Advancing the marketing-operations interface in omnichannel retail
JOURNAL OF OPERATIONS MANAGEMENT(2023)
摘要
Omnichannel retail has experienced enormous growth in the last decade, becoming the new normal for many consumer products (McKinsey & Company, 2021). Many retailers have moved from being either pure bricks-and-mortar or online-only to serving customers across channels. For example, traditional retailers such as Walmart and Best Buy have opened e-commerce channels. Conversely, online-first retailers and vertically integrated brands like Amazon and Nike have added physical stores to their e-commerce channels (Avery et al., 2012). In contrast to multichannel retail where channels coexist without any coordination, omnichannel retail combines expansion into different channels with coordination and integration to facilitate seamless customer journeys (Akturk et al., 2018; Bijmolt et al., 2021; Hübner et al., 2016; Rooderkerk & Kök, 2019; Verhoef et al., 2015). To integrate their channels, retailers have adopted omnichannel fulfillment models such as “buy online pick up in-store” and “ship-from-store.” Channels have been (re)designed in terms of omnichannel customer journeys. To illustrate, digital vertical-native brands, such as Bonobos (men's wear) and Warby Parker (eyewear) employ “zero-inventory” showrooms where shoppers can inspect a product but not take it home. Such omnichannel fulfillment models combine the strengths of the different channels within a single customer journey: the store for physical inspection, and the online channel for convenient fulfillment. Omnichannel retail aims at facilitating customer switching between channels across and within customer journeys. Achieving this requires retailers to carefully consider the challenges that customers experience in combining channels within a given customer journey. This includes difficulty in accessing online information from within the store, or in obtaining reliable information as to whether a product found online is available for inspection in a given store (Rooderkerk & Kök, 2019). The friction created from traversing channels within a given customer-journey stage1 or between two consecutive stages is reduced through information technology and a variety of omnichannel fulfillment solutions (see, e.g., Akturk et al., 2018; Hübner et al., 2022). Balancing the advantages of seamless omnichannel integration with the cost of seamlessness represents a standard operations management (OM) trade-off exercise. Trading off effectiveness and efficiency must consider both the marketing and the operations perspective: Omnichannel fulfillment models that are effective with respect to measures like sales conversion and service quality may not appear to be efficient with respect to measures like fulfillment cost and lead time. The set of decisions related to the coordination of multiple channels within or across the same customer journey, aimed at carefully balancing superior customer journey experience and reliable and efficient product flow during the customer journey. Following Browning (2020) we observe that the marketing-operations interface in omnichannel retail is manifest in several areas, such as developing and managing new technologies to support omnichannel retail, new product development in omnichannel retail (Gallino & Rooderkerk, 2020), on-demand production in omnichannel settings (e.g., 3D printing), and leveraging omnichannel solutions for product support. Customer behavior and fulfillment emerge as key areas of focus in this special issue, building around key decisions that arise from the interaction between marketing and operations when implementing and managing omnichannel retail. Omnichannel consumer behavior and fulfillment models require retailers to involve both their operations and marketing functions in decision-making. Table 1 lists key decisions facing retailers concerning a specific type of omnichannel customer behavior and fulfillment, as well as the opportunities and threats that retailers should consider in making these decisions. Same as BOPIS, but in addition: For pure in-store customer journeys, all activities happen in the store: orientation, purchase, fulfillment, and—when applicable—return of goods. Here, buying the desired goods results in immediate fulfillment. Pure-online journeys aim to mimic the in-store experience while offering home delivery. Pickup points, whether attended or unattended, may be used to curb last-mile costs or offer the customer the convenience of not having to be at home for the delivery. Returns are done by mail, potentially involving prespecified drop-off points. The discussion on omnichannel customer behavior typically centers on the notion of research shopping (Verhoef et al., 2007), a term describing two different situations in which the orientation and purchase stages occur in different channels (in-store vs. online). (1a) Showrooming refers to in-store orientation followed by purchase from the online channel, while (1b) webrooming (or reverse showrooming) refers to in-store purchase preceded by online orientation. Both types and their key benefits, opportunities, threats, and decisions are highlighted in the first two rows of Table 1. Customers engage in research shopping to leverage the specific strengths of each channel (e.g., ease of use, technological support, quality of the information provision). For instance, online orientation provides the comfort of operating from home, while in-store orientation offers access to salesperson assistance and sensopathic (e.g., seeing, hearing, and touching) experiences. Purchasing in-store caters to instant gratification, while online purchases are associated with the convenience of home delivery. Depending on specific needs, the consumer composes her omnichannel customer journey by matching benefits sought in each decision-making stage to the best channel delivering those. Showrooming behavior provides retailers with the opportunity to operate zero-inventory showrooms, greatly reducing store-level inventory costs. However, competitive showrooming poses the threat that after seeing a product in a showroom, customers may end up buying it online from a competing retailer. Showrooming brings novel challenges. Optimizing store location is already complex for traditional brick-and-mortar-only retail, considering sociodemographics, the presence of rivals, and the availability and cost of real estate. Omnichannel retail increases the complexity of store location optimization. The opening of a site may cannibalize the online channel, or it may boost online purchases (e.g., Kumar et al., 2019) as well as raise the overall (store + online) demand by facilitating within-retailer showrooming behavior. When serving showroomers, retailers must decide what part of the assortment to show in-store versus what to keep online. Relatedly, retailers must choose what part of the online-only assortment to feature in-store — using, for example, virtual aisles — as they sense how customer substitution works across channels. Obvious reasons to showcase a certain product in the store include a high margin and low return rate of the item in question. However, Dzyabura et al. (2019) have shown that it also makes sense to showroom a product when the physical inspection is likely to alter original consumer preferences. Showrooming products with zero inventory in the store, on the other hand, may boost primary demand (category sales), but this could also increase online sales of long-tail items. The central inventorying of these products in an online warehouse then reduces retailers' inventory and fulfillment costs. In other situations where considerable webrooming behavior arises, the challenge is how to leverage clickstream data to extract early demand signals for stores. Acting on these insights, retailers can proactively position inventory toward certain stores in anticipation of the webrooming demand that materializes in-store. Table 1 further documents the seven most prominent fulfillment models applied in omnichannel retail, representing three specific groups. The first group of models involves the forward supply chain, including (2a) buy online, pick up in-store, (2b) reserve online, pick up in-store, and (2c) buy in-store, ship to home. These relate to online-to-offline channel migration, as well as buy-in-store and ship-to-home, which feature the opposite path in channel migration (offline-to-online). The second group focuses on the reverse supply chain. These include (3a) buy in-store, return online and (3b) buy online, return in-store. Finally, the third group, consisting of, (4a) ship-to-store and (4b) ship-from-store can be seen as fulfillment models that support other fulfillment models. Ship-to-store can be used to support buying online, pickup in-store, and ship-from-store to facilitate home delivery of online purchases. In the following, we will highlight the opportunities, threats, and key decisions for each of these models. Successful (2a) buying online and pickup in-store requires reliable store-inventory information that is accessible in real-time (Gallino & Moreno, 2014). The retailer must dynamically decide what part of the store inventory to reserve for “pure” store demand versus online pickup orders. With limited inventories, rules will have to be in place that balance expected in-store demand with the probability that the online consumer will settle for alternative home delivery. Some retailers also decide to accept online orders for store pickups with the option to cancel them later when store demand is observed, which imposes a trade-off between increasing online sales and customer irritation with canceled orders (Jia et al., 2022). Regarding store operations, retailers need to carefully consider how to integrate the picking and fulfillment of buy online, pickup in-store orders into their store labor flow (e.g., using dedicated resources to speed up the lead time). The optimal configuration is defined by factors like the (relative) volume of buy online, pickup in-store demand, and its correlation over time with in-store traffic and conversion. A slight variation of this model, (2b) reserve online, pickup in-store, imposes inventory-allocation decisions like when the retailer should cancel a reservation to make the product available again for in-store sales. The fulfillment model (2c) buy in-store, ship to home offers customer convenience when compared to a pure in-store journey. It also offers cross-selling and upselling opportunities for the retailer in comparison to an online purchase. This model results in an increase in picking costs relative to in-store pickup that is incurred in the store or DC. It also results in shipping costs from either the store or the DC to customers. When it comes to returning orders across channels with (3a) buy in-store, return online and (3b) buy online and return in-store, marketing, and operations objectives need to be aligned to minimize handling costs, address inventory imbalances, and enable the conversion of returns into a positive outcome for the retailer. Retailers can require that returns be made by postal mail when it is more cost-efficient to process the returns in a central returns center. At other times, retailers may want to encourage in-store returns when this facilitates direct replacements, the sale of substitutes, or cross-selling (e.g., selling a complimentary item that will increase the performance of the item intended for return). Inventory imbalance may lead sellers to encourage in-store returns: When a given store is low on inventory of an item that is about to be returned, the retailer can encourage the customer to return its online purchase thereby citing factors such as the ease of processing an immediate refund. Retailers can adopt (4a) ship-to-store to facilitate in-store pickups that boost store traffic, leverage more efficient picking systems in the DC, or offer long-tail products stocked at DCs. The extent to which retailers can offer this service cost-efficiently depends on their ability to consolidate the ship-to-store deliveries with regular supplies to the store such that they do not overly increase the lead time for consumers (Wu & Chen, 2022). (4b) Ship-from-store was often adopted early on in retailers' evolution toward omnichannel retail and became popular again during the pandemic (Sillitoe, 2022). It can be a very effective strategy for retailers with large store networks. For very dense store networks, an interesting optimization problem is deciding from which store to ship an item, factoring in the distance to the consumer, picking capacity at the store, and the level and age of inventory. Section 2 made clear that understanding and mitigating the potential information barriers between online and offline channels are key to success in the omnichannel context. Ren et al. (2023), the first paper in this special issue, study the effect of sharing brick-and-mortar store product availability in an omnichannel context with consumers subject to asymmetric assortments. Using data related to housewares, toys, and apparel of a North-American retailer, they show that online sharing of offline product availability data increased overall sales, particularly from customers within the trading area of the store. They show that sharing this information shifted sales from the physical to the online channel. Consumers were observed to switch to the online channel when they became aware that local stores might not have the product in stock, with this shift to online accompanied by a slight increase in the return rate. This study shows the importance of jointly considering marketing and operations aspects of omnichannel initiatives, unveiling interesting (unintended) side-effects: Cross-channel product assortment transparency in omnichannel retail not only turns out to boost overall sales, but it also increases return rates because of customers migrating to the online channel. To the extent that e-marketplaces such as Taobao and Amazon are part of omnichannel customer journeys, friction involved with the use of this channel type could result in substantial losses to retailers. However, the online-offline integration of platforms has received little attention so far. Addressing this gap, Fang et al. (2023) compare two fulfillment models that arise when, under competitive pressure, platforms decide to provide third-party sellers support for channel integration. In the first fulfillment model, the so-called (i) informational online-offline channel integration, the platform provides so-called “SAME” labels to signal that the identical product is also available at third-party sellers' sites. The second model, called (ii) transactional online-offline channel integration, constitutes the buy online, pickup in-store (at one of the third-party's physical sites) fulfillment. Using data from a Chinese B2C platform, Fang et al. (2023) show that offering (i) informational integration dampens a platform's sales growth while (ii) transactional integration has no significant effect on sales growth. They further reveal how these effects are moderated by the seller's inter-platform function usage. Platforms that feel competitive pressure to respond to third-party seller demands by developing cross-channel integration may reduce friction and channel cannibalization effectively by prioritizing a buy-online, pickup-in-store model. Current omnichannel literature has relied heavily on analytical modeling to portray the mechanisms in omnichannel retail (e.g., Gao & Su, 2017; Wu & Chen, 2022), or on quasi-experimental work that analyzes the effect of channel expansion-integration and the introduction of omnichannel fulfillment models (e.g., Akturk et al., 2018; Avery et al., 2012; Fang et al., 2023; Gallino & Moreno, 2014; Ren et al., 2023). This work has mainly been descriptive and sales-centric. Our special issue has sought to add empirical work to this important topic. The presentation of customer behavior and fulfillment models in Table 1 suggests that ample opportunities exist for both retailers and customers to gain an advantage from omnichannel fulfillment, and empirical research can lead to improved decision-making in this context. The growing availability of omnichannel systems and data encourages researchers to investigate the omnichannel marketing-operations interface. Key research areas include: Omnichannel retail can prove successful only with proper data management and data lakes in place that cut across channel siloes. Facilitating consumers in the multitude of customer journeys requires the use of advanced analytics (Rooderkerk et al., 2022). This calls for a better understanding of the IT systems and analytical capabilities necessary to optimize omnichannel interactions and fulfillment, as well as what these imply for IT functionality and interoperability requirements among network partners (Wollenburg et al., 2018). More empirical research is needed to investigate which analytical capabilities will become decisive and which tools and digital services will efficiently support the customer journey. Further insights are also needed into how to integrate omnichannel order and return management with inventory planning and how and when to make specific information (e.g., on product availability) available along the customer journey. For online customers, it is possible to link customer data to behavior; for store traffic, this is impossible without identifying customers. However, mitigation of privacy infringement is pivotal to consumer trust and patronage favor (Cheah et al., 2020). Many retailers thus prefer the “opt-in” route, for example setting up loyalty-card programs where customers consent to the use of their personal data. Research can help identify the best way to target omnichannel customers and their fulfillment preferences. In general, an important question that requires attention is how retailers can develop omnichannel analytics capabilities that start from the premise of respecting privacy rather than seeing that as an afterthought. Customer preferences for fulfillment models need to be combined with the different economics of these fulfillment models to actively steer customers to the most efficient options. Enhanced inventory, capacity, and workforce management combined with marketing activities (e.g., nudging certain fulfillment models or channels) result in a better balance of the workload, shorten the lead time, and increase product availability. Exemplary research questions are the following. How can omnichannel fulfillment operations be organized and planned to account for the varying delivery requirements of consumers in each channel? How can marketing and operations activities be used to smooth demand across channels? How can revenue management techniques contribute to optimal cross-channel coordination of assortment and inventory? Consideration for the social and environmental impacts of different omnichannel fulfillment models remains rather limited but will become more front and center as demanded by vocal customers and novel legislation.2 Research in this domain has launched with, for example, the work of Agatz et al. (2021) on the impact of green labels on time-slot choice in webshops. Further empirical research is needed to understand the trade-off between lead time (e.g., fast delivery) and sustainability (e.g., a longer lead time when bundling orders) of different fulfillment options. Furthermore, the impact of technological innovations in last-mile delivery such as robot delivery needs to be analyzed in terms of their potential for sustainable omnichannel fulfillment. Related, it is important to consider how and if omnichannel fulfillment can reduce product returns and their CO2 footprint. Other domains at the marketing-operations interface in omnichannel retail such as new product development and product support certainly deserve more attention. Interesting questions for future research to answer include how we can better design products when keeping the specific characteristics of information, transaction, and fulfillment channels into account (Gallino & Rooderkerk, 2020), and how the different own channels (e.g., web, store, and call center) but also third-party e-marketplaces can be used to provide product support. The authors would like to thank Nicole DeHoratius for joining as guest editor of the special issue. In addition, the authors would like to thank the associate editors and reviewers for their great service. Finally, the authors would like to thank the Co-Editors-in-Chief, Suzanne de Treville and Tyson Browning, for their support.
更多查看译文
关键词
omnichannel retail,marketing‐operations,interface
AI 理解论文
溯源树
样例
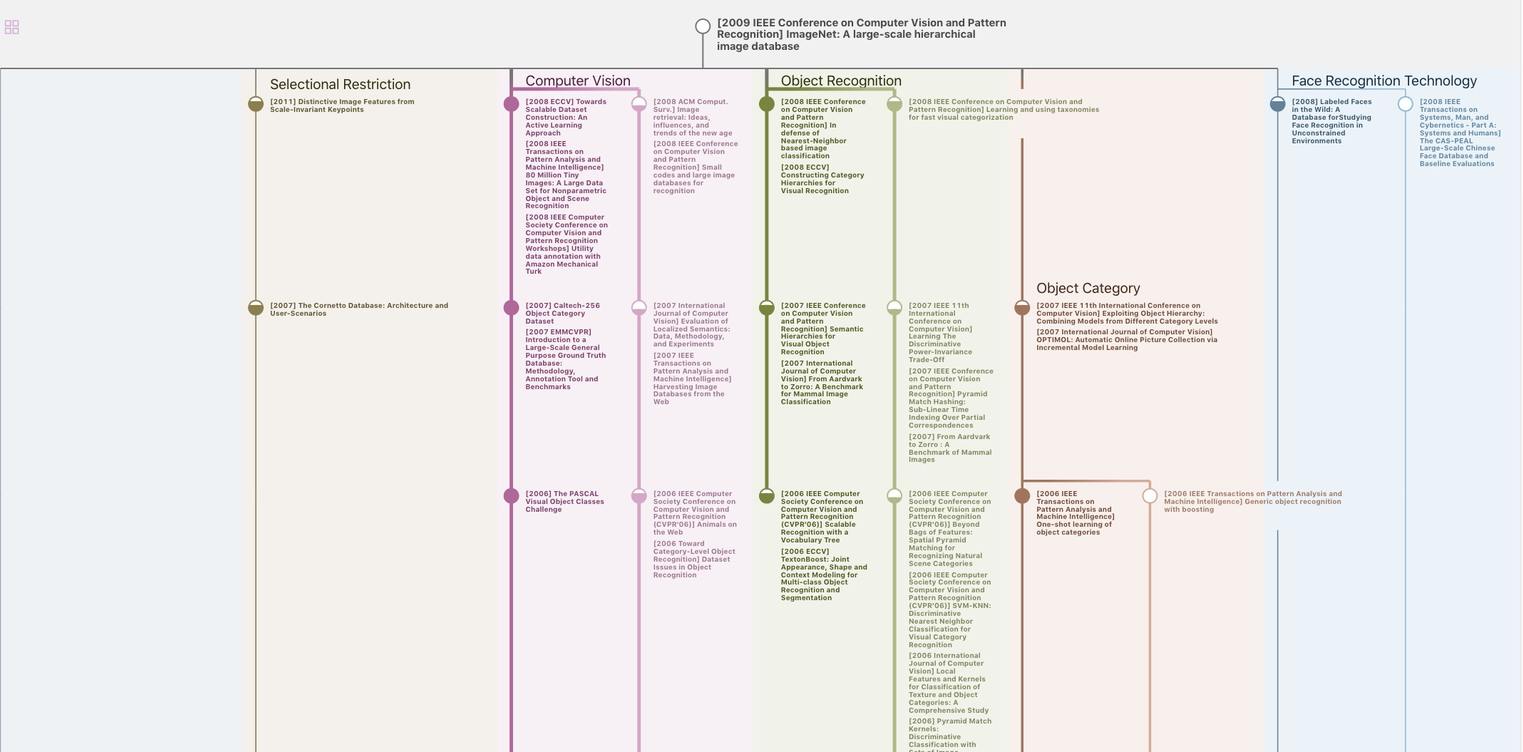
生成溯源树,研究论文发展脉络
Chat Paper
正在生成论文摘要