Spatiotemporal consistent selection-correction network for deep interactive image segmentation
NEURAL COMPUTING & APPLICATIONS(2023)
摘要
Interactive image segmentation can extract specific targets meeting users’ intention, and has received widespread attention in computer vision. The conventional interactive methods rely too much on the user interaction due to the limitation caused by the hand-crafted low-level features. Recently, deep interactive approaches have significantly improved the segmentation performance thanks to the semantic perception ability. However, in these approaches each interaction is generally treated independently by the same way, regardless of its own intention of each click and the potential relationships among the continuous interactions. The above defects still leads them restricted to the conflict between the interaction quantity and the interaction number. To overcome the above problem, this paper focuses on the click-based interactive segmentation task by explicitly mining the intention of each click and linking the relationships among all clicks. A selection-collection training framework is first established to impose the global object selection and the local error correction roles during the whole interaction process. Then a temporal network architecture is designed to continuously connect the entire click sequence. In this case, the respective role of each click can be played as much as possible, and the spatially varying segmentation cues can be propagated in time series. Experiments on the challenging SBD, GrabCut, DAVIS and Berkeley segmentation datasets demonstrate the effectiveness of the proposed method.
更多查看译文
关键词
Interactive segmentation,Deep learning,Iterative training,Spatiotemporal consistency
AI 理解论文
溯源树
样例
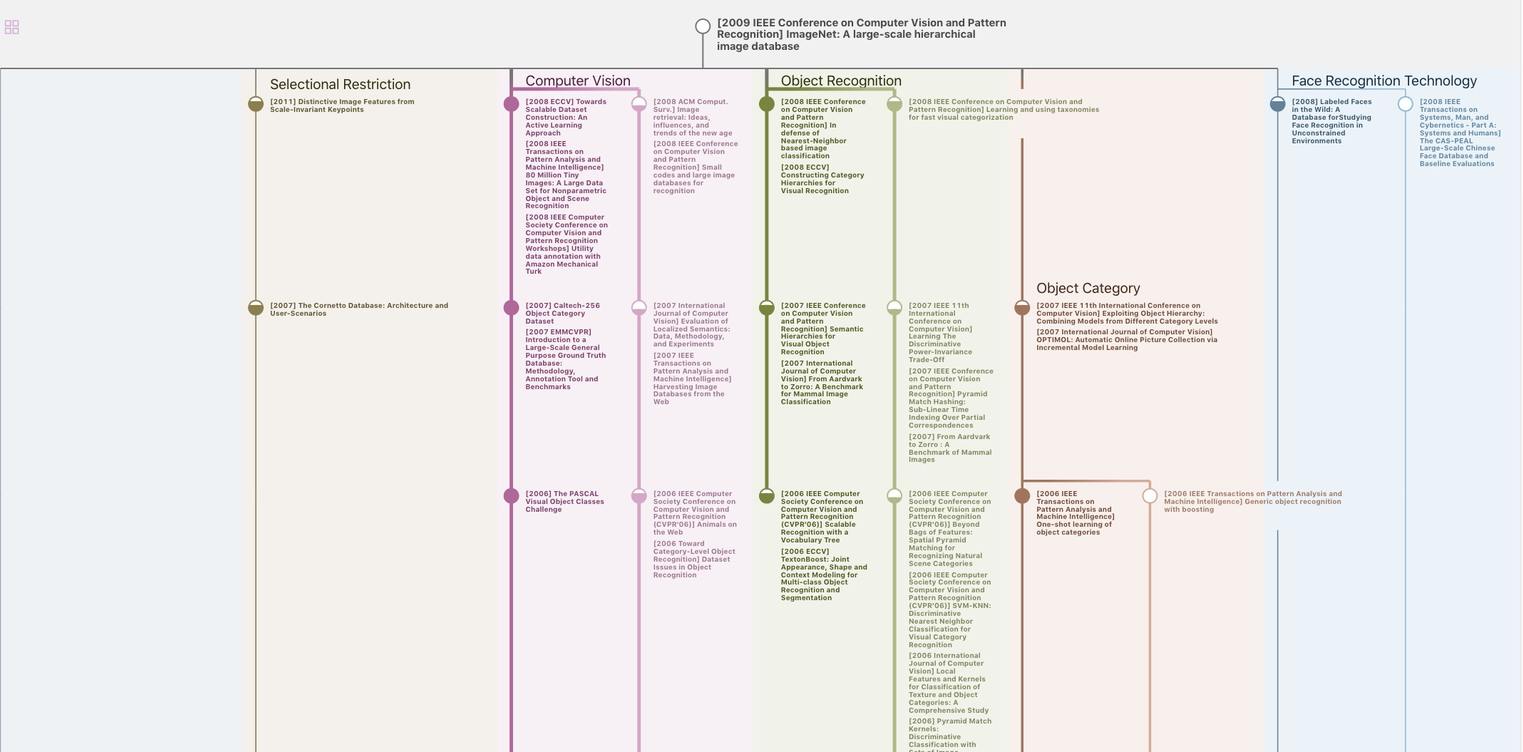
生成溯源树,研究论文发展脉络
Chat Paper
正在生成论文摘要