Dictionary reduction in sparse representation-based classification of motor imagery EEG signals
MULTIMEDIA TOOLS AND APPLICATIONS(2023)
摘要
Recently, sparse representation-based classification has turned into a successful technique for motor imagery electroencephalogram signal analysis. In this approach, the data is sparsely represented using a pre-defined or learned dictionary and classified based on the residual error. Recent works have proved that learned dictionary performs significantly better than the fixed dictionary. But in dictionary learning approach, when the number of training trials increases, the dictionary size increases and hence calculating sparse representation takes longer time and affects the performance accuracy. Thus, a compact dictionary should be considered to reduce the computation time without compromising the accuracy. However, building a compact dictionary is a non-trivial task, as it depends on the size of training data, the number of motor imageries and the discriminative power of features. In this work, two dictionary reduction strategies, namely redundancy identification and dictionary learning have been investigated to build a compact dictionary. Under the redundancy identification strategy, two methods based on distance measure and correlation analysis have been considered. For dictionary learning, discriminative K-SVD (D-KSVD) and label consistent K-SVD (LC-KSVD) have been explored. Extensive experiments show that the LC-KSVD dictionary learning approach produces a better compact dictionary, which takes lower computation time as well as improved accuracy. Further, the results of reduced dictionary with LC-KSVD is comparable to the existing works on sparsity-based motor imagery electroencephalogram signals classification.
更多查看译文
关键词
Brain computer interface,Electroencephalogram signal analysis,Motor imagery brain signal,Sparsity-based classification,Dictionary reduction,Dictionary learning
AI 理解论文
溯源树
样例
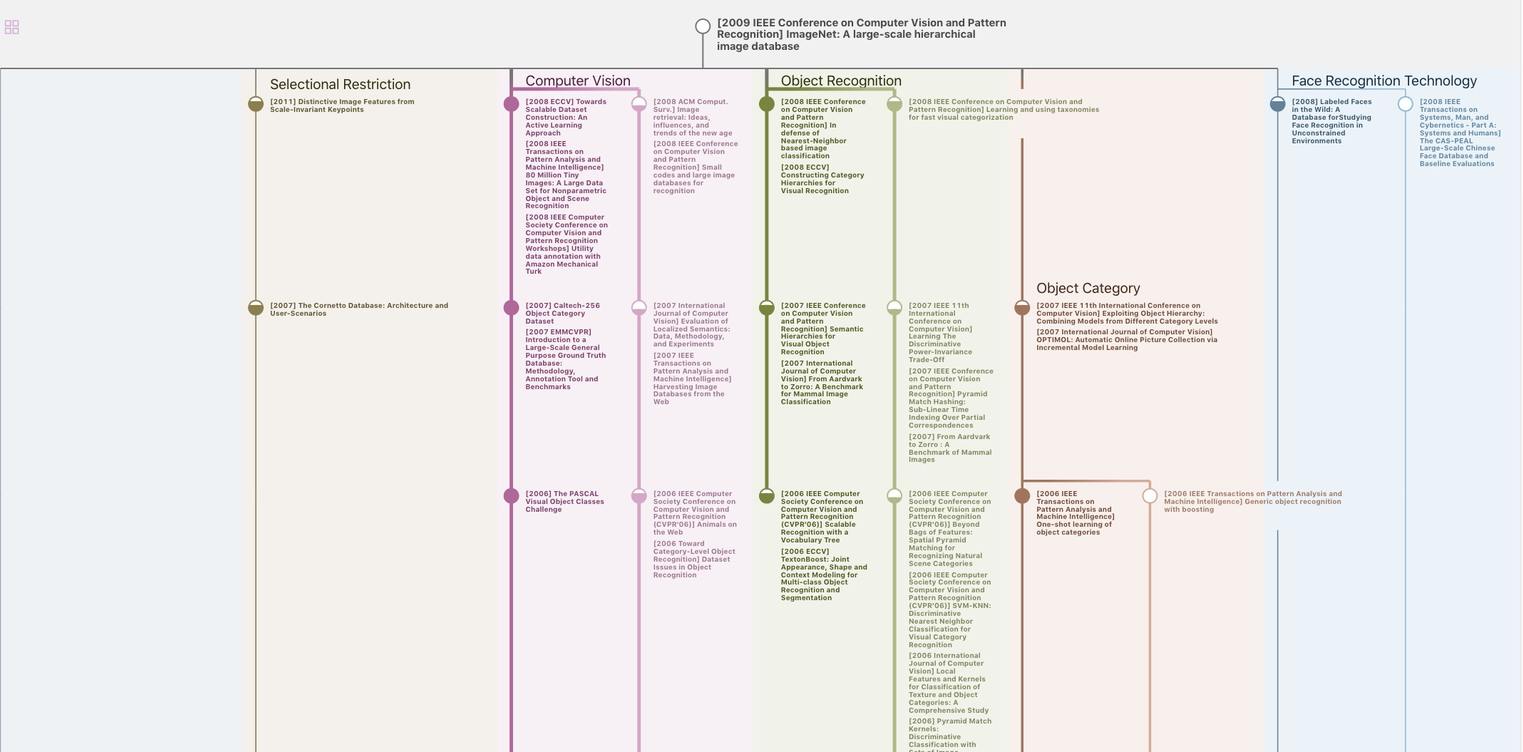
生成溯源树,研究论文发展脉络
Chat Paper
正在生成论文摘要