ETM-face: effective training sample selection and multi-scale feature learning for face detection
MULTIMEDIA TOOLS AND APPLICATIONS(2023)
摘要
In recent years, deep-learning-based face detectors have achieved promising results and been successfully used in a wide range of practical applications. However, extreme appearance variations are still the major obstacles for robust and accurate face detection in the wild. To address this issue, we propose an Improved Training Sample Selection (ITSS) strategy for mining effective positive and negative samples during network training. The proposed ITSS procedure collaborates with face sampling during data augmentation and selects suitable positive sample centres and IoU overlap for face detection. Moreover, we propose a Residual Feature Pyramid Fusion (RFPF) module that collects semantically robust features to improve the scale-invariance of deep features and better represent faces at different feature pyramid levels. The experimental results obtained on the FDDB and WiderFace datasets demonstrate the superiority of the proposed method over the state-of-the-art approaches. Specially, the proposed method achieves 96.9% and 96.2% in terms of AP on the easy and medium test sets of WiderFace.
更多查看译文
关键词
Face detection,Scale variation,Sample selection,Multi-scale feature extraction
AI 理解论文
溯源树
样例
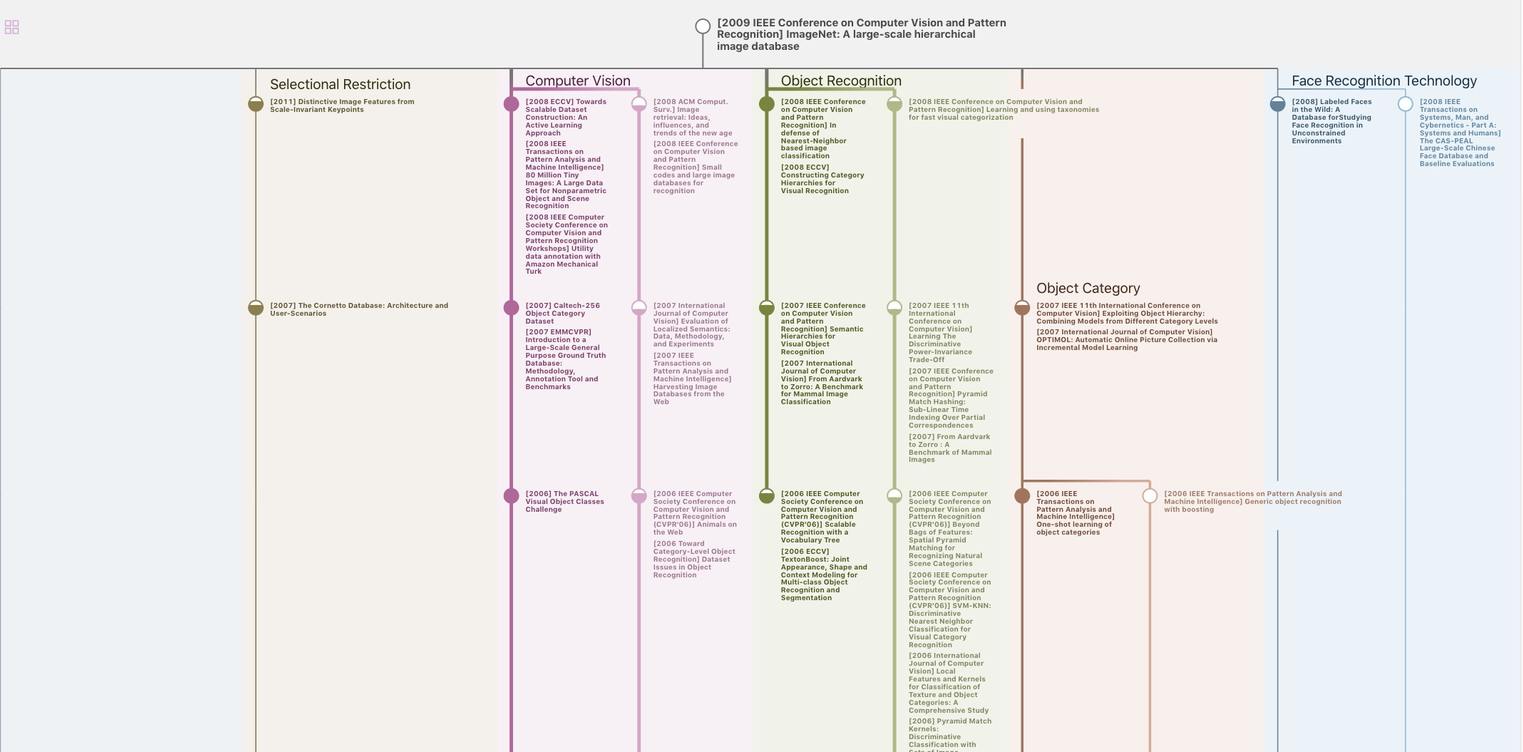
生成溯源树,研究论文发展脉络
Chat Paper
正在生成论文摘要