HMLNet: a hierarchical metric learning network with dual attention for change detection in high-resolution remote sensing images
INTERNATIONAL JOURNAL OF REMOTE SENSING(2023)
摘要
Change detection (CD) in high-resolution remote sensing images (RSIs) can be regarded as a binary visual recognition problem. Metric learning (ML) is a reliable method to determine pixel class attributes based on discriminative distance function between learnable image features. However, most related works learn discriminative distance functions in single-scale feature pairs, which suffer from slow convergence and poor local optima, partially due to that the loss function employs only large-scale feature samples while not interacting with the other scale features. Furthermore, more effective features are a prerequisite for improving the performance of ML-based CD. Hence, we propose a novel hierarchical metric learning network (HMLNet) with dual attention for CD in RSIs, where the key point is that hierarchical metric learning is performed in an ensemble manner to improve detection accuracy and accelerate model convergence. Specifically, based on the features extracted from the encoder-decoder backbone, we construct a feature pyramid to handle the complex details of objects at various scales in RSIs, and then perform metric learning between the paired pyramid features at the same scale. In addition, the dual-attention module is proposed to enhance the internal consistency of changed objects and effectively obtain more detailed information by acting on multi-scale pyramid features. Extensive experiments on the two public RSIs CD datasets, and the results demonstrate that the proposed HMLNet can accurately locate changed objects, which consistently outperforms the state-of-the-art CD competitors.
更多查看译文
关键词
Hierarchical metric learning,dual attention,high-resolution remote-sensing images,change detection
AI 理解论文
溯源树
样例
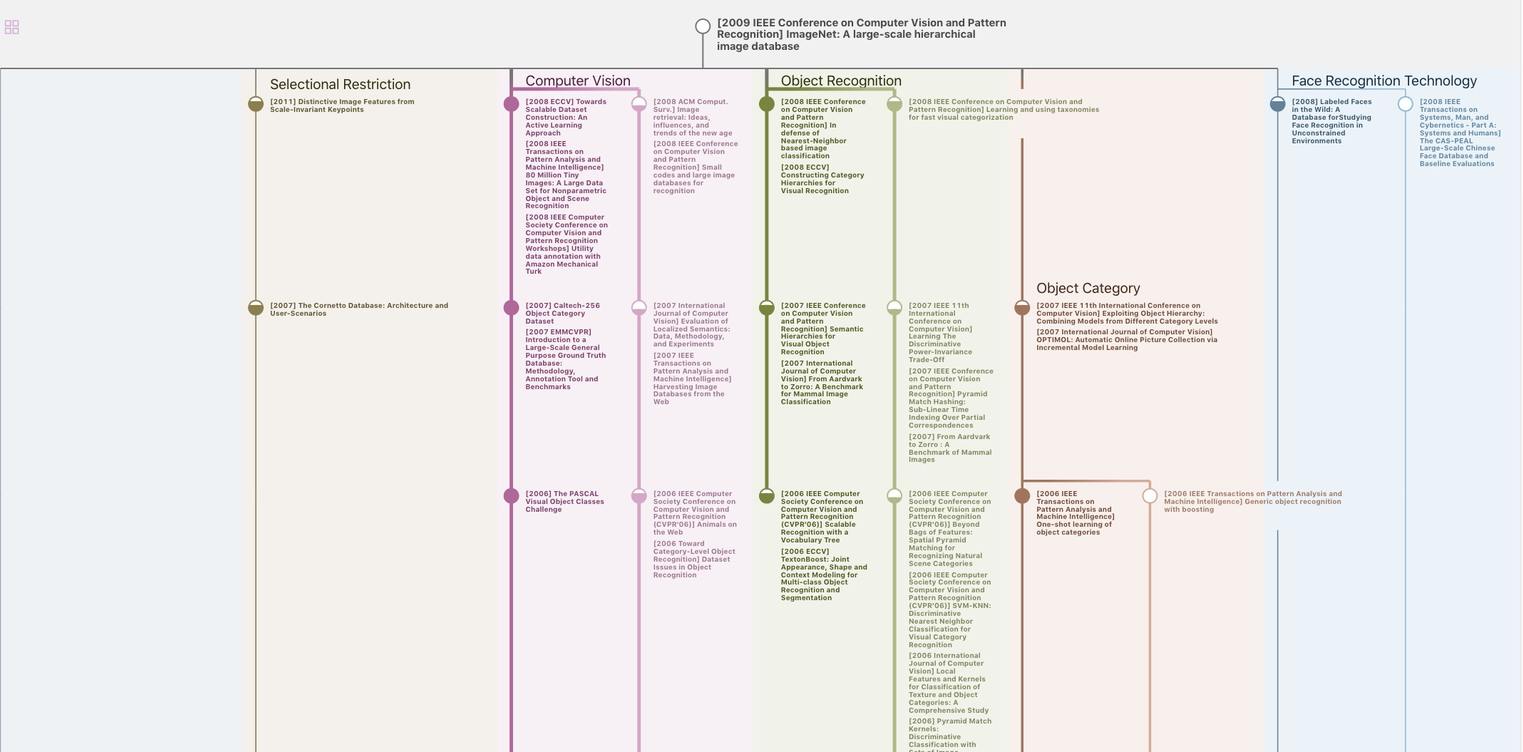
生成溯源树,研究论文发展脉络
Chat Paper
正在生成论文摘要