An importance sampling method for structural reliability analysis based on interpretable deep generative network
Engineering with Computers(2024)
摘要
Importance sampling methods are widely used in structural reliability analysis. However, owing to the complex shape of optimal importance sampling densities, it is usually difficult to fit the optimal importance sampling densities and sample from the fitted distributions using conventional importance sampling methods. In this paper, a novel importance sampling method based on interpretable deep generative network (IDGN-IS) is proposed for structural reliability analysis. The proposed IDGN-IS model can be directly trained using the data from original distribution of random variables and efficiently sampling from an arbitrary importance sampling density. The developed interpretable deep generative network consists of a deep generative network and a monotonic network, which enables the network to fit and sample from the target distributions while being interpretable. Using the interpretability of the deep generative network, the IDGN-IS method can sample from an arbitrary conditional probability distribution of the fitted distributions by choosing an appropriate threshold of the input Gaussian distribution samples. When the threshold of the input Gaussian distribution samples is set to a value close to zero, the IDGN-IS method can efficiently sample from the optimal importance sampling density and provide accurate estimation of the failure probability. The calculation efficiency and estimation accuracy of the proposed IDGN-IS method in structural reliability analysis are demonstrated using four examples.
更多查看译文
关键词
Structural reliability analysis,Importance sampling,Interpretable deep generative network,Flow-based generative model,Monotonic network
AI 理解论文
溯源树
样例
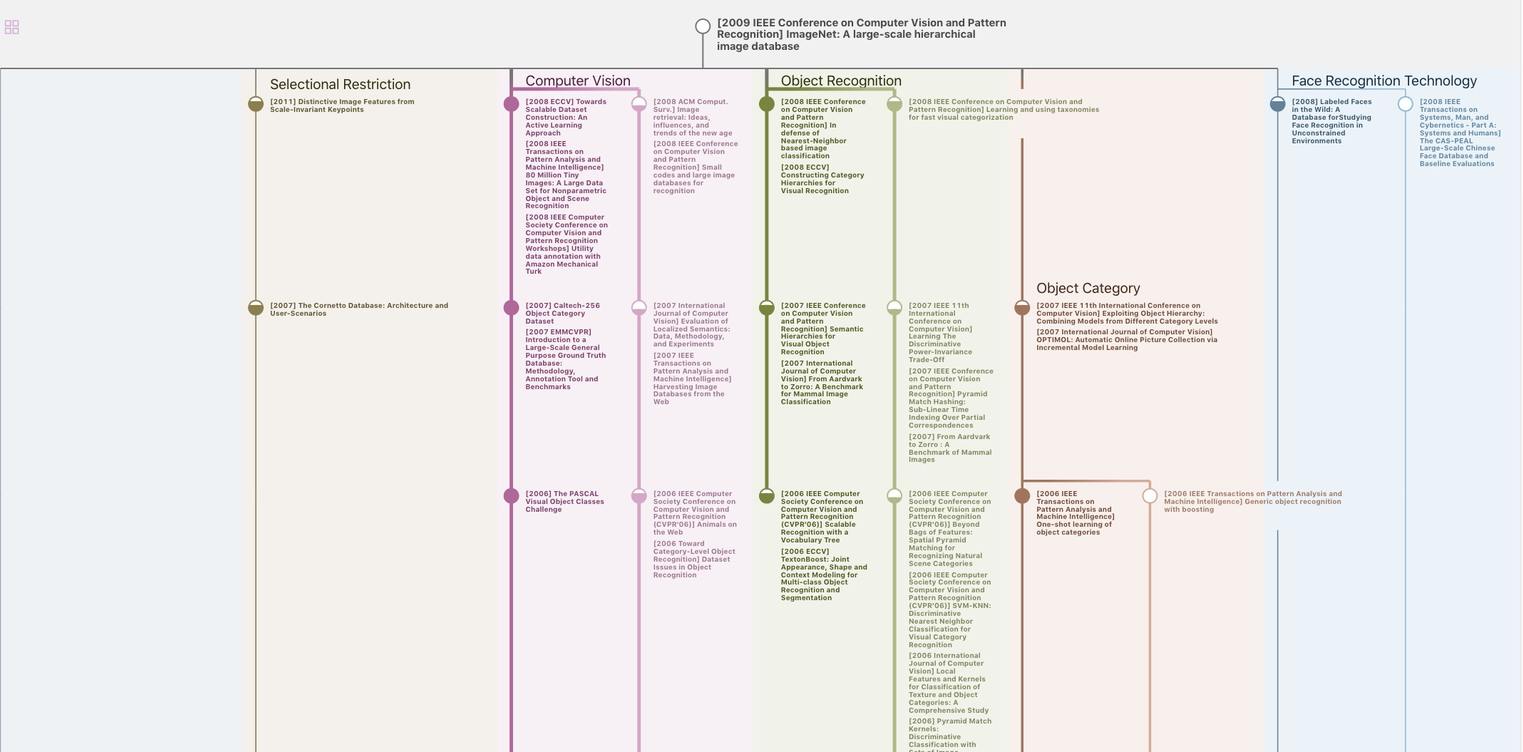
生成溯源树,研究论文发展脉络
Chat Paper
正在生成论文摘要