An interactive deep model combined with Retinex for low-light visible and infrared image fusion
NEURAL COMPUTING & APPLICATIONS(2023)
摘要
Directly fusing the low-light visible and infrared images is hard to obtain fusion results containing rich structural details and critical infrared targets, due to the limitations of extreme illumination. The generated fusion results are typically not accurate to describe the scene nor are suitable for machine perception. Consequently, a novel image fusion framework combined with Retinex theory, termed as LLVIFusion, is designed for the low-light visible and infrared image fusion. Specifically, the training of LLVIFusion is accomplished via a two-stage training strategy. First, a new interactive fusion network is trained to generate initial fusion results with more informative features. Within the interactive fusion network, features are continuously reused in the same branch network and feature information from different branches are constantly interacted through the designed fusion blocks, which allows the fusion network to not only avoid losing information but also strengthen the information for subsequent processing. Further, an adaptive weight-based loss function is proposed to guide the fusion network for training. Next, a refinement network incorporating Retinex theory is introduced to optimize the visibility of the initial fusion results for obtaining high visual quality fusion results. In contrast to the 14 state-of-the-art comparison methods, LLVIFusion achieves the best values for all six objective measures on the LLVIP and the MF-Net datasets, while obtaining two best values and two second-best values for the objective measures on the TNO dataset. Such experimental results show that LLVIFusion can successfully perform low-light visible and infrared image fusion and produce good fusion results under normal illumination. The codes of LLVIFusion are accessible on https://github.com/govenda/LLVIFusion .
更多查看译文
关键词
Low-light visible image and infrared image fusion,Retinex,Deep learning,Information interaction
AI 理解论文
溯源树
样例
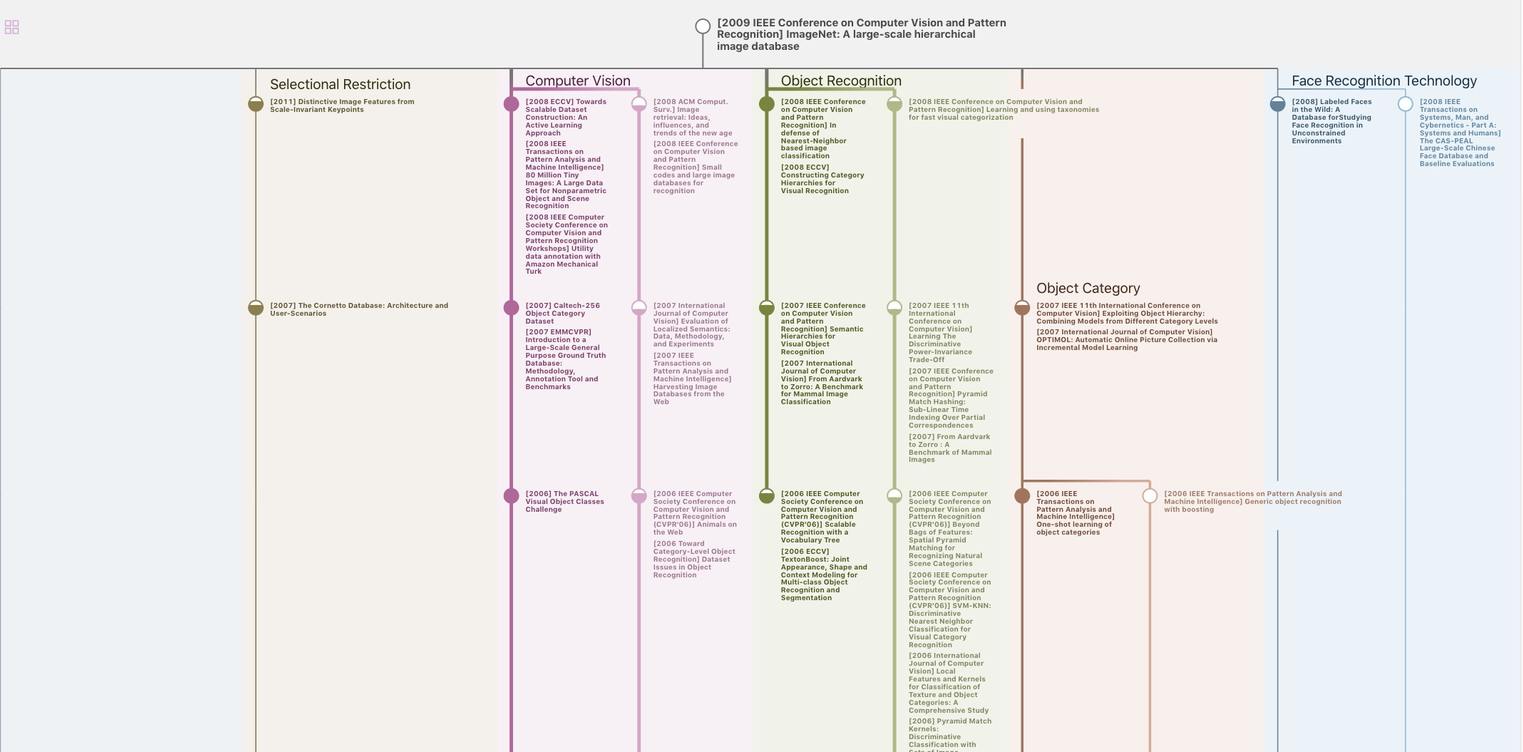
生成溯源树,研究论文发展脉络
Chat Paper
正在生成论文摘要