A two-step anomaly detection based method for PU classification in imbalanced data sets
DATA MINING AND KNOWLEDGE DISCOVERY(2023)
摘要
Several machine learning applications, including genetics and fraud detection, suffer from incomplete label information. In such applications, a classifier can only train from positive and unlabeled (PU) examples in which the unlabeled data consist of both positive and negative examples. Despite a substantial presence of PU learning in the literature, few works have considered a class imbalance setting. Hence, we propose a novel two-step method that exploits anomaly detection to identify hidden positives within the unlabeled data. Our method allows the end-user to choose the anomaly detector depending on preference or domain knowledge. Moreover, we introduce Nearest-Neighbor Isolation Forest (NNIF), a novel semi-supervised anomaly detector based on the Isolation Forest. In contrast to unsupervised anomaly detectors, NNIF can utilize all available label information. Empirical analysis shows that our method generally outperforms, using NNIF as the anomaly detector, state-of-the-art PU learning methods for imbalanced data sets under different labeling mechanisms. Further experiments suggest that our two-step method shows strong robustness to wrong class prior estimates.
更多查看译文
关键词
PU learning,Weak supervision,Anomaly detection,Imbalanced classification
AI 理解论文
溯源树
样例
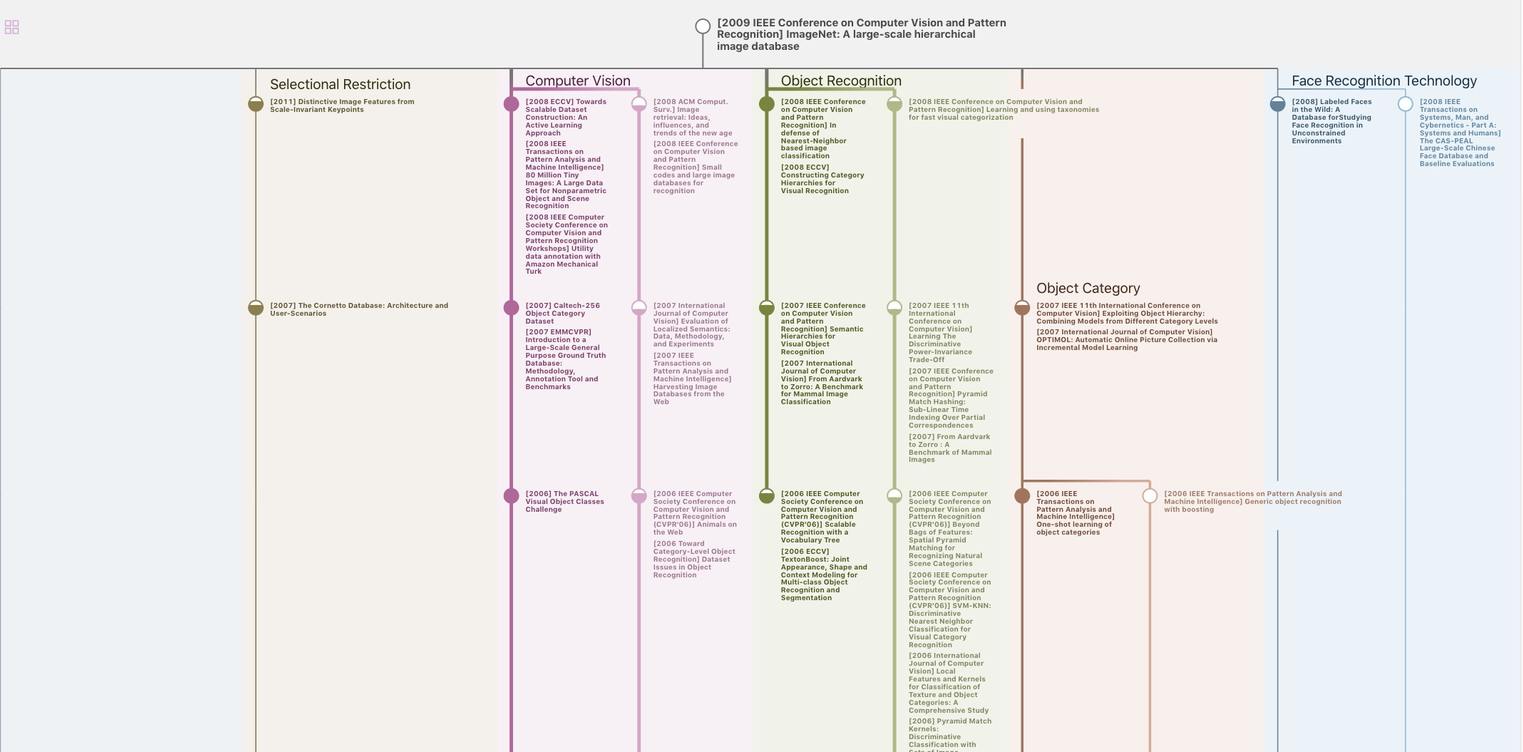
生成溯源树,研究论文发展脉络
Chat Paper
正在生成论文摘要