Ensemble machine learning-based models for estimating the transfer length of strands in PSC beams
EXPERT SYSTEMS WITH APPLICATIONS(2023)
摘要
This study aims to develop four ensemble machine learning (ML) models, including Random Forest (RF), Adaptive Gradient Boosting (AGB), Gradient Boosting (GB), and Extreme Gradient Boosting (XGB), for estimating the transfer length of strands in prestressed concrete (PSC) beams. The results of eleven well-known empirical equations and four single ML models, including Support Vector Machine (SVM), Multi-Layer Perceptron (MLP), K-nearest Neighbors (KNN), and Decision Tree (DT), are used to evaluate the performance of the developed ensemble ML models. This study shows that the GB and XGB models agree well with experimental results and significantly outperform empirical equations and single ML models. The SHapley Additive exPlanations method based on the GB and XGB models determines the compressive strength of concrete at prestress release, initial prestress, strand diameter, concrete cover, beam section width, and beam section height have the most significant effect on the transfer length of strands in PSC beams. Eventually, a web application is built based on the best ML models for practical use. It can predict the transfer length of strands in PSC beams without costly and timeconsuming tests.
更多查看译文
关键词
Ensemble machine learning,Prestressed concrete beam,Transfer length,Web application
AI 理解论文
溯源树
样例
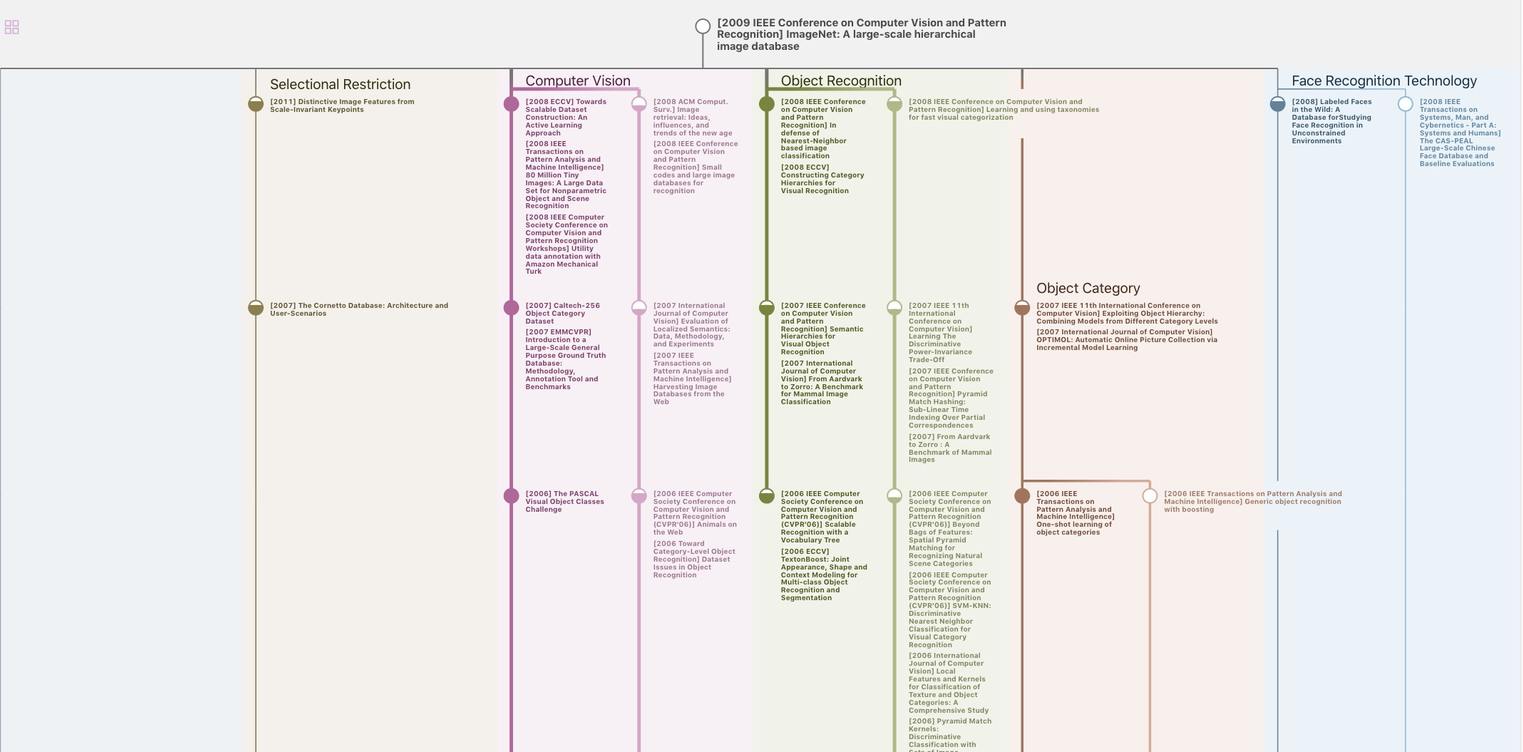
生成溯源树,研究论文发展脉络
Chat Paper
正在生成论文摘要