Self-Sufficient Feature Enhancing Networks for Video Salient Object Detection
IEEE TRANSACTIONS ON MULTIMEDIA(2023)
摘要
Single image deraining (SIDR) often suffers from over/under deraining due to the nonuniformity of rain densities and the variety of raindrop scales. In this paper, we propose a continuous density-guided network (CODE-Net) for SIDR. Particularly, it is composed of a rain streak extractor and a denoiser, where the convolutional sparse coding (CSC) is exploited to filter out noises from the extracted rain streaks. Inspired by the reweighted iterative soft-threshold (ISTA) for CSC, we address the problem of continuous rain density estimation by learning the weights with channel attention blocks from sparse codes. We further develop a multiscale strategy to depict rain streaks appearing at different scales. Experiments on synthetic and real-world data demonstrate the superiority of our methods over recent state-of-the-arts, in terms of both quantitative and qualitative results. Additionally, instead of quantizing rain density with several levels, our CODE-Net can provide continuous-valued estimations of rain densities, which is more desirable in real applications.
更多查看译文
关键词
Rain,Convolutional codes,Estimation,Encoding,Convolutional neural networks,Minimization,Mathematical models,Convolutional sparse coding,image deraining,rain density estimation
AI 理解论文
溯源树
样例
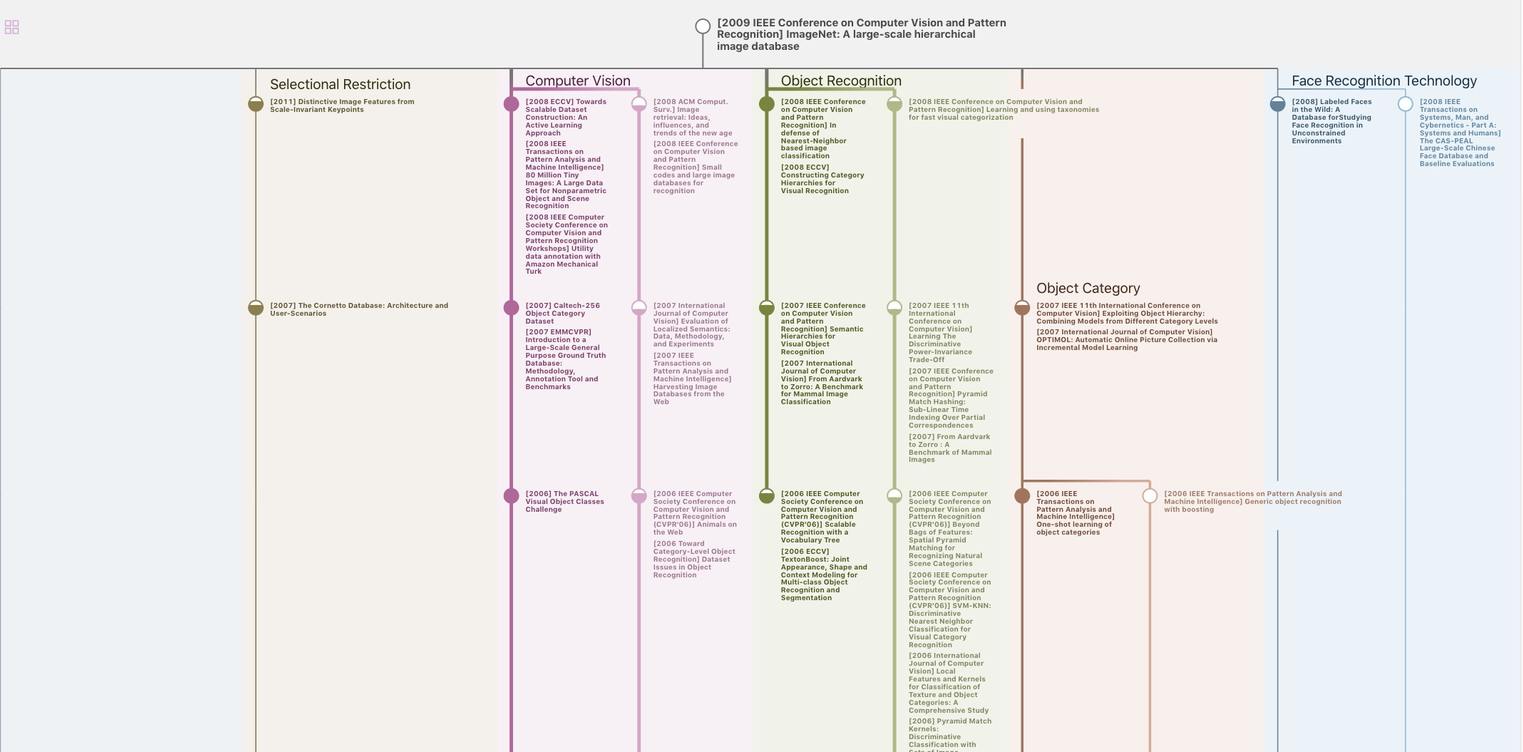
生成溯源树,研究论文发展脉络
Chat Paper
正在生成论文摘要