Addressing the class imbalance problem in network intrusion detection systems using data resampling and deep learning
JOURNAL OF SUPERCOMPUTING(2023)
摘要
Network intrusion detection systems (NIDS) are the most common tool used to detect malicious attacks on a network. They help prevent the ever-increasing different attacks and provide better security for the network. NIDS are classified into signature-based and anomaly-based detection. The most common type of NIDS is the anomaly-based NIDS which is based on machine learning models and is able to detect attacks with high accuracy. However, in recent years, NIDS has achieved even better results in detecting already known and novel attacks with the adoption of deep learning models. Benchmark datasets in intrusion detection try to simulate real-network traffic by including more normal traffic samples than the attack samples. This causes the training data to be imbalanced and causes difficulties in detecting certain types of attacks for the NIDS. In this paper, a data resampling technique is proposed based on Adaptive Synthetic (ADASYN) and Tomek Links algorithms in combination with different deep learning models to mitigate the class imbalance problem. The proposed model is evaluated on the benchmark NSL-KDD dataset using accuracy, precision, recall and F-score metrics. The experimental results show that in binary classification, the proposed method improves the performance of the NIDS and outperforms state-of-the-art models with an achieved accuracy of 99.8%. In multi-class classification, the results were also improved, outperforming state-of-the-art models with an achieved accuracy of 99.98%.
更多查看译文
关键词
Class imbalance,Cybersecurity,Deep convolutional neural networks,Intrusion detection,Long-short-term memory
AI 理解论文
溯源树
样例
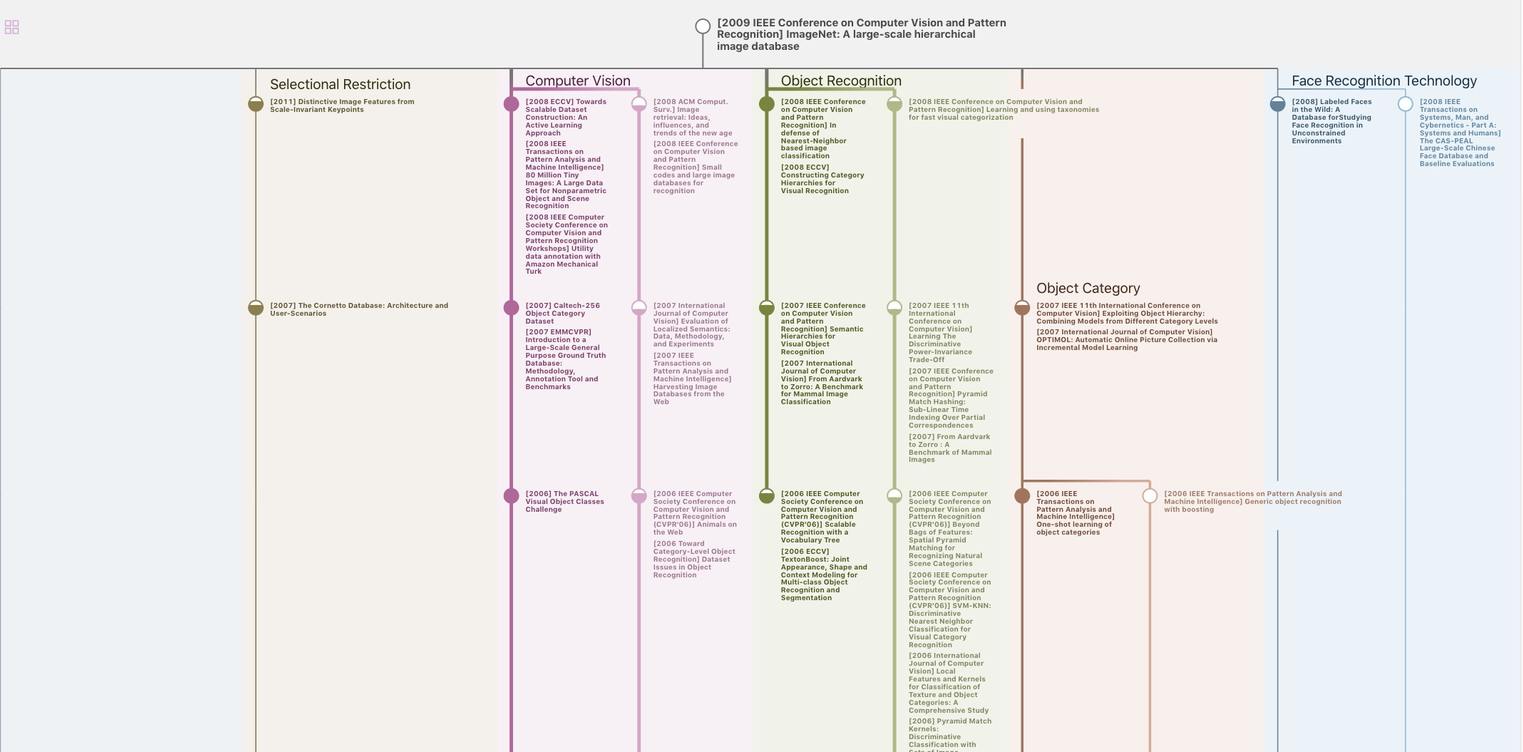
生成溯源树,研究论文发展脉络
Chat Paper
正在生成论文摘要