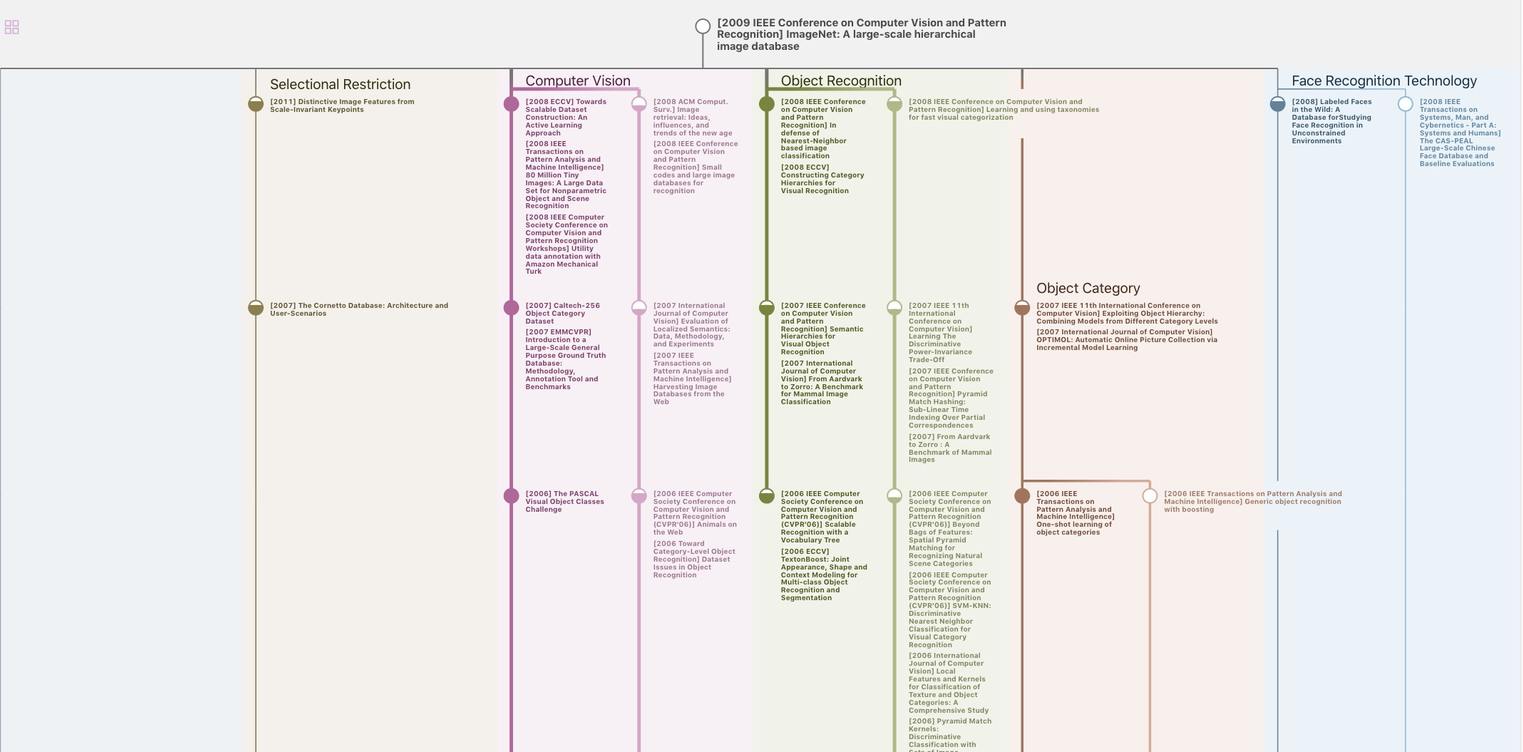
生成溯源树,研究论文发展脉络
Chat Paper
正在生成论文摘要