Learning multiscale pipeline gated fusion for underwater image enhancement
MULTIMEDIA TOOLS AND APPLICATIONS(2023)
摘要
Evidence suggests that vision is among the most critical factors in marine information exploration. Instead, underwater images are generally poor quality due to color casts, lack of texture details, and blurred edges. Therefore, we propose the Multiscale Gated Fusion conditional GAN (MGF-cGAN) for underwater image enhancement. The generator of MGF-cGAN consists of Multiscale Feature Extract Module (Ms-FEM) and Gated Fusion Module (GFM). In Ms-FEM, we use three different parallel subnets to extract feature information, which can extract richer features than a single branch. The GFM can adaptively fuse the three outputs from Ms-FEM. GFM generates better chromaticity and contrast than other fusion ways. Additionally, we add the Multiscale Structural Similarity Index Measure (MS-SSIM) loss to train the network, which is highly similar to human perception. Extensive experiments across three benchmark underwater image datasets corroborate that MGF-cGAN can generate images with better visual perception than classical and State-Of-The-Art (SOTA) methods. It achieves 27.1078dB PSNR and 11.9437 RMSE on EUVP dataset. More significantly, enhanced results of MGF-cGAN also provide excellent performance in underwear saliency detection, SURF key matching test, and so on. Based on this study, MGF-cGAN is found to be suitable for data preprocessing in an underwater multimedia system.
更多查看译文
关键词
Underwater image enhancement,Multiscale feature extraction,Gated fusion,MS-SSIM loss,Conditional GAN
AI 理解论文
溯源树
样例
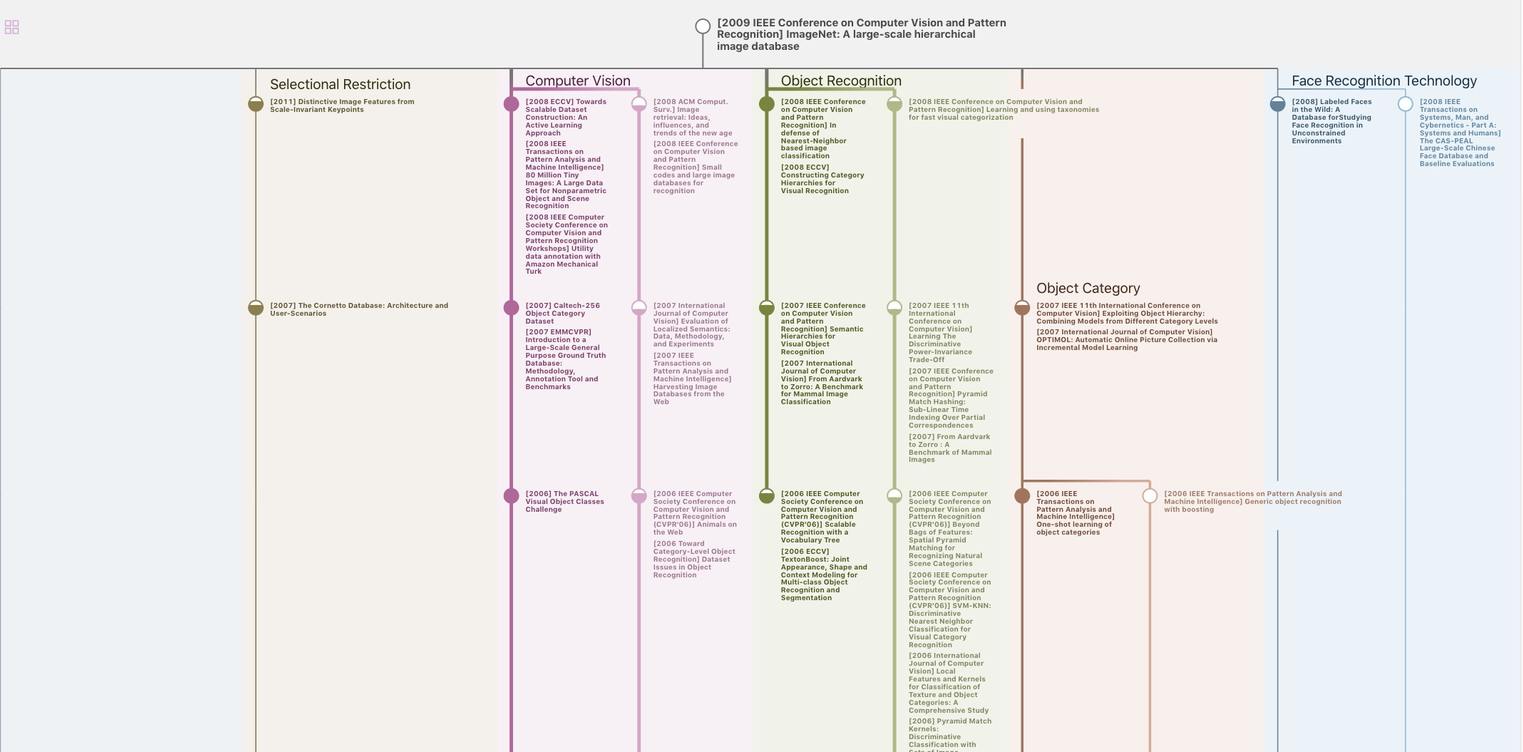
生成溯源树,研究论文发展脉络
Chat Paper
正在生成论文摘要