A novel paradigm for integrating physics-based numerical and machine learning models: A case study of eco-hydrological model
ENVIRONMENTAL MODELLING & SOFTWARE(2023)
摘要
Modeling is an essential tool for studying environmental systems. Due to the interdisciplinary nature, several models should be integrated to consider the nexus between different natural processes. However, the computational cost and accuracy of physics-based numerical models are always limited, even contradictory. In this study, a novel paradigm for integrating numerical models and machine learning models is proposed based on a previous study which integrates WOFOST, HYDRUS and MODFLOW. In the paradigm, the MODFLOW is replaced by Radial Basis Function neural network to reduce the computational costs with comparable accuracy. Object Modeling System is used as modeling framework which provides a run-time environment for the components. A synthetic case is implemented with data collected from Linze Inland River Basin Research Station in northwestern of China. Furthermore, the computation efficiency and generalization ability are discussed by refining the MODFLOW grids and changing the driving forces (precipitation). The proposed paradigm shows significant better performance on flexibility and computational efficiency with similar accuracy and acceptable generalization ability which would be further improved by larger dataset. This paper explores a computational efficiency paradigm by integrating physics-based and machine learning models which also provides a reference for physicsinformed/guided model integration.
更多查看译文
关键词
Model integration,Physics -based model,Machine learning,Eco-hydrological
AI 理解论文
溯源树
样例
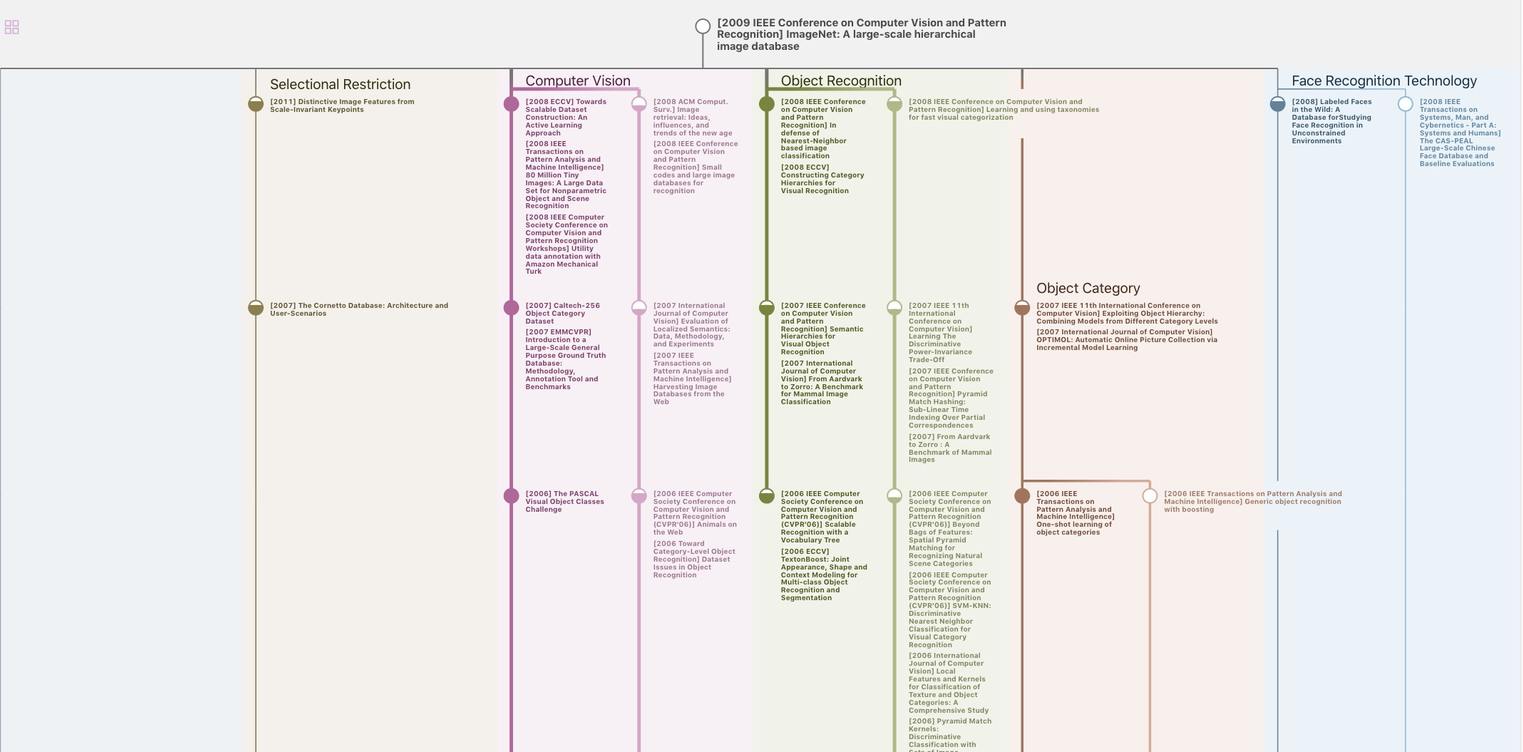
生成溯源树,研究论文发展脉络
Chat Paper
正在生成论文摘要