Online quantitative monitoring of milling cutter health condition based on deep convolutional autoencoder
INTERNATIONAL JOURNAL OF ADVANCED MANUFACTURING TECHNOLOGY(2023)
摘要
The health condition of milling cutters (HCOMC) could heavily affect workpiece quality. However, it is extremely difficult to be quantified online. To solve this problem, an online quantitative monitoring method (OQM) is proposed based on a deep convolutional autoencoder (CAE). In this method, a health indicator (HI) is constructed for fast HCOMC monitoring. The OQM is composed of two parts, offline training and online monitoring. In the offline stage, the multi-sensor monitoring data that record in the cutter normal wear stage (named normal wear data, NWD) are selected from a subsampled life testing dataset to train a deep CAE. In the online stage, each monitoring data segment (MDS) is directly input into the trained CAE to obtain deep representations. Then, the HI is constructed by the mean square error (MSE) between the MDS and the deep representations to monitor the HCOMC. It is called convolutional-autoencoder-reconstruction-error-based health indicator (CARE-HI). In addition to the above-mentioned method, a new metric named isometric fusion metric (IFM) is also designed to assess HI. IFM is able to address the uneven problem of property contribution when using some widely used HI metrics. In the experiment, 28 milling cutters were subjected to cutting experiments under different working conditions. The experimental result demonstrates that the proposed OQM can efficiently improve feature quality and precisely monitor HCOMC. It also illustrates that the CARE-HI outperformed some existing ones in five metric dimensions. Therefore, the proposed CARE-HI can provide more accurate guidance for tool changing in machining.
更多查看译文
关键词
Online quantitative monitoring,Health indicator,Convolutional autoencoder,Fusion metric,Milling cutters
AI 理解论文
溯源树
样例
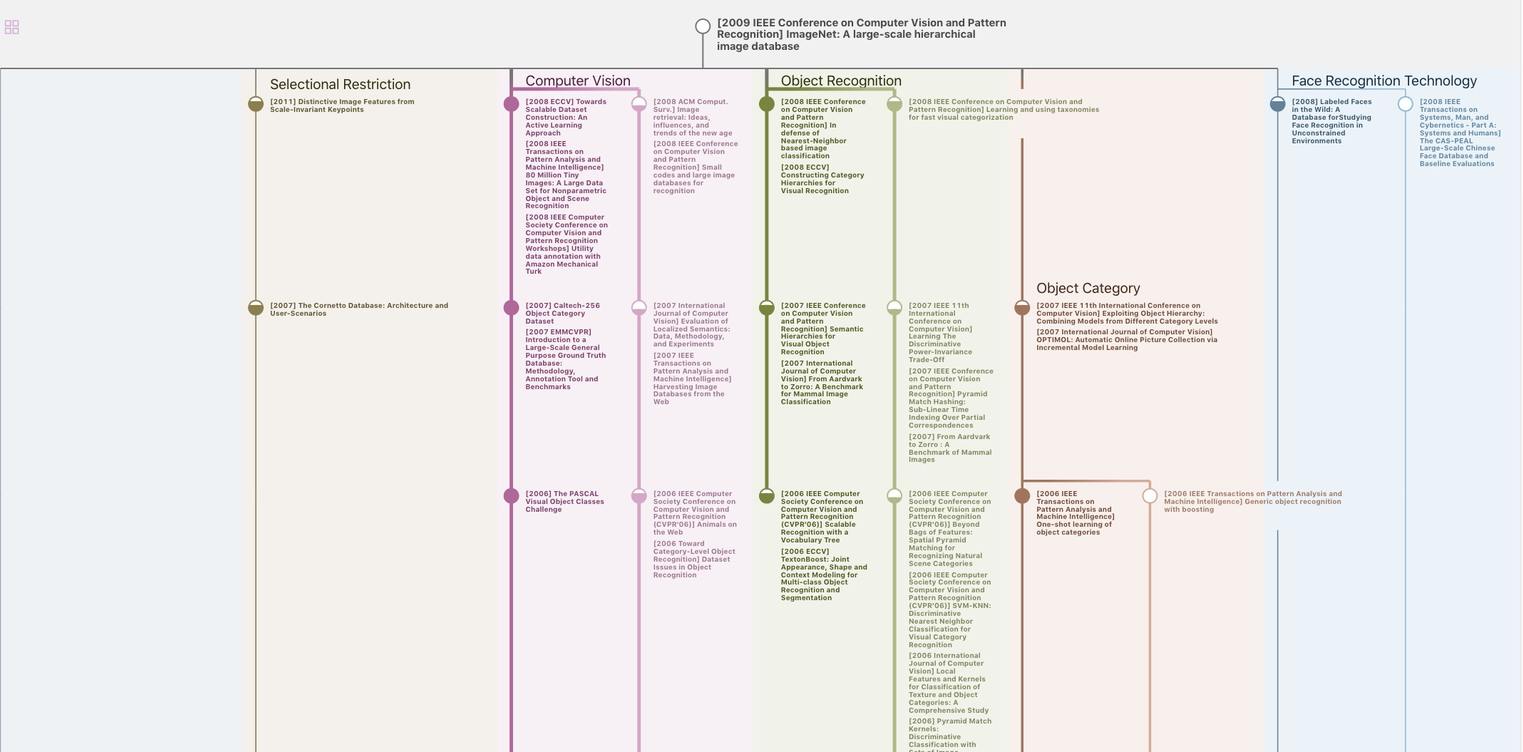
生成溯源树,研究论文发展脉络
Chat Paper
正在生成论文摘要