Multi-Scale Attention Generative Adversarial Network for Medical Image Enhancement
IEEE TRANSACTIONS ON EMERGING TOPICS IN COMPUTATIONAL INTELLIGENCE(2023)
摘要
High quality medical images are not only an important basis for doctors to carry out clinical diagnosis and treatment, but also conducive to downstream tasks such as image analysis. Although many medical image enhancement methods have achieved good results, some of them still have shortcomings in homogenizing illumination distribution and maintaining texture details, and even introduce boundary artifact noise. In order to deal with these problems, this paper proposes a multi-scale attention generative adversarial network (MAGAN) for medical image enhancement, which is suitable for unpaired images. Our MAGAN is trained in the confrontation between two generators and two discriminators. It tries to fuse multi-scale information in feature extraction by establishing feature pyramid, and filters irrelevant activation to highlight important regions based on attention distribution, which is positive for imaging. Moreover, MAGAN strengthens the constraints on the quality of enhanced image from the perspectives of illumination distribution, texture details, deep semantic features and smoothness, so as to improve the enhancement effect. Compared with six state-of-the-art methods, the experimental results show that MAGAN has the most significant image enhancement effect, and also performs best in the downstream task of image segmentation.
更多查看译文
关键词
Image enhancement,Decoding,Medical diagnostic imaging,Convolution,Feature extraction,Training,Semantics,Gan,medical image enhancement,attention,multi-scale,deep semantic feature
AI 理解论文
溯源树
样例
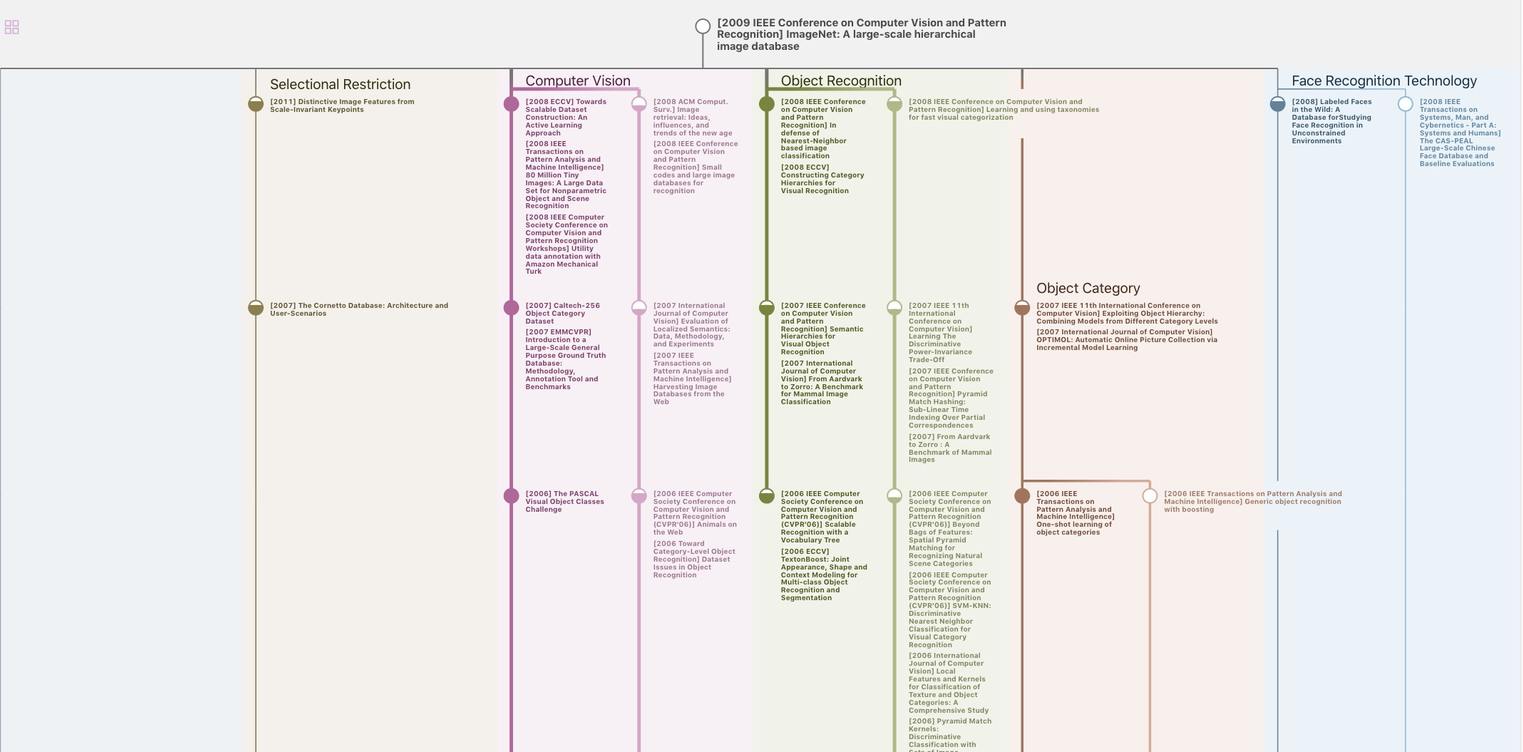
生成溯源树,研究论文发展脉络
Chat Paper
正在生成论文摘要