Decoupling Optimization for Complex PDN Structures Using Deep Reinforcement Learning
IEEE TRANSACTIONS ON MICROWAVE THEORY AND TECHNIQUES(2023)
摘要
This article presents a new optimization method for complex power distribution networks (PDNs) with irregular shapes and multilayer structures using deep reinforcement learning (DRL), which has not been considered before. A fast boundary integration method is applied to compute the impedance matrix of a PDN structure. Subsequently, a new DRL algorithm based on proximal policy optimization (PPO) is proposed to optimize the decoupling capacitor (decap) placement by minimizing the number of decaps while satisfying the desired target impedance. In the proposed approach, the PDN structure information is encoded into matrices and serves as the input of the DRL algorithm, which increases the flexibility of the method to be extended and generalized to different PDN configurations. Also, the output of the algorithm determines the selection of decap types and locations collaboratively, making it easier to find the optimal solution in a huge search space. The proposed method is compared with the state-of-the-art approaches and shows consistent advantages in reducing the number of decaps in different testing cases.
更多查看译文
关键词
Impedance,Optimization,Transmission line matrix methods,Shape,Search methods,Genetic algorithms,Optimization methods,Boundary integration,decoupling capacitor (decap),deep reinforcement learning (DRL),machine learning,power distribution network (PDN)
AI 理解论文
溯源树
样例
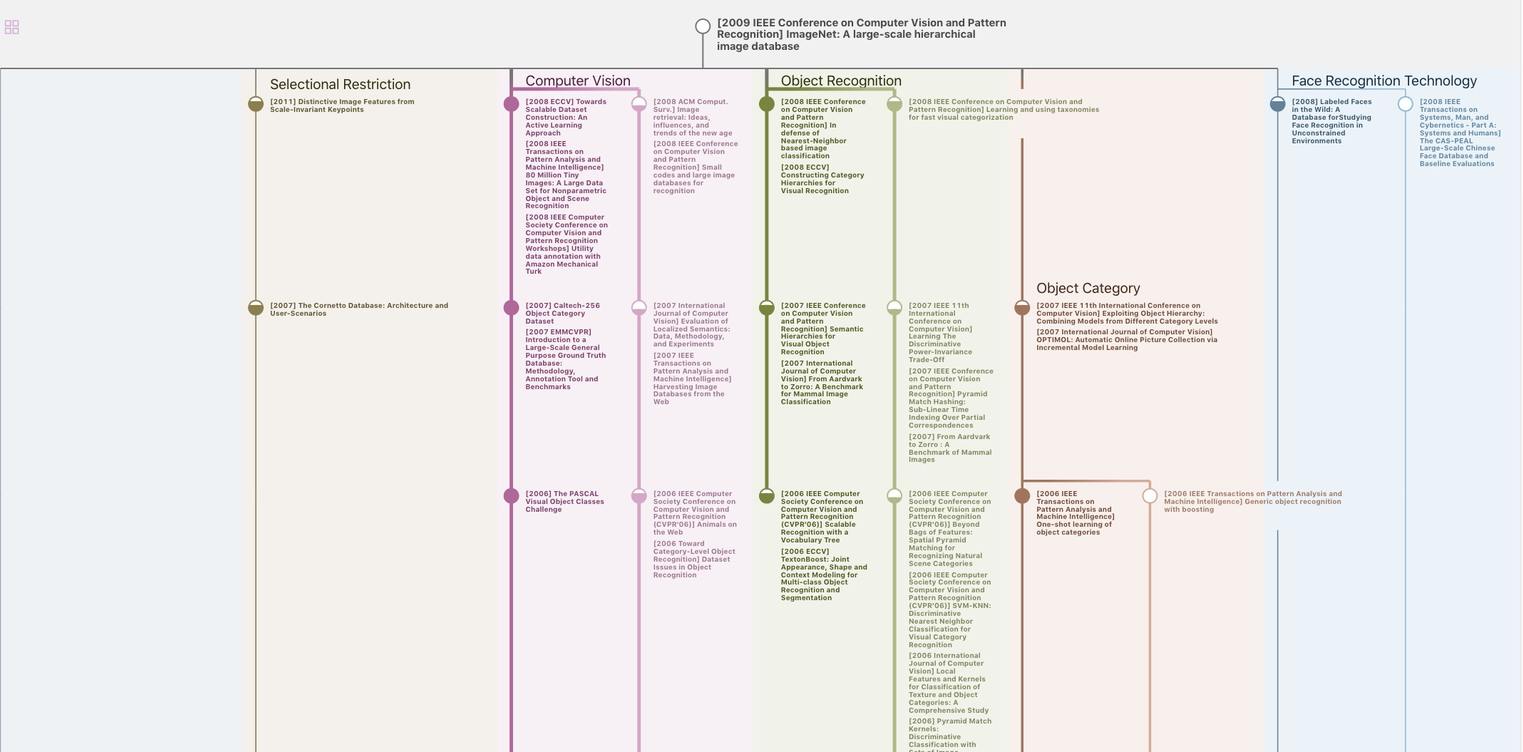
生成溯源树,研究论文发展脉络
Chat Paper
正在生成论文摘要