Product quality prediction in dense medium coal preparation process based on recurrent neural network
INTERNATIONAL JOURNAL OF COAL PREPARATION AND UTILIZATION(2024)
摘要
Dense medium separation is one of the most widely used methods for coal beneficiation, and an accurate estimation of product coal ash is the prerequisites to maximize the resource value. In this work, data pre-cleaning and multi-layer feature learning process of deep learning are explored to predict the product quality more accurately. The research results show that, the deep learning model considering the time series characteristics of process variables can predict the change of product quality better, which could be attributed to the fact that the full considers time series relationship between process data and product quality. Specifically, the prediction value obtained by using a single-layer, multi-unit recurrent neural network model (GRU) is improved with Root Mean Square Error (RMSE) at 0.061, and the RMSE for multi-step prediction (10 min) is 0.262. What's more, the prediction error gradually decreases and tends to be smooth with increasing time step. It is suggested that the time step used for data reconstruction should be the time of raw coal coming through to the clean coal belt. This work provides an innovative idea for improving online prediction of coal ash and is of significant importance for precise control of dense medium separation in application.
更多查看译文
关键词
Process industry,Quality prediction,Dense medium separation,Recurrent neural network,Deep learning
AI 理解论文
溯源树
样例
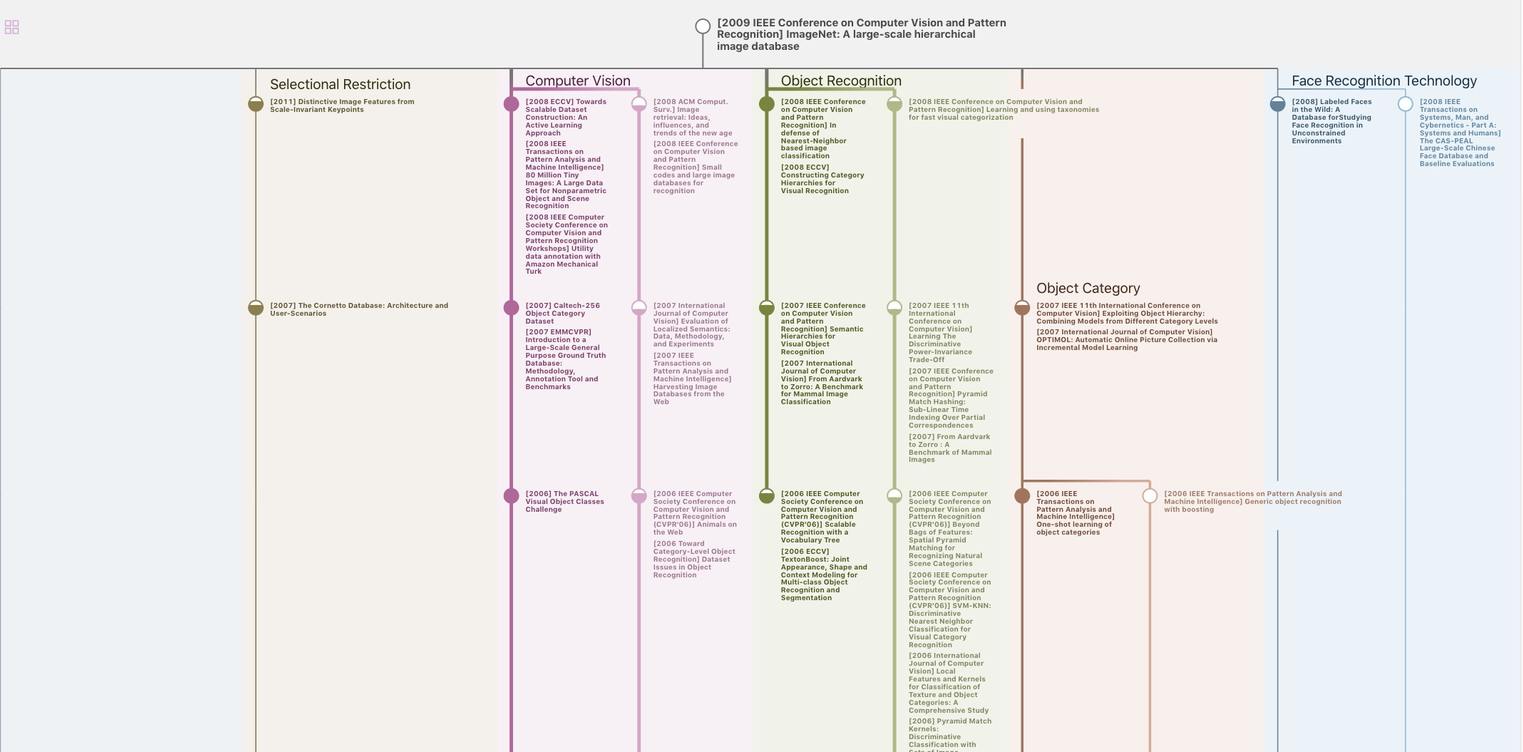
生成溯源树,研究论文发展脉络
Chat Paper
正在生成论文摘要