A hybrid model for spatial–temporal prediction of PM 2.5 based on a time division method
INTERNATIONAL JOURNAL OF ENVIRONMENTAL SCIENCE AND TECHNOLOGY(2023)
摘要
In order to address the problem of air pollution along with the fast development of the economy and an increase of urban population, this paper aims to capture the spatial–temporal correlation in air quality data and improve the prediction accuracy of PM 2.5 concentration. Existing models usually neglect the correlation in temporal and spatial dimensions, and the prediction results do not correspond to those in practical applications. We propose a multi-period spatial–temporal graph convolutional network (MST-GCN), in which the time division module fully explores the temporal correlation within the data and builds separate models for different time periods; the spatial–temporal convolution module captures the characteristics of historical data in the time dimension and spatial dimension, in which GCN is used to learn spatial dependencies between stations, and CNN is applied to learn the temporal dependencies of adjacent time points; meanwhile, a spatial–temporal attention mechanism module is introduced to enhance the key information in different dimensions, thereby capturing the spatial–temporal dynamic correlation. Finally, the experiments with real air quality data from Beijing monitoring stations show that the time division method is effective to extract the features in time dimensions; therefore, the model is able to capture the characteristics and dynamic correlation of air quality data in spatial–temporal dimensions. The superiority of the proposed method has been validated over state-of-the-art methods, and the average mean absolute error and root-mean-square error of MST-GCN are 34.37 and 35.55, respectively, which achieves the best performance in the experiments.
更多查看译文
关键词
Air quality,Graph convolutional networks,PM2.5,Spatial–temporal correlation,Time division
AI 理解论文
溯源树
样例
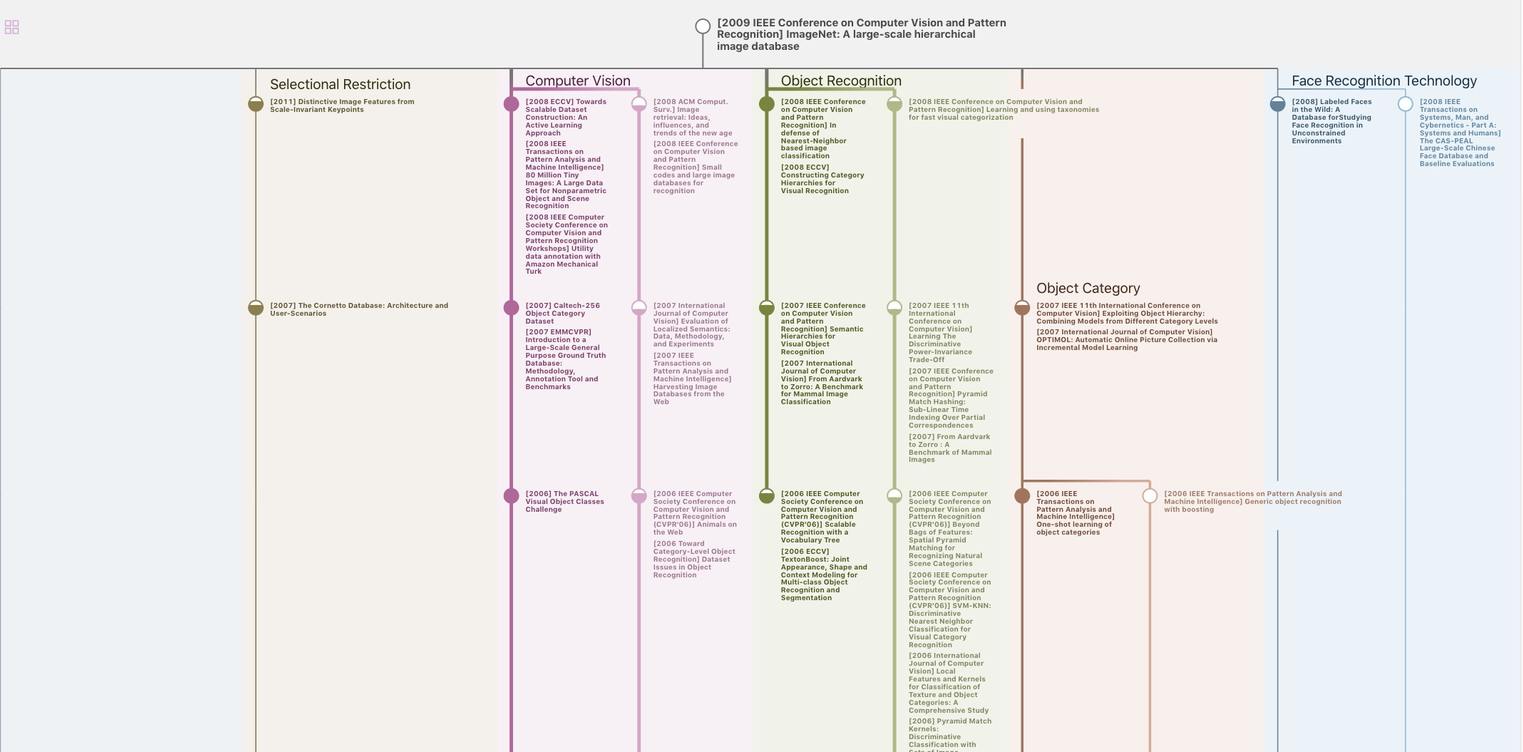
生成溯源树,研究论文发展脉络
Chat Paper
正在生成论文摘要