Identification Method of Microplastics Based on Raman-infrared Spectroscopy Fusion
OPTICAL DESIGN AND TESTING XII(2023)
摘要
The combination of spectroscopic technology and machine learning algorithm for rapid identification of microplastics provides great technical support for the field detection of microplastics, which is a new field that has attracted great attention. Raman spectroscopy can identify organic and inorganic additives and coatings, as well as polymer substrates. However, under the action of fluorescence, additives or pigments affect the Raman spectrum signal. Infrared spectrum detection technology can usually for polymer gives a direct identification of information as a result, the problems existed in the Raman spectroscopy detection technology to evade, but if the detection technology for the additive, especially trace amounts of additives are hard to measure, so the Raman spectroscopy detection technology and infrared spectrum detection technology can complement each other. In this paper, Raman - Infrared spectroscopy fusion detection technology is used to compare the combination of Random Forest (rf),Extreme Gradient Boosting(XGBoost, xgb), and Artificial Neural Network(ANN) three machine learning classification algorithms to build a high-speed and effective recognition and classification model of microplastics. Raman and infrared double-channel microplastic detection system was used to collect the spectral data of 13 common microplastic standard samples. In order to prevent over-fitting, each sample was sampled several times, and a total of 1430 microplastic samples were collected. The 2068 spectral data points of Raman spectral data were compressed to 512 and fused with infrared spectral data. The XGBoost algorithm was used to rank the importance of the fused data, and a total of 69 features which had a great influence on the recognition accuracy were extracted. To eliminate dimensionality, min-max normalization is used to linearly transform the original data, mapping the original data values to between [0-1]. Rf, ANN, and XGBoost algorithm were used to establish the microplastic recognition model for 69 data features extracted after dimensionality reduction, and the confusion matrix and 10-fold cross validation were used to evaluate the model. The results show that the recognition accuracy of single hidden layer ANN model is 97%, that of double hidden layer ANN model is 98%, that of XGBoost model is 97%, and that of random forest model is 99%. The overall performance of random forest model is better than XGBoost model and artificial neural network model.
更多查看译文
关键词
microplastics,Raman infrared spectroscopy fusion,Random forest,Neural network,XGBoost
AI 理解论文
溯源树
样例
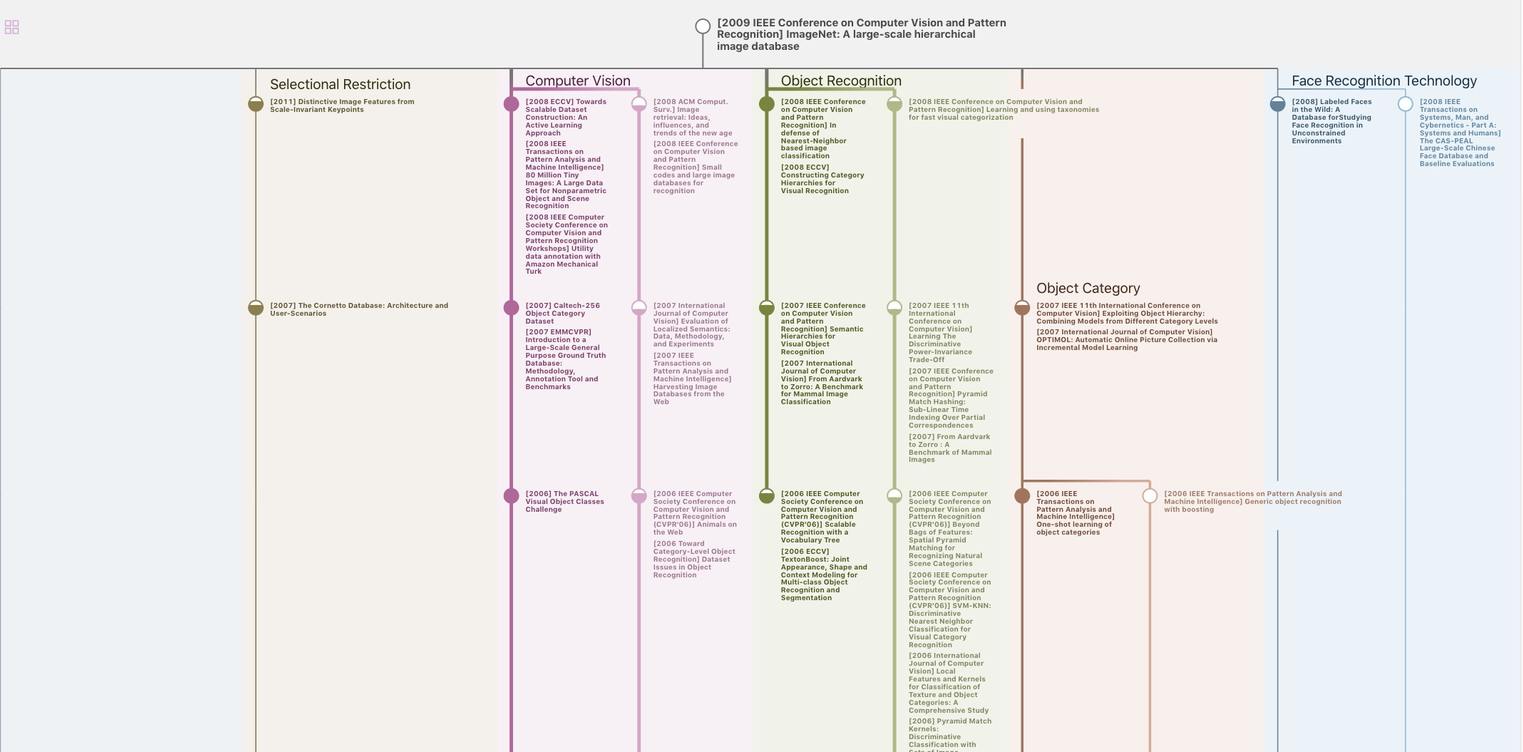
生成溯源树,研究论文发展脉络
Chat Paper
正在生成论文摘要