Detection of Deep Low-Frequency Tremors From Continuous Paper Records at a Station in Southwest Japan About 50 Years Ago Based on Convolutional Neural Network
JOURNAL OF GEOPHYSICAL RESEARCH-SOLID EARTH(2023)
摘要
Since deep low-frequency tremors are considered to be associated with large earthquakes that occur adjacently on the same subducting plate interface, it is important to investigate tremors that occurred before the establishment of modern seismograph networks such as the High Sensitivity Seismograph Network (Hi-net). We propose a deep-learning solution to detect evidence of tremors in scanned images of paper seismogram records from over 50 years ago. In this study, we fine-tuned a convolutional neural network (CNN) based on the Residual Network, which was pre-trained using images of synthetic waveforms from our previous study, using a data set comprised of images generated from real seismic data recorded digitally by Hi-net to facilitate a supervised analysis. The fine-tuned CNN was able to predict the presence or absence of tremors in the Hi-net images with an accuracy of 98.64%. Gradient-weighted Class Activation Mapping heatmaps created to visualize model predictions indicated that the CNN's ability to detect tremors is not degraded by the presence of teleseisms. Once validated using the Hi-net images, the CNN was applied to paper seismograms recorded from 1966 to 1977 at the Kumano observatory in southwest Japan, operated by Earthquake Research Institute, The University of Tokyo. The CNN showed potential for detecting tremors in scanned images of paper seismogram records from the past, facilitating downstream tasks such as the creation of new tremor catalogs. However, further training using an augmented data set to control for variables such as inconsistent plotting pen thickness is required to develop a universally applicable model.
更多查看译文
关键词
deep low-frequency tremor,deep learning,convolutional neural network,Residual Network,image recognition,past seismogram
AI 理解论文
溯源树
样例
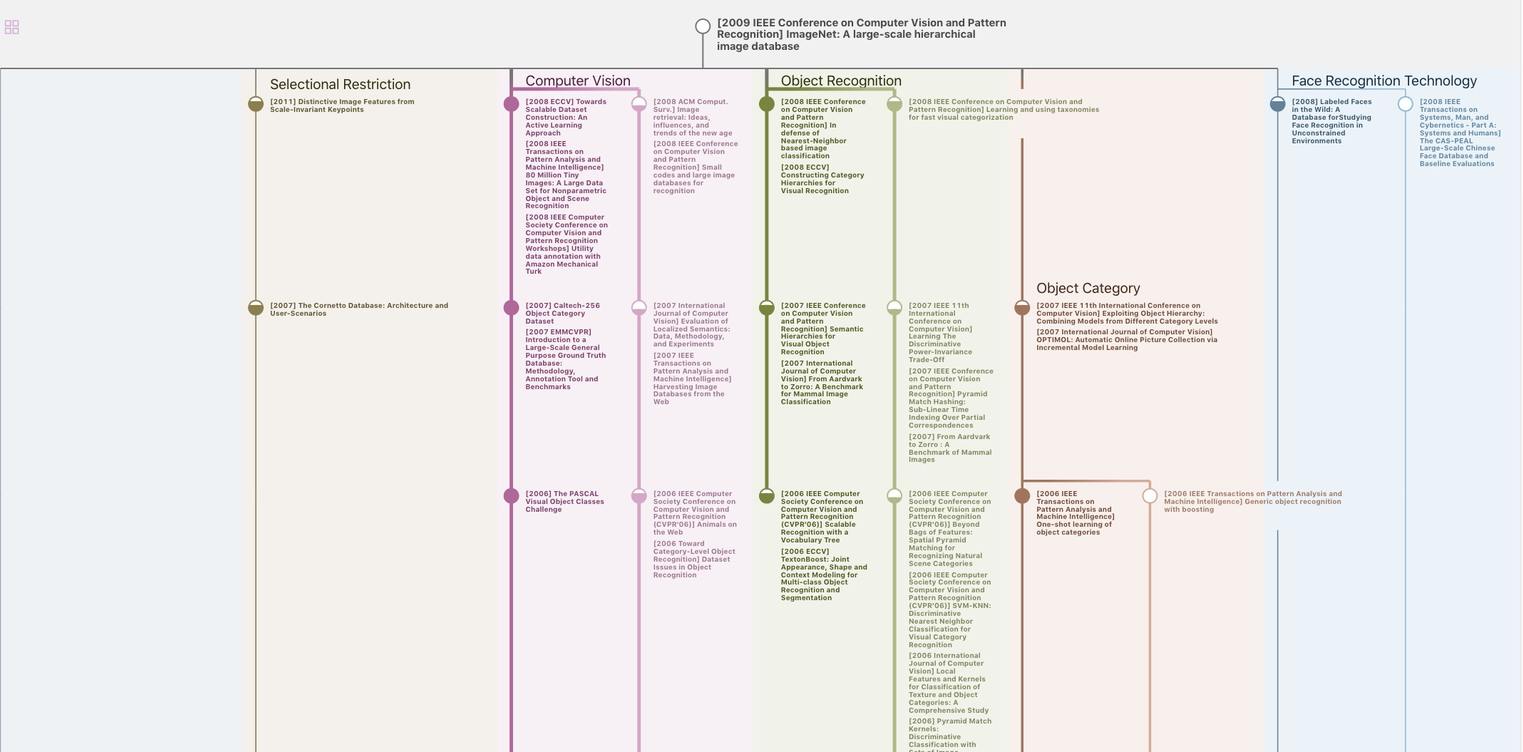
生成溯源树,研究论文发展脉络
Chat Paper
正在生成论文摘要